Pairwise contrastive learning for sentence semantic equivalence identification with limited supervision.
Knowl. Based Syst.(2023)
摘要
Sentence semantic equivalence identification (SSEI) targets to measure the semantic equivalence between two sentences. To supplement limited supervision, existing methods extensively employ contrastive learning to obtain sentence semantics. Albeit considerable progress, traditional sentence-wise contrastive learning cannot grasp the diverse semantics in the polysemic sentence. To alleviate this multi-vocal issue, a Pairwise Contrastive learning method (named PairContrast) for SSEI is developed in this study to imitate the pairwise and intercompared scenarios. Specifically, two unlabelled sentences in any anchor pair are first augmented with an enhanced augmentation strategy to generate three augmented pairs. To reduce augmentation noise, a pair mix-up strategy is also employed to merge these augmented pairs into an anchor-positive pair, which is further combined with the anchor pair to pretrain the interaction module through contrastive learning. Finally, the pretrained SSEI model is finetuned on limited supervision by the binary cross entropy objective. Experiments on two publicly available SSEI datasets demonstrate the superiority of PairContrast against state-of-the-art baselines. The robustness of PairContrast under different scales of limited supervision is also verified.
更多查看译文
关键词
Contrastive learning, Text matching, Sentence semantic, Equivalence identification, Limited supervision
AI 理解论文
溯源树
样例
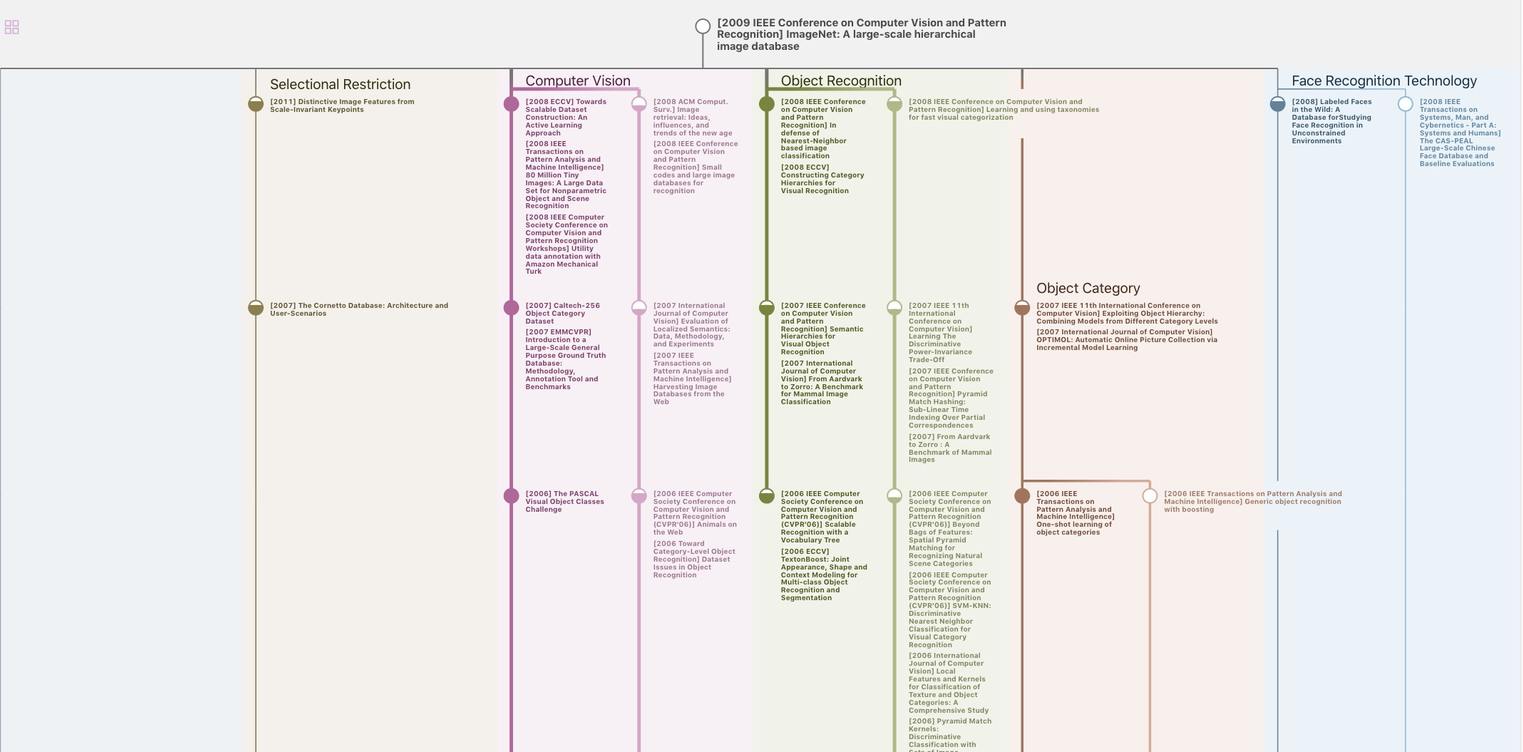
生成溯源树,研究论文发展脉络
Chat Paper
正在生成论文摘要