MFCCGAN: A Novel MFCC-Based Speech Synthesizer Using Adversarial Learning.
CoRR(2023)
摘要
In this paper, we introduce MFCCGAN as a novel speech synthesizer based on adversarial learning that adopts MFCCs as input and generates raw speech waveforms. Benefiting the GAN model capabilities, it produces speech with higher intelligibility than a rule-based MFCC-based speech synthesizer WORLD. We evaluated the model based on a popular intrusive objective speech intelligibility measure (STOI) and quality (NISQA score). Experimental results show that our proposed system outperforms Librosa MFCC-inversion (by an increase of about 26% up to 53% in STOI and 16% up to 78% in NISQA score) and a rise of about 10% in intelligibility and about 4% in naturalness in comparison with conventional rule-based vocoder WORLD that used in the CycleGAN-VC family. However, WORLD needs additional data like F0. Finally, using perceptual loss in discriminators based on STOI could improve the quality more. WebMUSHRA-based subjective tests also show the quality of the proposed approach.
更多查看译文
关键词
Index Terms- MFCC feature inversion,speech coding,speech synthesis,generative adversarial learning,perceptual optimization
AI 理解论文
溯源树
样例
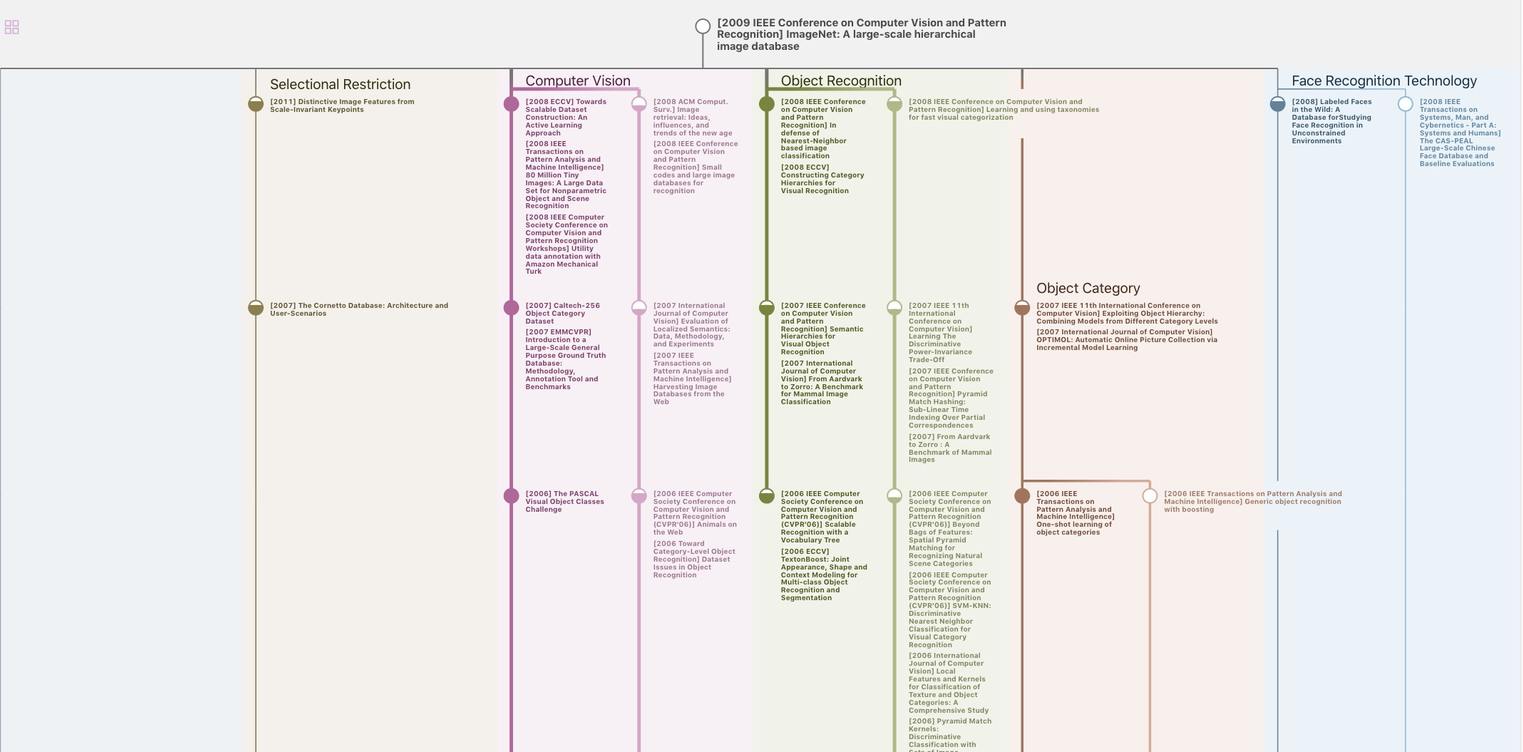
生成溯源树,研究论文发展脉络
Chat Paper
正在生成论文摘要