AIM: An Android Interpretable Malware detector based on application class modeling.
J. Inf. Secur. Appl.(2023)
摘要
Smartphones are one of the IoT gadgets that have revolutionized our lives. Perhaps the most significant threat that endangers the security of smartphones is mobile malware. Despite the recent efforts to combat smartphone malware, it remains one of the significant challenges in smartphone security. Modern smartphone malware is complex and growing at a rapid pace. Thus, techniques based on machine learning have gained significant popularity in recent years. However, most of the existing methods fail to provide enough interpretability regarding their decisions. In other words, if an application is classified as malicious, it is not clear which part of the application is performing the malicious behavior. In this work, we address this gap by presenting an Android Interpretable Malware detection method (AIM) based on application class modeling. AIM utilizes hybrid analysis and a neural network classifier to distinguish malware from benign applications. Furthermore, AIM identifies malicious parts of malware applications by utilizing a novel class modeling approach based on used APIs and employing the attention mechanism. We implement AIM and evaluate its performance on up-to-date benchmark datasets. We also compare the results obtained by the proposed technique with others and investigate the impact of several feature sets on it. The results indicate that AIM outperforms most of the existing methods with an accuracy of more than 98.9% and correctly highlights malicious code snippets inside applications.
更多查看译文
关键词
Android,Malware detection,Neural networks,Hybrid analysis
AI 理解论文
溯源树
样例
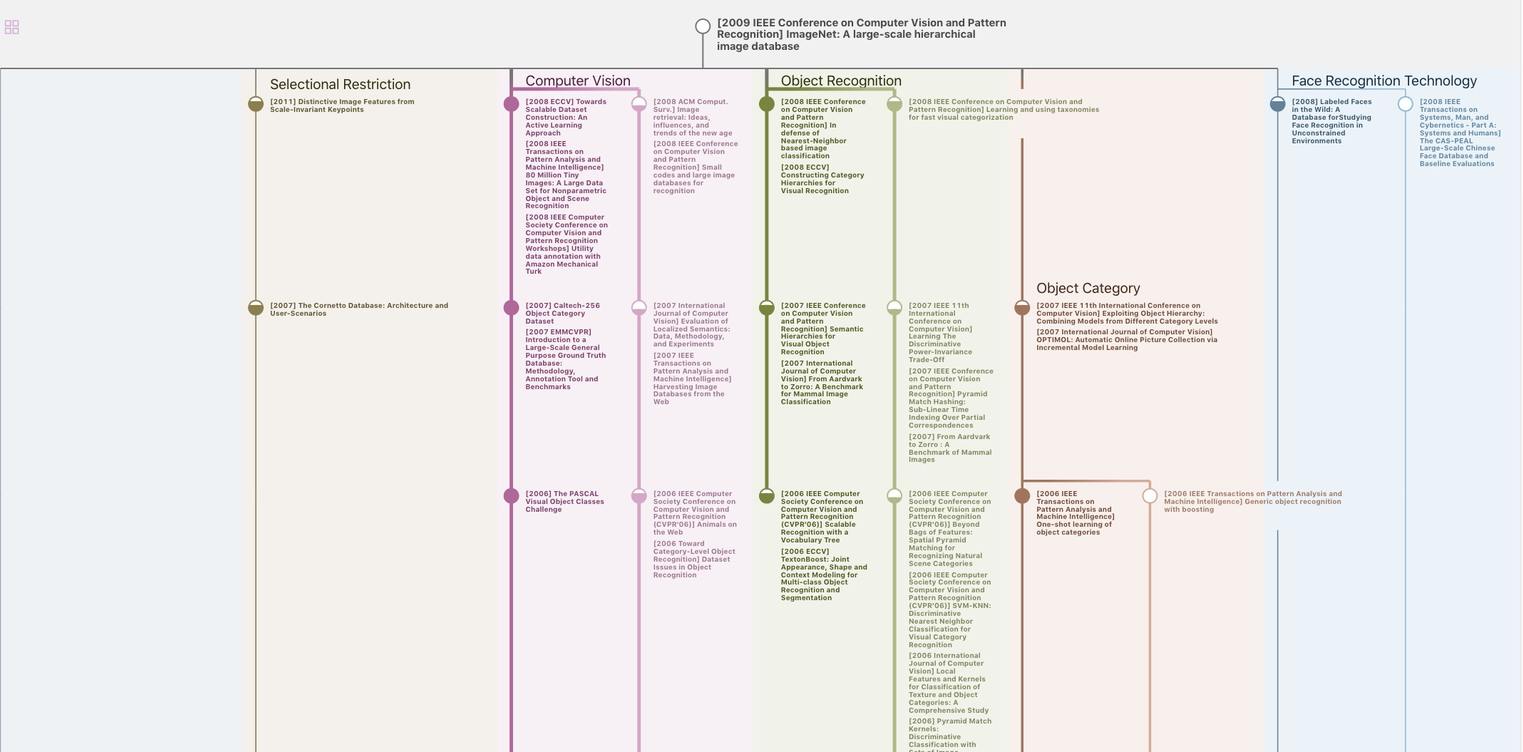
生成溯源树,研究论文发展脉络
Chat Paper
正在生成论文摘要