Device Quantization Policy and Power-Performance- Area Co-Optimization Strategy in Variation-Aware In-memory Computing Design
Research Square (Research Square)(2021)
摘要
Abstract Device quantization of in-memory computing (IMC) that considers the non-negligible variation and finite dynamic range of practical memory technology is investigated, aiming for quantitatively co-optimizing system performance on accuracy, power, and area. Architecture- and algorithm-level solutions are taken into consideration. Weight-separate mapping, VGG-like algorithm, multiple cells per weight, and fine-tuning of the classifier layer are effective for suppressing inference accuracy loss due to variation and allow for the lowest possible weight precision to improve area and energy efficiency. Higher priority should be given to developing low-conductance and low-variability memory devices that are essential for energy and area-efficiency IMC whereas low bit precision (< 3b) and memory window (<10) are less concerned.
更多查看译文
关键词
computing,power-performance,co-optimization,variation-aware,in-memory
AI 理解论文
溯源树
样例
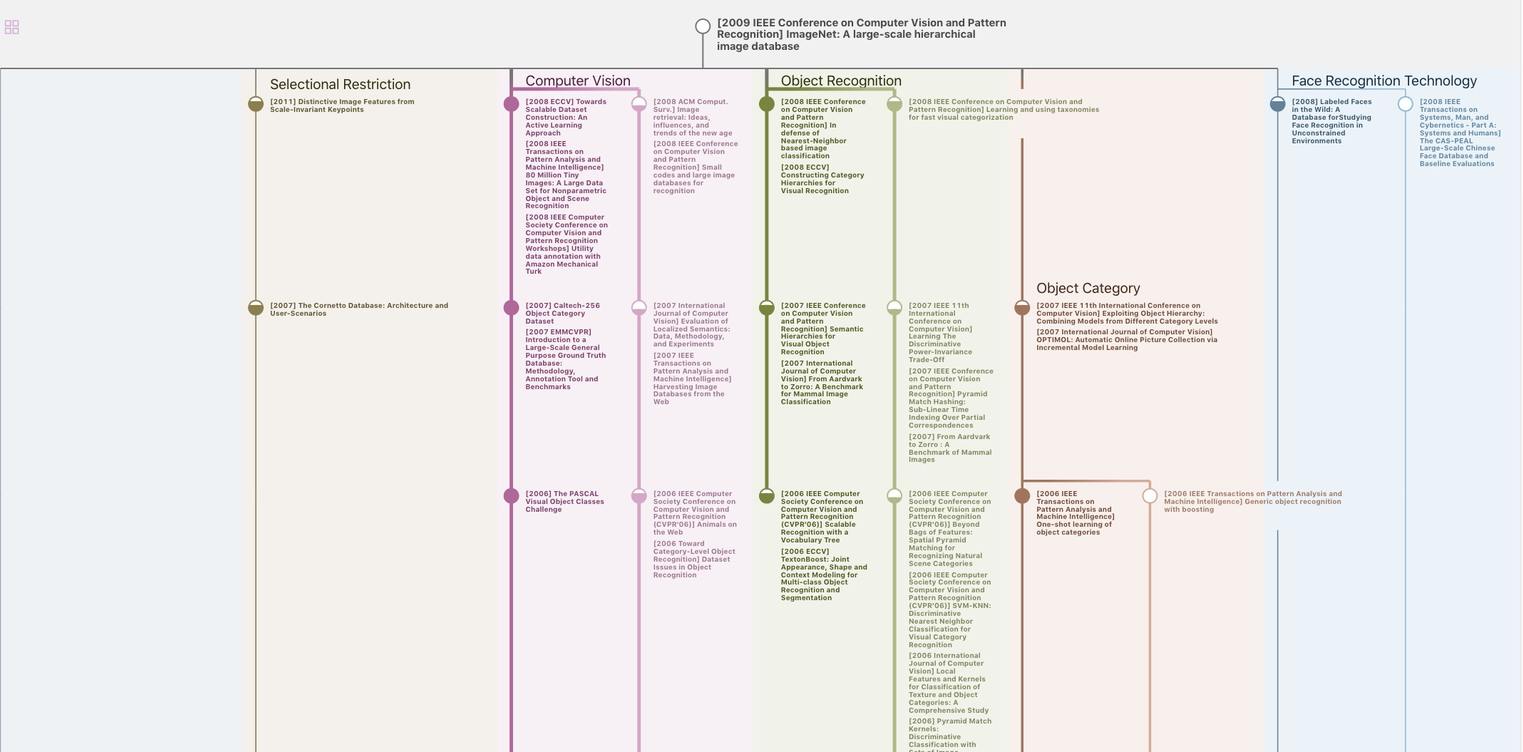
生成溯源树,研究论文发展脉络
Chat Paper
正在生成论文摘要