NE-GConv: A lightweight node edge graph convolutional network for intrusion detection.
Comput. Secur.(2023)
摘要
Resource constraint devices are now the first choice of cyber criminals for launching cyberattacks. Network Intrusion Detection Systems (NIDS) play a critical role in the detection of cyberattacks. The latest Graph Neural Network (GNN) technology, which learns over graph-structured data and thus can capture the impact of the network, has shown profuse results in network attack detection. However, most GNN approaches are limited to considering either node features or edge features. Our proposed approach overcomes this limitation by presenting a Node Edge-Graph Convolutional network (NE-GConv) framework which is equipped with both node and edge features. In particular, the network graph is formed by combining IP addresses and port numbers, and node and edge features are defined from packet contents and network flow data, respectively. Then, a two-layer model is designed, which implicitly performs edge classification by explicitly using node and edge features. Hence, the model is sensitive to intrusions in both packet contents and network flow. Furthermore, our framework addresses the constraints of lightweight devices by employing a feature selection unit before the NE-GConv and minimizing the number of hidden layers in the NE-GConv. The experimental results demonstrate our model outperforms other GNN models in terms of accuracy and false-positive rate and is computationally efficient.
更多查看译文
关键词
GNN,NIDS,Lightweight,IoT networks,Computational complexity
AI 理解论文
溯源树
样例
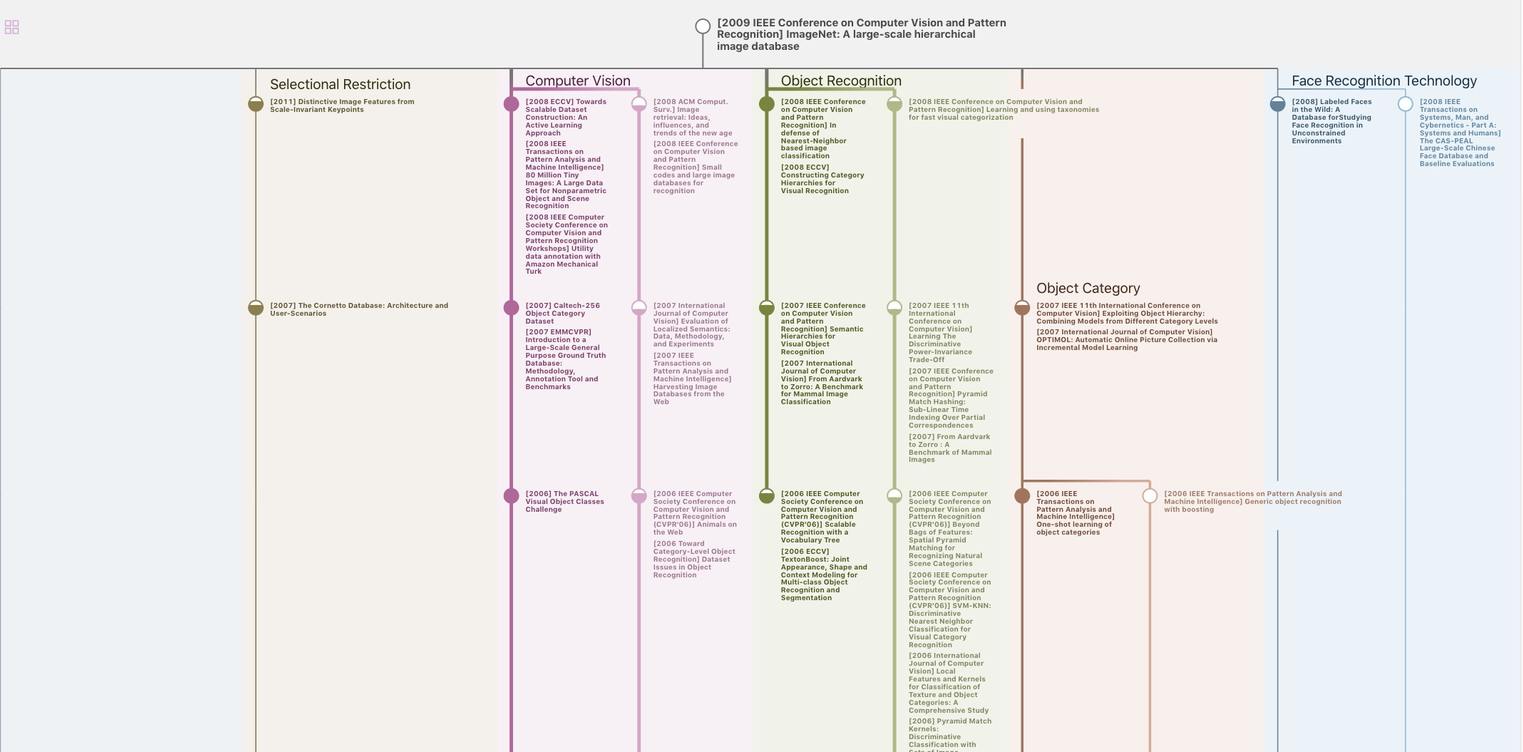
生成溯源树,研究论文发展脉络
Chat Paper
正在生成论文摘要