A Novel Combined DenseNet and Gated Recurrent Unit Approach to Detect Energy Thefts in Smart Grids.
IEEE Access(2023)
摘要
Due to the illegal use of electricity, non-technical losses are exponentially increasing in electricity distribution systems day by day. With the debut of smart meters in the smart grid, new electricity theft attacks are welcomed. The investigation of abnormal electricity consumption patterns helps in detecting electricity thieves. Moreover, existing methods have poor electricity theft detection (ETD) accuracy due to imbalanced datasets provided by the utilities. They have also failed to capture both periodicity and non-periodicity of 1-D daily electricity usage data. We primarily propose a novel sampling technique to balance the dataset, named as random oversampling using both classes (ROBC). This technique performs oversampling using both the theft and normal classes. With this technique, the problem of low accuracy has been resolved. We also propose a unique ETD model using densenet-fully convolutional network (DenseNet-FCN) and gated recurrent unit (GRU) with a light gradient boosting machine (LightGBM), known as DenseNet-GRU-LightGBM, to address the above mentioned concerns. DenseNet-FCN module extracts periodic and non-periodic patterns from 2-D electricity consumption data in a precise way. Whereas, GRU module captures as well as memorizes features from 1-D consumption data. Afterwards, LightGBM module is used as an ensemble classifier to give final ETD results. As a result, the proposed model has excellent ETD results. Comprehensive simulations indicate that the proposed scheme outperforms other existing methods regarding ETD.
更多查看译文
关键词
energy thefts,gated recurrent unit approach,novel combined densenet
AI 理解论文
溯源树
样例
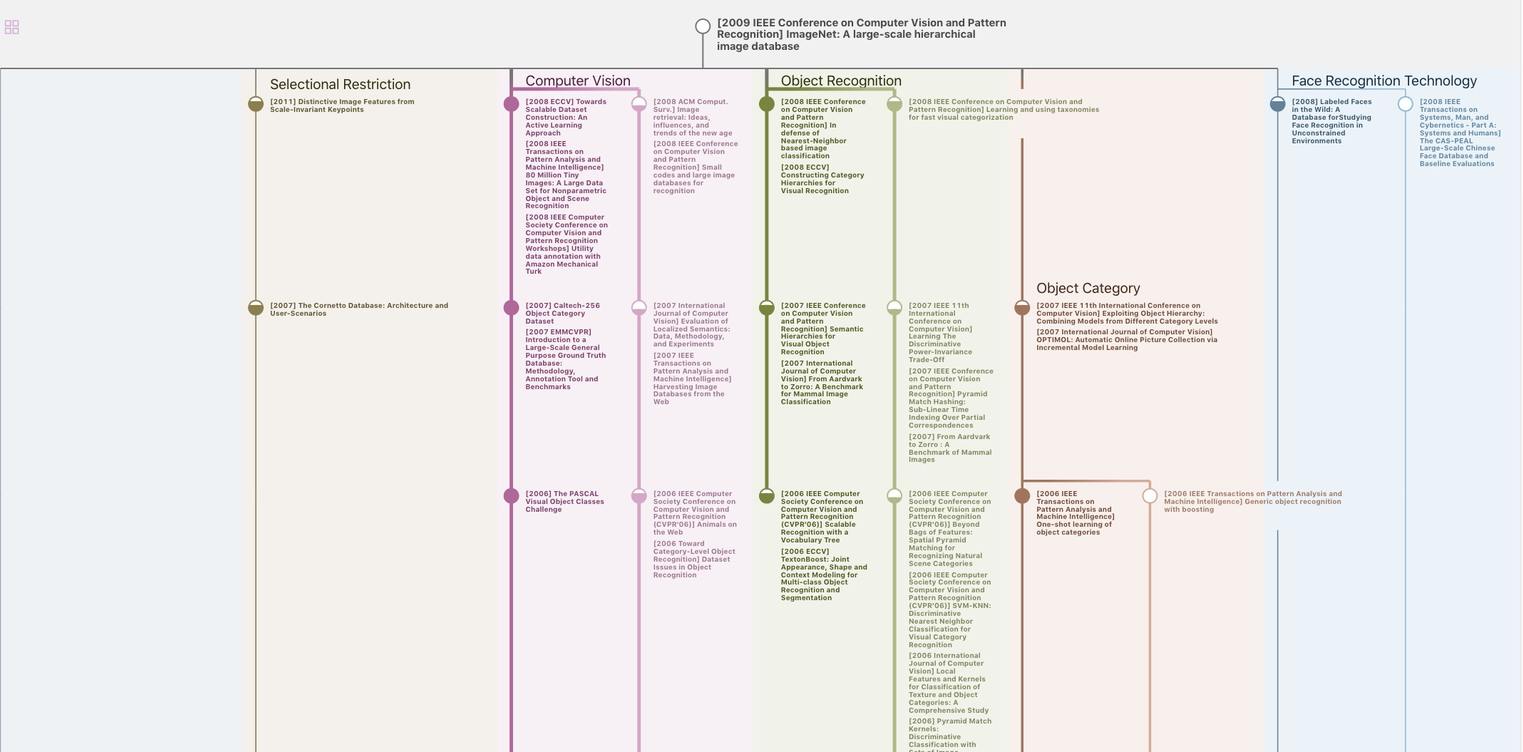
生成溯源树,研究论文发展脉络
Chat Paper
正在生成论文摘要