Multi-MCCR: Multiple models regularization for semi-supervised text classification with few labels
Knowl. Based Syst.(2023)
摘要
Semi-supervised learning has achieved impressive results and is commonly applied in text classifica-tions. However, in situations where labeled texts are exceedingly limited, neural networks are prone to over-fitting due to the non-negligible inconsistency between model training and inference caused by dropout mechanisms that randomly mask some neurons. To alleviate this inconsistency problem, we propose a simple Multiple Models Contrast learning based on Consistent Regularization, named Multi-MCCR, which consists of multiple models with the same structure and a C-BiKL loss strategy. Specifically, one sample first goes through multiple identical models to obtain multiple different output distributions, which enriches the sample output distributions and provides conditions for subsequent consistency approximation. Then, the C-BiKL loss strategy is proposed to minimize the combination of the bidirectional Kullback--Leibler (BiKL) divergence between the above multiple output distributions and the Cross-Entropy loss on labeled data, which provides consistency constraints (BiKL) for the model and simultaneously ensures correct classification (Cross-Entropy). Through the above setting of multi-model contrast learning, the inconsistency caused by the randomness of dropout between model training and inference is alleviated, thereby avoiding over-fitting and improving the classification ability in scenarios with limited labeled samples. We conducted experiments on six widely-used text classification datasets, including sentiment analysis, topic categorization, and reviews classification, and the experimental results show that our method is universally effective in semi-supervised text classification with limited labeled texts. (c) 2023 Elsevier B.V. All rights reserved.
更多查看译文
关键词
Semi-supervised learning,Multiple models contrast learning,Consistent regularization,Text classification
AI 理解论文
溯源树
样例
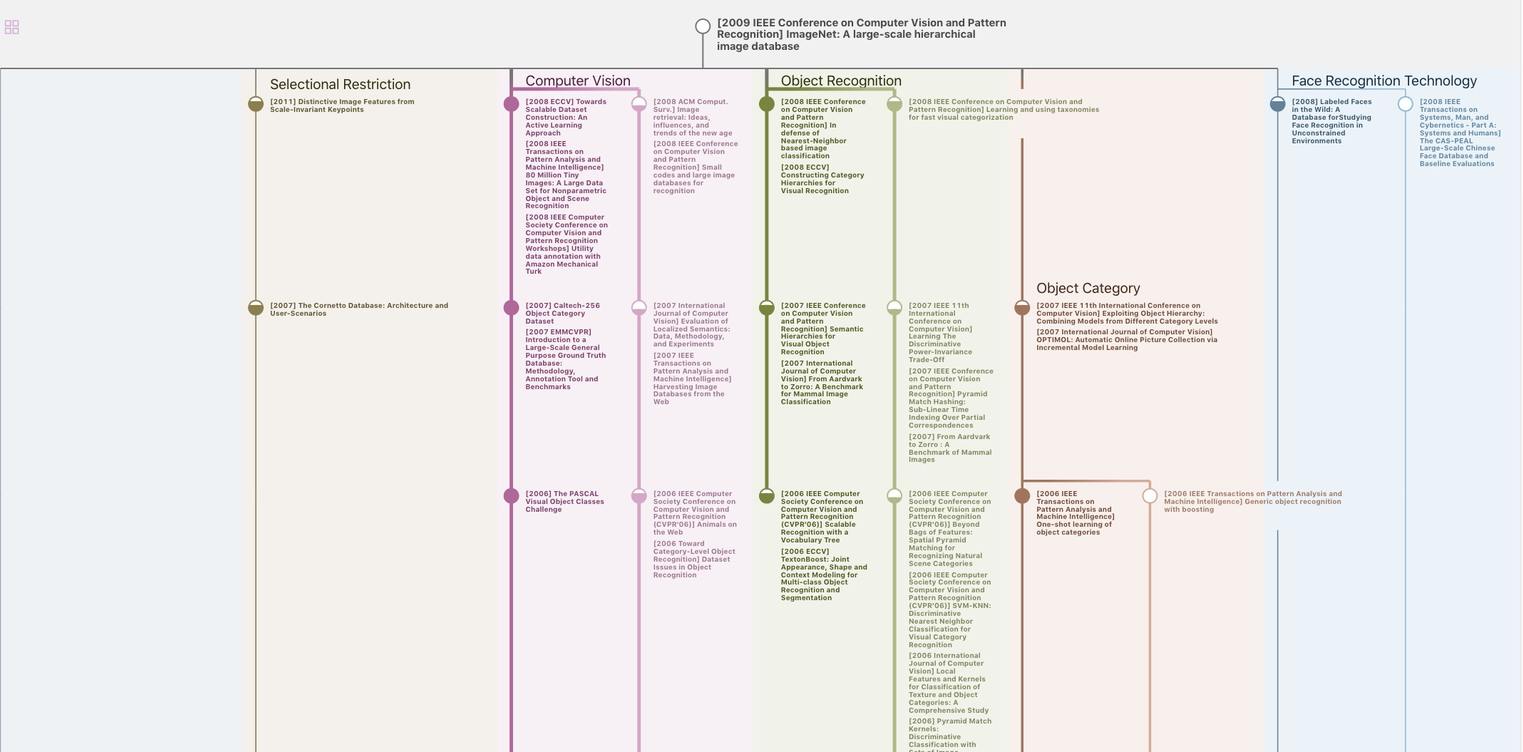
生成溯源树,研究论文发展脉络
Chat Paper
正在生成论文摘要