Automatic coarse-to-refinement-based ultrasound prostate segmentation using optimal polyline segment tracking method and deep learning
Appl. Intell.(2023)
摘要
Automatic segmentation of the prostate in transrectal ultrasound (TRUS) images provides useful information for prostate cancer diagnosis and treatment. However, boundaries between the prostate and other tissues are often absent or ill defined in TRUS images, which means that automatic segmentation of the prostate in TRUS images is highly challenging. In this study, we attempted to overcome these challenges by developing a novel method we termed “automatic prostate segmentation” (Auto-ProSeg) that is capable of effectively segmenting the prostate in TRUS images. Auto-ProSeg comprises two steps: the first step is a preprocessing step that uses attention U-Net to extract approximate prostate contours automatically; then, in the second step, the approximate prostate contours are optimized via a modified principal curve-based method linked to an evolutionary neural network, whereby a mathematical mapping formula based on the parameters of an enhanced evolutionary neural network is used to generate smooth prostate contours. Our results illustrate that Auto-ProSeg exhibits better prostate segmentation performance than other recently developed methods: the average Dice similarity coefficient and Jaccard similarity coefficient (Ω) of Auto-ProSeg-generated prostate contours against ground truths were 94.2% ± 3.2% and 93% ± 3.7%, whereas those for other state-of-the-art fully automatic segmentation methods were approximately 90% ± 5% and 89% ± 6%, respectively.
更多查看译文
关键词
Ultrasound prostate segmentation,Attention gate Unet,Optimal polyline segment tracking method,Evolution neural network,Mathematical mapping formula
AI 理解论文
溯源树
样例
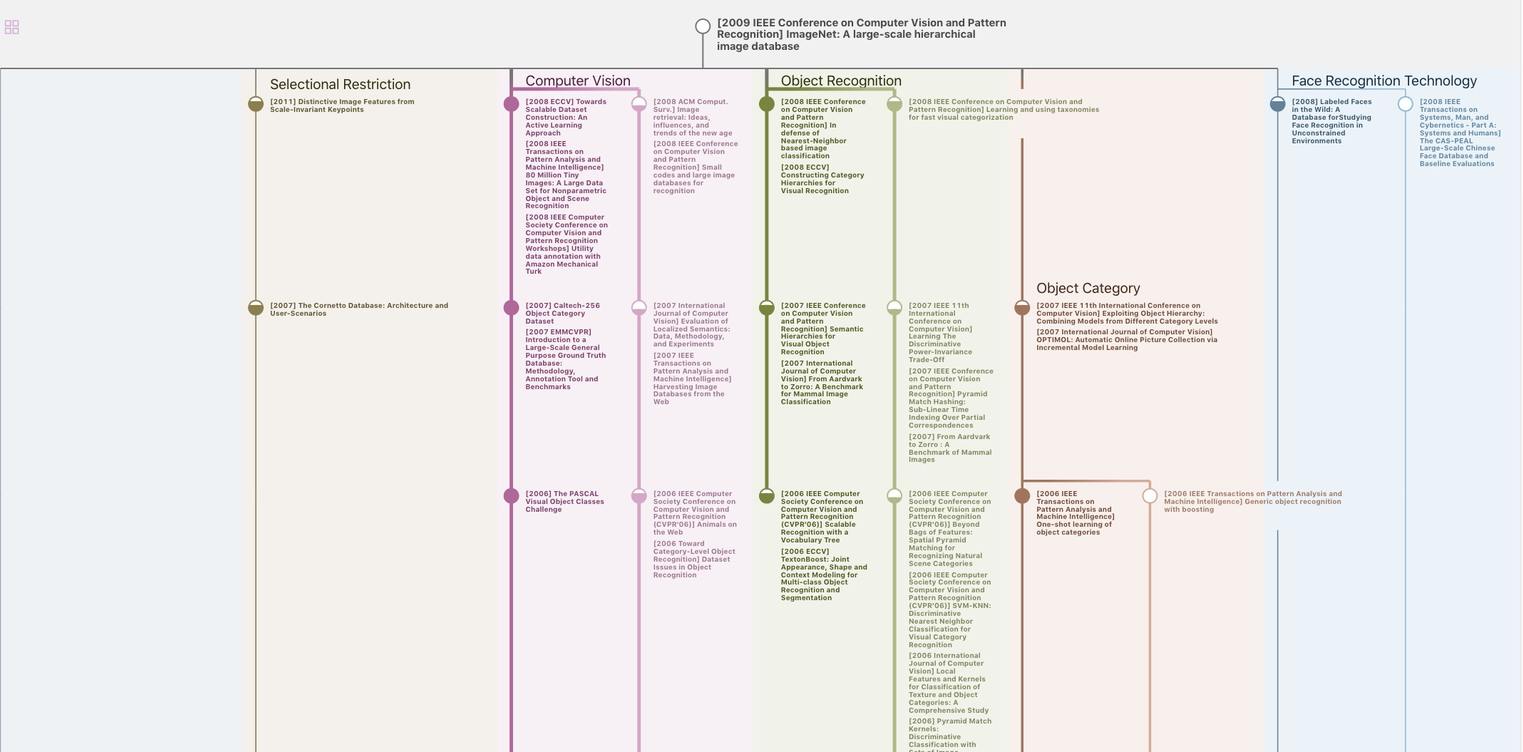
生成溯源树,研究论文发展脉络
Chat Paper
正在生成论文摘要