Improving the Results in Credit Scoring by Increasing Diversity in Ensembles of Classifiers.
IEEE Access(2023)
摘要
The ensembles of classifiers are techniques that have obtained excellent results in the credit scoring domain. It is known that Decision Trees (DTs) are suitable for ensembles because they encourage diversity, the key point for the success of an ensemble scheme. Ensembles of DTs have obtained good performance in a wide range of areas, including credit scoring. Some works have highlighted that DTs that employ imprecise probability models, called Credal Decision Trees (CDTs), improve the results of ensembles in credit scoring. The performance of CDT is strongly influenced by a hyperparameter. In fact, it was shown that different values of the hyperparameter might yield different models. Hence, the diversity in ensemble schemes can be increased by randomly selecting the value of the hyperparameter in each CDT, instead of fixing one. In this work, it is shown that increasing the diversity of the ensembles that use CDT by varying the value of the hyperparameter in each base classifier improves the results in credit scoring. Thereby, the use of CDT randomly selecting the value of the hyperparameter would suppose notable economic benefits for banks and financial institutions. Few gains in accuracy might imply huge gains in economic benefits.
更多查看译文
关键词
credit scoring,classifiers,ensembles,diversity
AI 理解论文
溯源树
样例
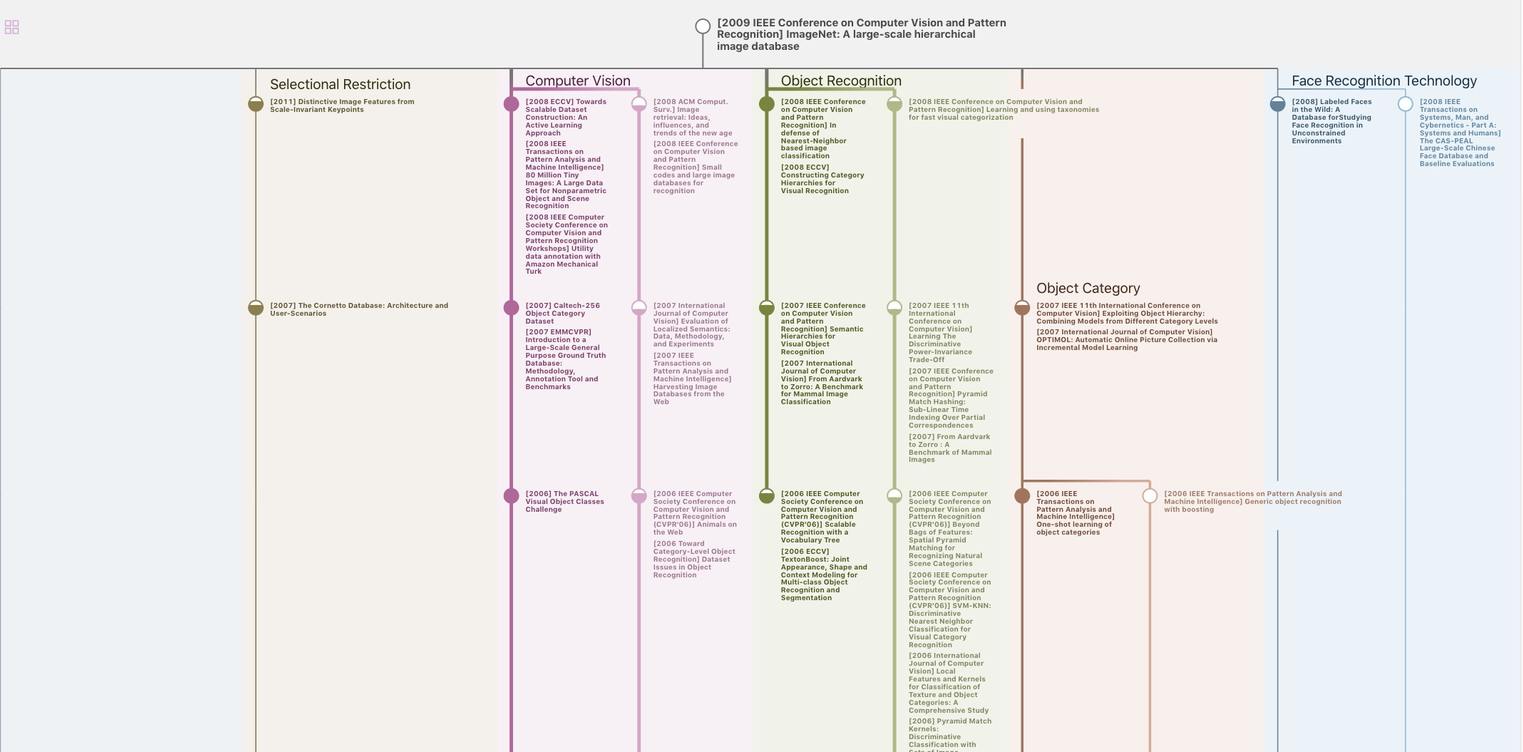
生成溯源树,研究论文发展脉络
Chat Paper
正在生成论文摘要