Assessing the Utility of Multiple Representations for Object Classification.
IEEE Trans. Geosci. Remote. Sens.(2023)
摘要
Classification of objects based on acoustic remote sensing is influenced by a variety of scattering phenomena, both in-air and underwater. Active sonar ensonification induces multiple types of acoustic scattering phenomena including direct geometric scattering as well as structural resonance. It is observed that the choice of representation for the raw measurement affects the shape and strength of discriminatory features and the performance of classifiers that utilizes them. Using in-air acoustic measurements collected in a noise-controlled laboratory setting, this work develops a statistical model for discriminatory features and a framework to identify the discriminatory pixels in multiple representations as well as an approach to quantify their discriminatory capacity in the presence of additive noise of varying levels. This framework is used to assess the utility and robustness of multiple representation for object classification over a wide range of noise levels (NLs), and to compare the relative classification performance bounds under independent pixels assumption as well as conventional feature energy detectors.
更多查看译文
关键词
Acoustic signal processing,classification,performance prediction,remote sensing,statistical model
AI 理解论文
溯源树
样例
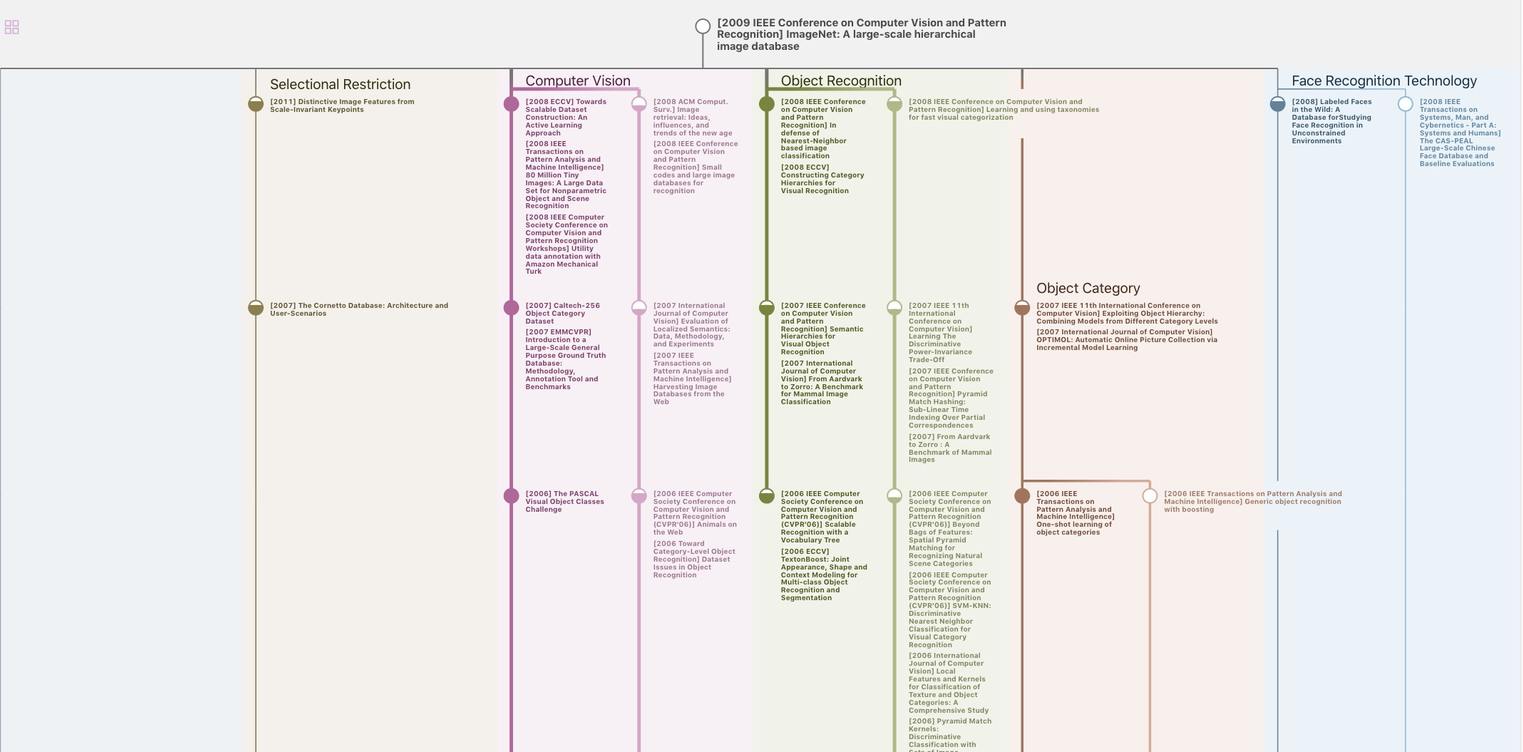
生成溯源树,研究论文发展脉络
Chat Paper
正在生成论文摘要