A Novel Approach for Disaster Victim Detection Under Debris Environments Using Decision Tree Algorithms With Deep Learning Features.
IEEE Access(2023)
摘要
Search and Rescue operations for victim identification in an unstructured collapsed building are high-risk and time-consuming. The possibility of saving a victim is high only during the first 48 hours, and then the prospect tends to zero. The faster the response and identification, the sooner the victim can be taken to medical assistance. Combining mobile robots with practical Artificial Intelligence (AI) driven Human Victim Detection (HVD) systems managed by professional teams can considerably reduce this problem. In this paper, we have developed a Transfer Learning-based Deep Learning approach to identify human victims under collapsed building environments by integrating machine learning classification algorithms. A custom-made human victim dataset was created with five class labels: head, hand, leg, upper body, and without the body. First, we extracted the class-wise features of the dataset using fine-tuning-based transfer learning on ResNet-50 deep learning model. The learned features of the model were then extracted, and then a feature selection was performed using J48 to study the impact of feature reduction in classification. Several decision tree algorithms, including decision stump, hoeffiding tree, J48, Linear Model Tree (LMT), Random Forest, Random Tree, Representative (REP) Tree, J48 graft, and other famous algorithms like LibSVM, Logistic regression, Multilayer perceptron, BayesNet, Naive Bayes are then used to perform the classification. The classification accuracy of the abovementioned algorithms is compared to recommend the optimal approach for real-time use. The random tree approach outperformed all other tree-based algorithms with a maximum classification accuracy of 99.53% and a computation time of 0.02 seconds.
更多查看译文
关键词
disaster victim detection,debris environments,deep learning features,deep learning
AI 理解论文
溯源树
样例
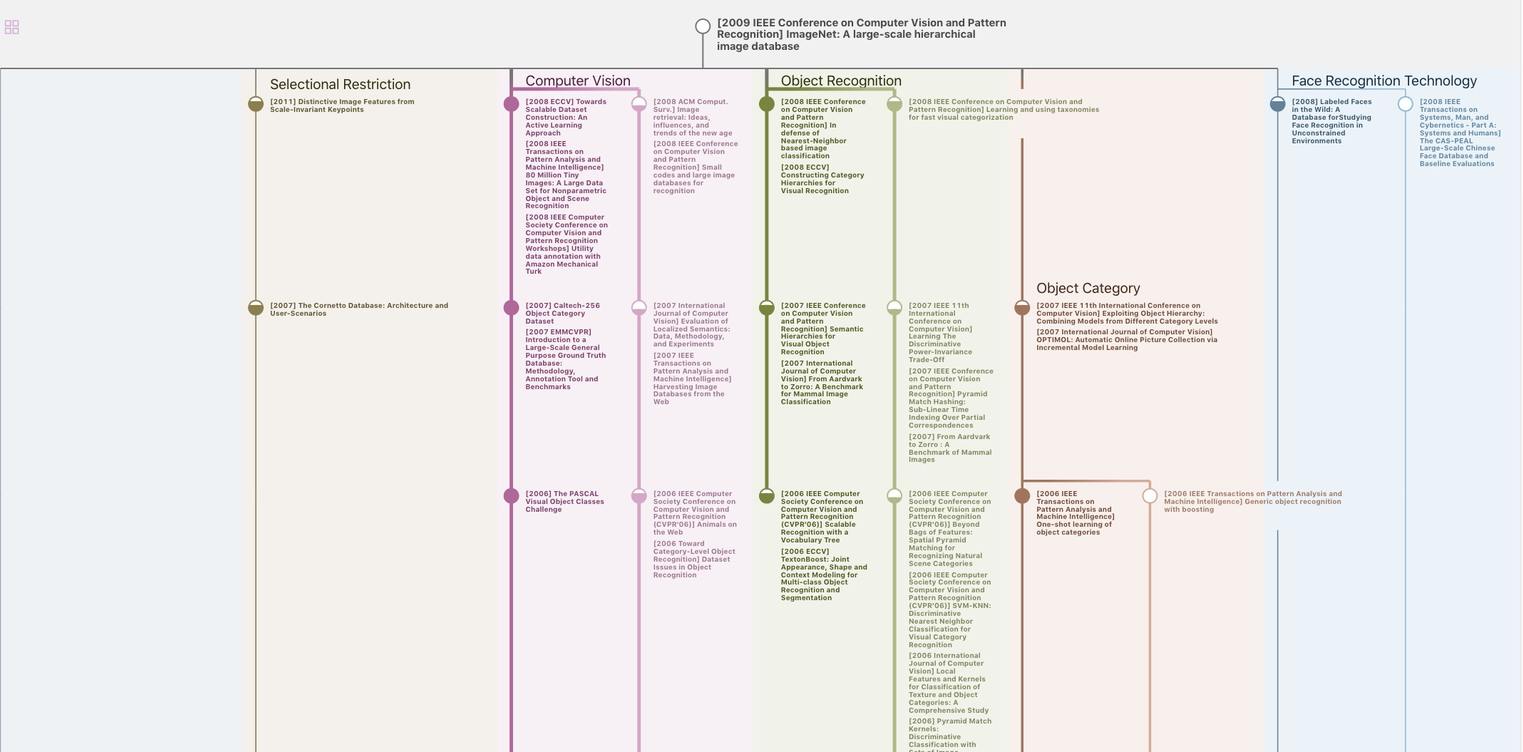
生成溯源树,研究论文发展脉络
Chat Paper
正在生成论文摘要