Use of remote sensing data to predict soil organic carbon in some agricultural soils of Iran
REMOTE SENSING APPLICATIONS-SOCIETY AND ENVIRONMENT(2023)
摘要
Quantifying and monitoring of SOC (soil organic carbon) content in an agriculture soil is essential for understanding the ecological environment, influencing the climate change and implementing the best agriculture management. Present study tried to derive some machine learning-based models for predicting SOC content by employing remote sensing data in some agriculture soils enriched by calcareous materials, in central part of Iran. Soil samples were taken from 336 points at arable and permanent agriculture soils. Soil features such as SOC, sand, silt, clay, bulk density and calcium carbonate equivalent contents were measured at each point. After that, machine learning models including artificial neural networks (ANN), support vector regression (SVR) and genetic expression programming (GEP) were employed to estimate SOC content. The input variables were spectral indices extracted from Landsat-8, Sentinel-2, Sentinel-3 and MODIS images (Scenario I), integrating of the spectral indices extracted from Landsat-8—MODIS and Sentinel-2—Sentinel-3 images (Scenario II) and the spectral indices extracted from the fusing Sentinel-2 and Sentinel-3 images (Scenario IIII). The results of present study showed that the SVR model was the best performance (MAE = 0.0056%, RMSE = 0.620% and R2 = 0.627 at test data sets) for all sampled data by using the extracted remote sensing information at scenario II. Moreover, SVR model yielded the better result in the arable and permanent agriculture soils by using the extracted remote sensing information at scenario II (MAE = 0.0042%, RMSE = 0.672% and R2 = 0.574) and scenario III (MAE = 0.0032%, RMSE = 0.342% and R2 = 0.381), respectively. It was concluded that the combining of remote sensing data extracted from different types of satellites in a various method could improve the accuracy of SOC prediction.
更多查看译文
关键词
Agriculture soils,Environmental data,Machine learning models,Spectral indices
AI 理解论文
溯源树
样例
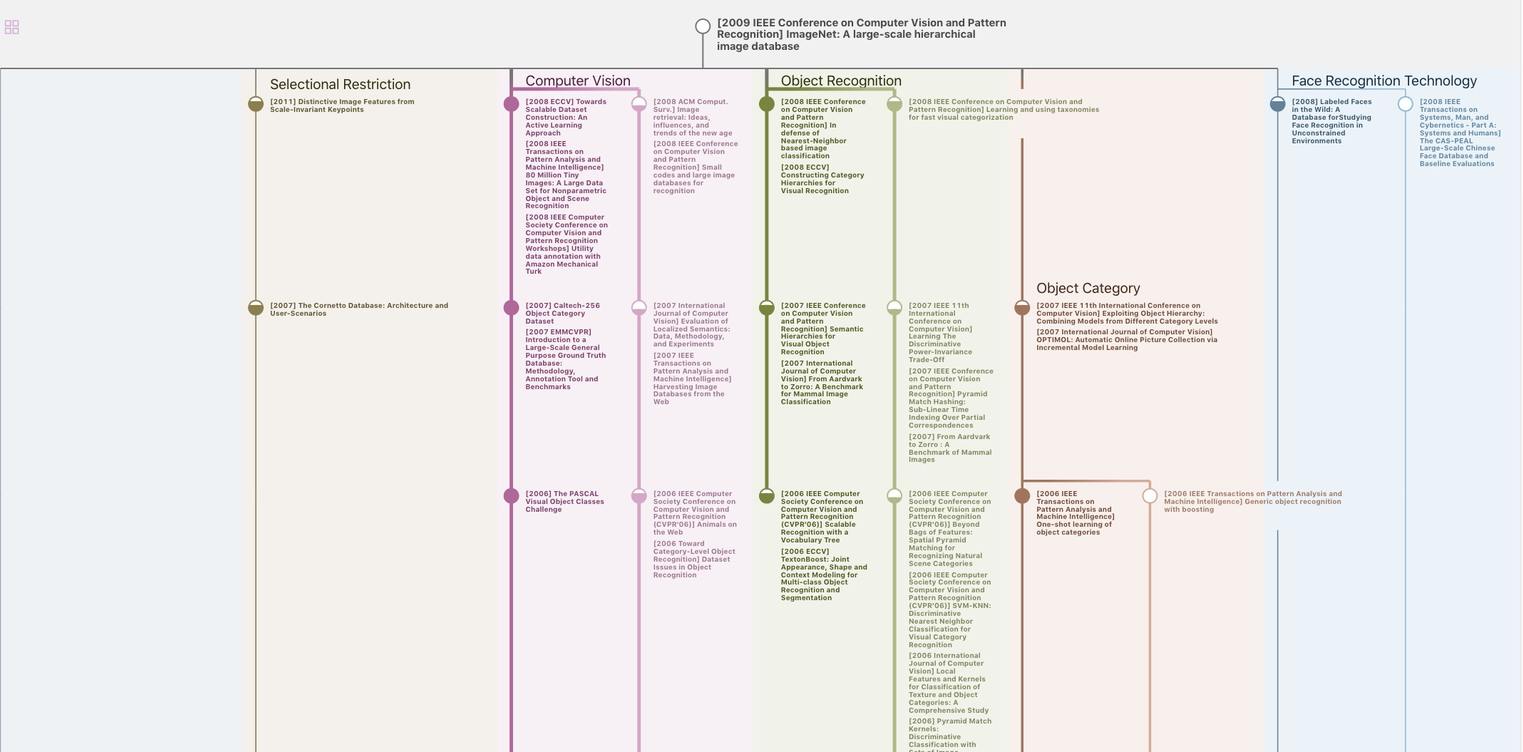
生成溯源树,研究论文发展脉络
Chat Paper
正在生成论文摘要