Learning discriminative visual semantic embedding for zero-shot recognition.
Signal Process. Image Commun.(2023)
摘要
We present a novel zero-shot learning (ZSL) method that concentrates on strengthening the discriminative visual information of the semantic embedding space for recognizing object classes. To address the ZSL problem, many previous works strive to learn a transformation to bridge the visual features and semantic representations, while ignoring that the discriminative property of the semantic embedding space can benefit zero-shot prediction tasks. Among these existing approaches, human-defined attributes are typically employed to build up the mid-level semantics. However, the discriminative capability and completeness of manually defined attributes are hard to guarantee, which may easily cause semantic ambiguity. To alleviate this issue, we propose a discriminative visual semantic embedding (DVSE) model that formulates the ZSL problem as a supervised dictionary learning framework. The proposed method is capable of exploring a set of discriminative visual attributes and ensures knowledge transfer across categories. Moreover, a unified objective is introduced to generate an augmented semantic embedding space where these learned visual attributes and human-defined attributes are incorporated jointly for consolidating the visual cues of feature representations. Finally, we treat the DVSE model as an optimization problem and further propose an iterative solver. Extensive experiments on several challenging benchmark datasets demonstrate that the proposed method achieves favorable performances compared with state-of-the-art ZSL approaches.
更多查看译文
关键词
discriminative visual semantic,recognition,learning,zero-shot
AI 理解论文
溯源树
样例
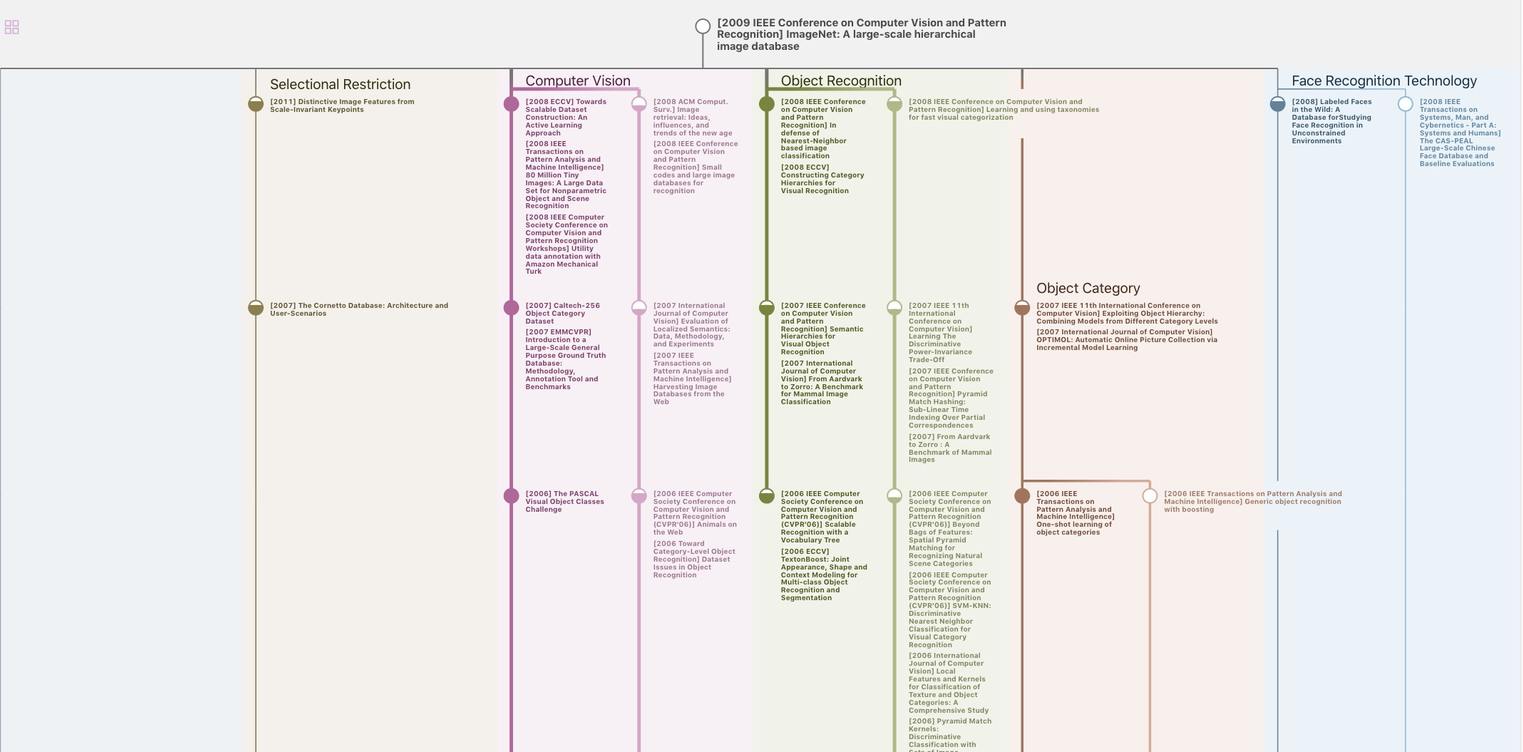
生成溯源树,研究论文发展脉络
Chat Paper
正在生成论文摘要