Locally private estimation of conditional probability distribution for random forest in multimedia applications.
Inf. Sci.(2023)
摘要
The application of artificial intelligence models to raw multimedia data is susceptible to various data inference attacks, posing a significant risk in terms of sensitive input information leakage. Most of the existing studies on privacy-preserving multimedia applications based on artificial intelligence, focus on a single intelligent model and thus have various limitations. In this paper, we attempt to directly perturb the core component of many multimedia intelligent models in Bayesian networks and deep learning. That is, we apply conditional probability distribution estimation to guarantee the privacy of the models. At first, we present the formal problem formulation of private conditional probability distribution estimation and apply it to random forest for task classification in multimedia applications. Then, we design a simple perturbation approach called NaivePrivDistEst, to add noise to all the elements of probability estimation in random forest. Next, we present an improved approach called FastLRG, that utilizes the taxonomy tree to discretize the continuous attributes, thereby combining the attribute features to improve the prediction accuracy of random forest. Finally, we perform extensive experiments to evaluate the performance of random forest based on the proposed estimation algorithms. The experimental results indicate that the proposed models have better performance compared with existing private decision trees.
更多查看译文
关键词
random forest,private estimation,conditional probability distribution,multimedia applications
AI 理解论文
溯源树
样例
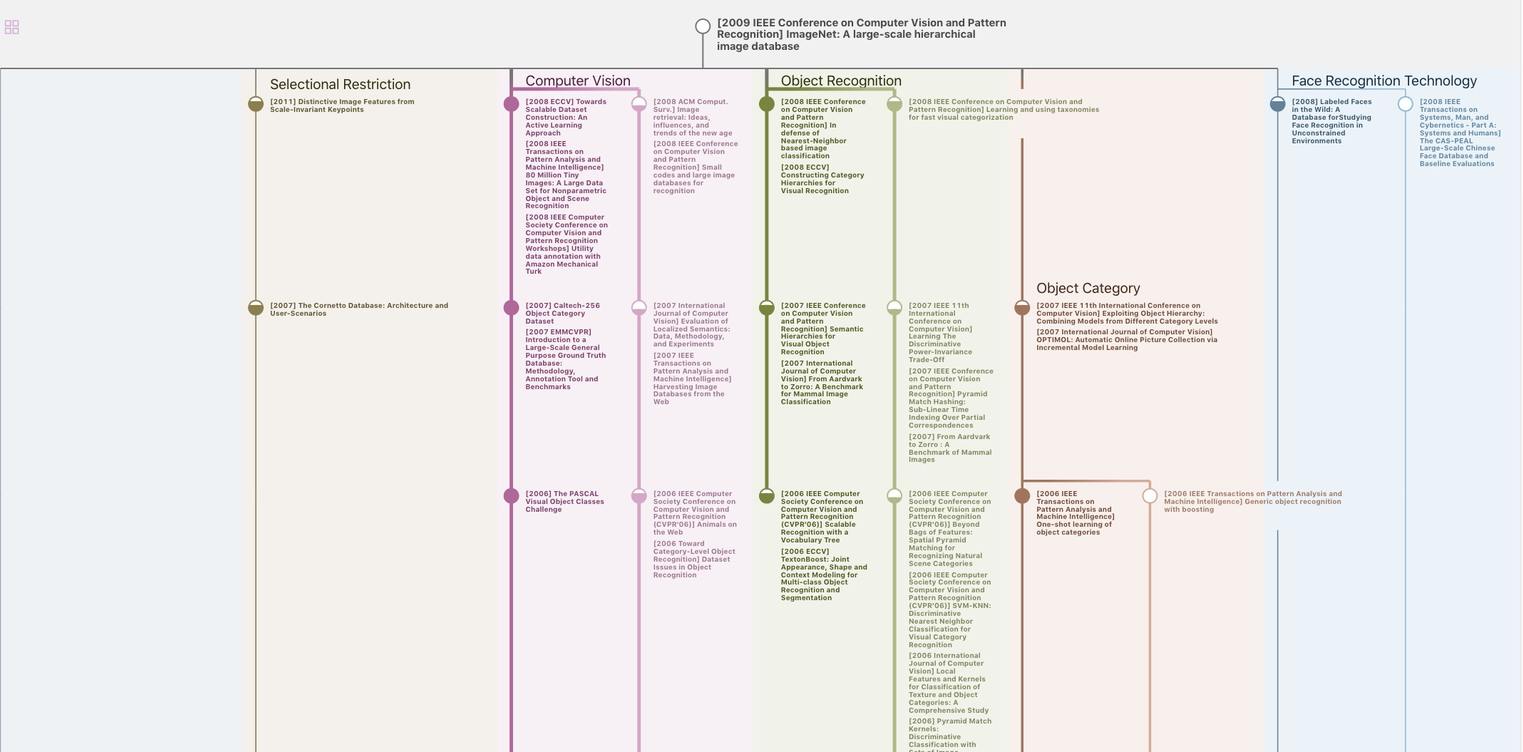
生成溯源树,研究论文发展脉络
Chat Paper
正在生成论文摘要