An Optimization-centric View on Bayes' Rule: Reviewing and Generalizing Variational Inference
J. Mach. Learn. Res.(2022)
摘要
We advocate an optimization-centric view of Bayesian inference. Our inspiration is the representation of Bayes' rule as infinite-dimensional optimization (Csiszar, 1975; Donsker and Varadhan, 1975; Zellner, 1988). Equipped with this perspective, we study Bayesian inference when one does not have access to (1) well-specified priors, (2) well-specified likelihoods, (3) infinite computing power. While these three assumptions underlie the standard Bayesian paradigm, they are typically inappropriate for modern Machine Learning applications. We propose addressing this through an optimization-centric generalization of Bayesian posteriors that we call the Rule of Three (ROT). The ROT can be justified axiomatically and recovers Bayesian, PAC-Bayesian and VI posteriors as special cases. While the ROT is primarily a conceptual and theoretical device, it also encompasses a novel sub-class of tractable posteriors which we call Generalized Variational Inference (GVI) posteriors. Just as the ROT, GVI posteriors are specified by three arguments: a loss, a divergence and a variational family. They also possess a number of desirable properties, including modularity, Frequentist consistency and an interpretation as approximate ELBO. We explore applications of GVI posteriors, and show that they can be used to improve robustness and posterior marginals on Bayesian Neural Networks and Deep Gaussian Processes.
更多查看译文
关键词
Bayesian Inference, Generalized Bayesian Inference, Variational Inference, Bayesian Neural Networks, Deep Gaussian Proceses
AI 理解论文
溯源树
样例
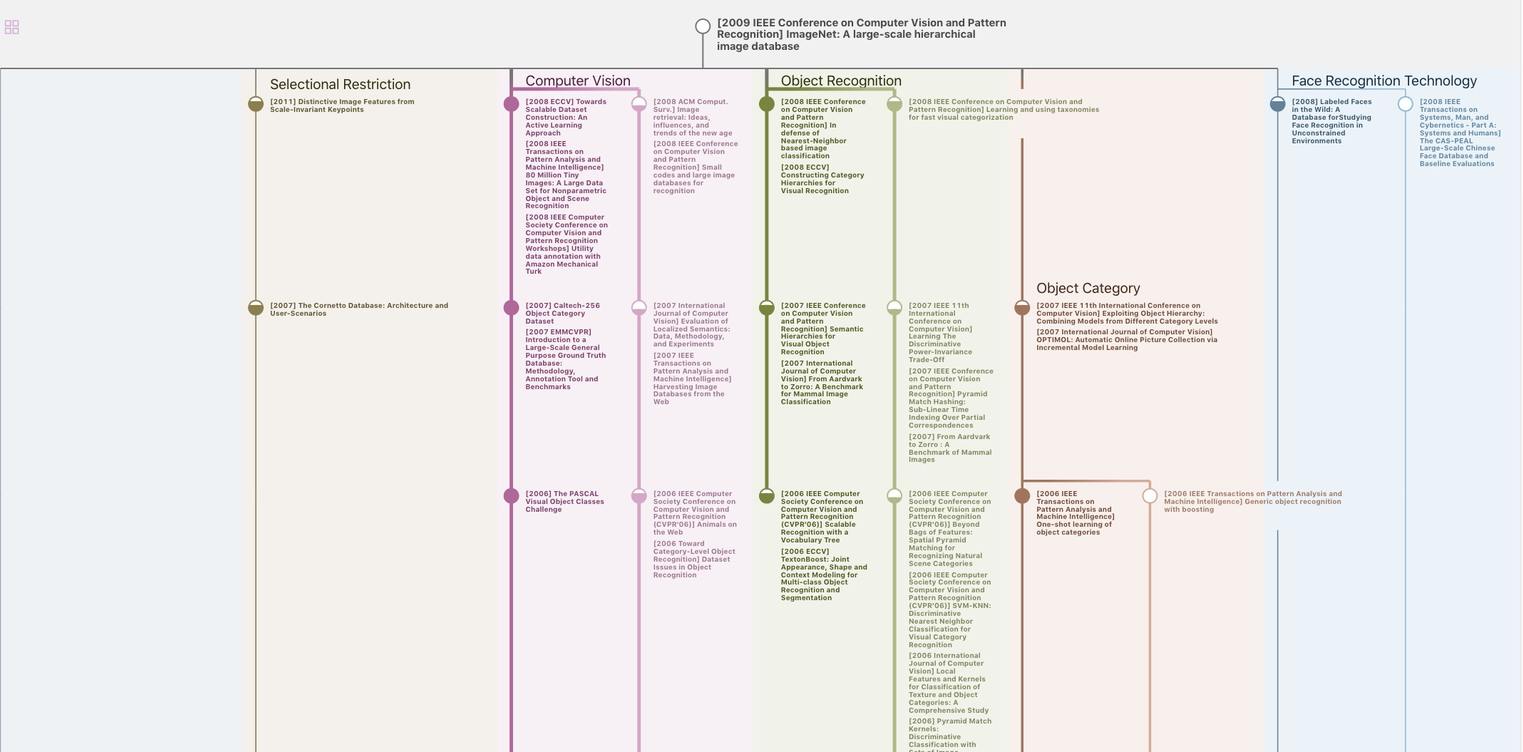
生成溯源树,研究论文发展脉络
Chat Paper
正在生成论文摘要