An efficient model for real-time wildfire detection in complex scenarios based on multi-head attention mechanism
J. Real Time Image Process.(2023)
摘要
Wildfire is a common natural disaster that destroys the ecological environment and endangers the lives and property of people. The average precision and speed of wildfire detection algorithms are critical in complex wildfire detection scenarios. However, the complex wildfire scenarios and multi-scale flaming objects decrease the detection accuracy of the model. How to design an accurate and fast wildfire detection model is a challenging study. To improve the average detection precision of the model while reducing its computational cost as much as possible, we propose an efficient model for real-time wildfire detection in complex scenarios called FireDetn. First, we use four different detection heads to help FireDetn detect different size flame objects. Then, we integrate Transformer Encoder blocks with multi-head attention in FireDetn to enhance its ability to capture global feature information and contextual information, thus improving its average precision in complex scenarios. Finally, we fuse the spatial pyramid pooling fast structure, which benefits in detecting multi-scale flame objects at a lower computational cost. Our experimental results show that the FireDetn can detect about 112 frames per second, meeting the requirement of real-time detection. We also compare FireDetn with other algorithms, demonstrating that FireDetn obtains satisfactory results in average precision and speed.
更多查看译文
关键词
Wildfire detection,Object detection,Deep learning,YOLO,Transformer
AI 理解论文
溯源树
样例
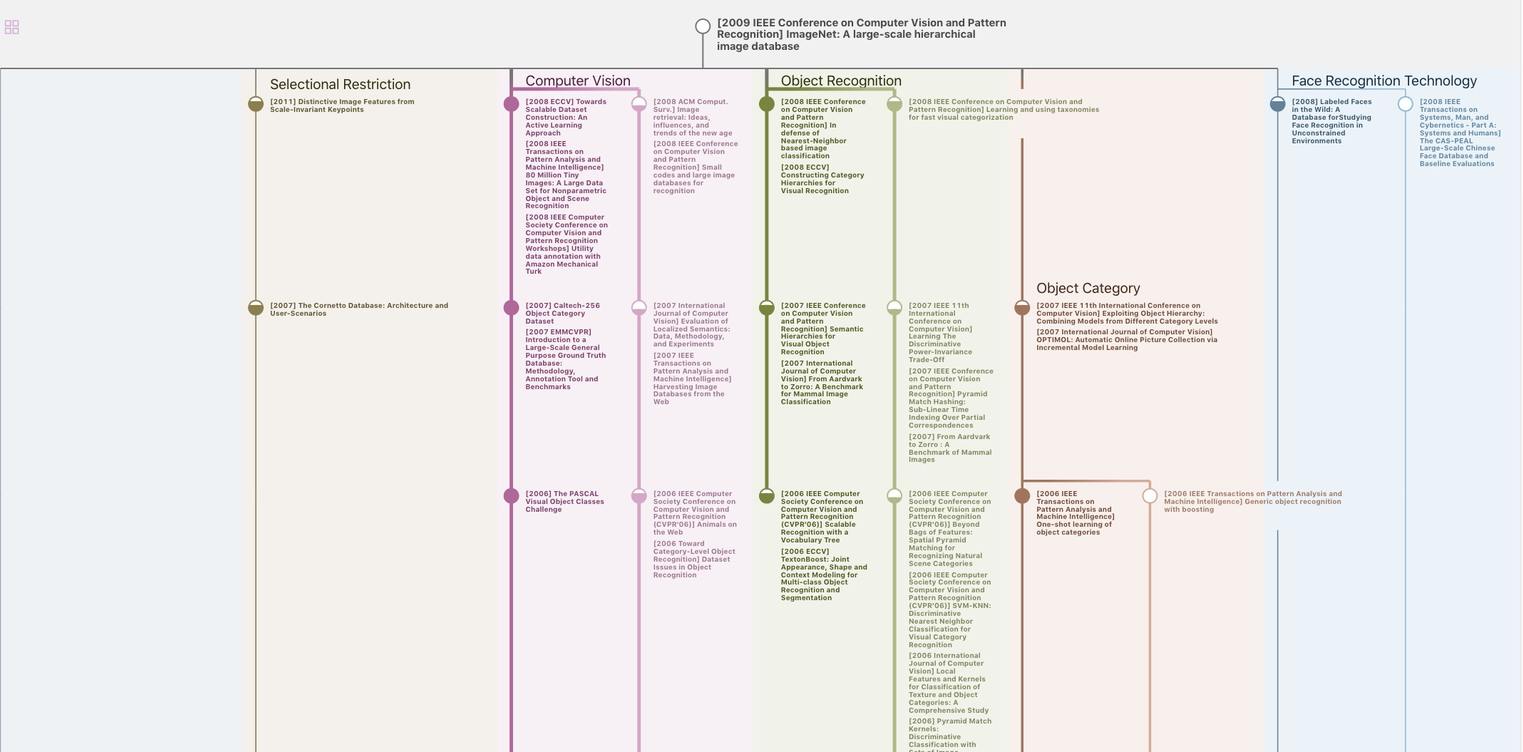
生成溯源树,研究论文发展脉络
Chat Paper
正在生成论文摘要