Robustness assessment and enhancement of deep reinforcement learning-enabled load restoration for distribution systems.
Reliab. Eng. Syst. Saf.(2023)
摘要
Efficient critical load restoration under extreme natural disasters is a promising solution to establish resilient distribution systems. Deep reinforcement learning (DRL) approaches are widely adopted in the load restoration problem to avoid incorporating the accurate distribution system model and improve online decision efficiency. However, the vulnerability of DRL towards adversarial examples may lead to unpracticable decisions and pose potential threats to load restoration. To address this issue, this paper proposes a robustness assessment and enhancement method for DRL-enabled distribution system load restoration. In particular, the distribution system load restoration problem is formulated as a Markov decision process, and a deep Q-network is adopted to learn the optimal decision policy. Then, an adversarial example generation optimization model incorporating the deep Q-network is established to implement the robustness assessment of the DRL-enabled load restoration against adversarial examples. Furthermore, adversarial training with the experience replay of adversarial examples is adopted to retrain the agent and improve the stability of the load restoration decision-making. Finally, the effectiveness of the proposed method is analyzed and verified in the modified IEEE 33-bus system and IEEE 123-bus system. The results show that robustness evaluation and enhancement significantly reduce the application risk of DRL in load restoration with safety-critical requirements.
更多查看译文
关键词
load restoration,distribution systems,learning-enabled
AI 理解论文
溯源树
样例
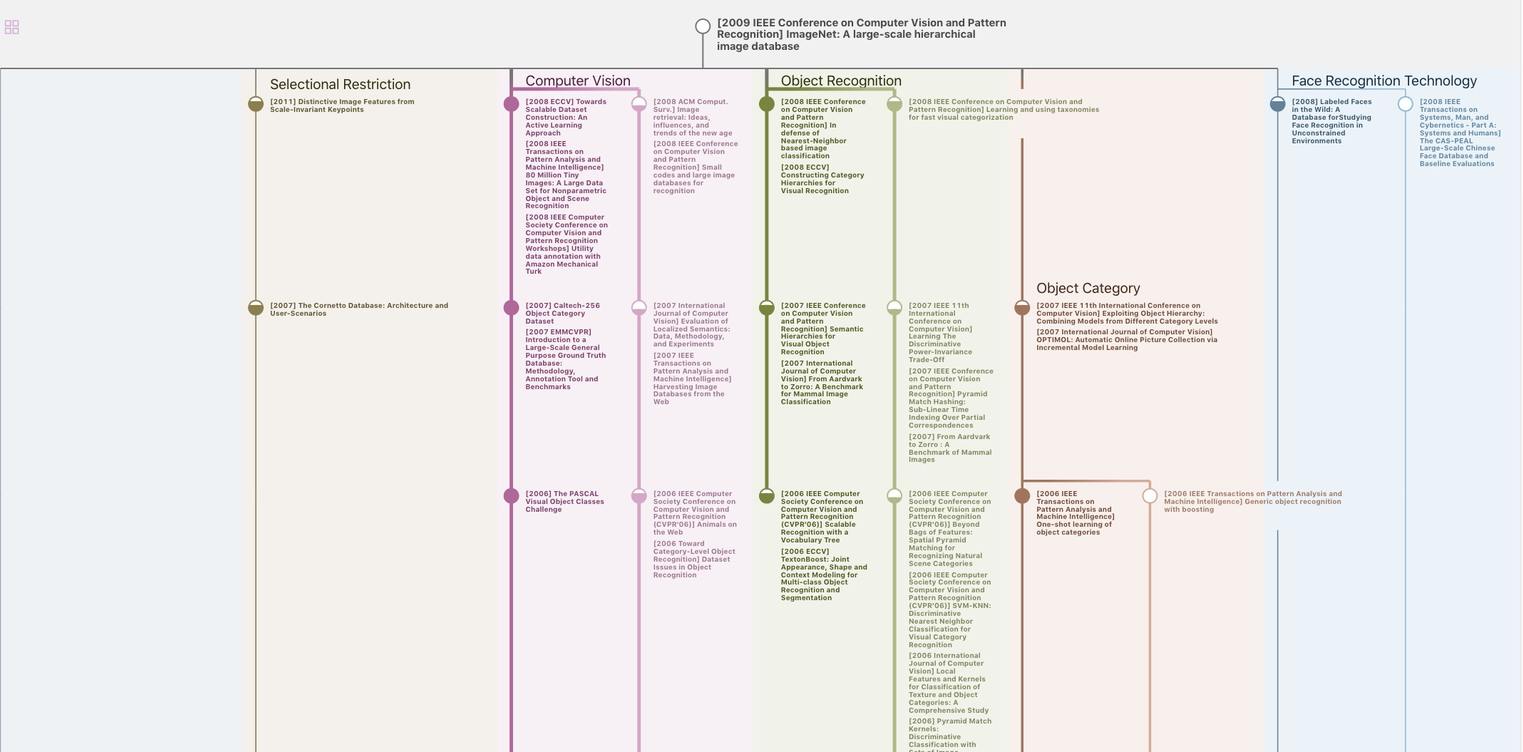
生成溯源树,研究论文发展脉络
Chat Paper
正在生成论文摘要