Distributed quantile regression in decentralized optimization.
Inf. Sci.(2023)
摘要
When dealing with massive data that is distributed across multiple servers, it is particularly important to solve the distributed learning problem while minimizing the communication cost between servers. In this paper, we investigate an estimation procedure based on the group alternating direction method of multipliers (GADMM) algorithm for computing distributed quantile regression models. Numerical experiments show that our proposed method has competitive performance in both communication cost and statistical computational efficiency. We also provide a real-world data application to demonstrate the superiority of our method.
更多查看译文
关键词
Decentralized learning, Distributed computing, Quantile regression, Massive data
AI 理解论文
溯源树
样例
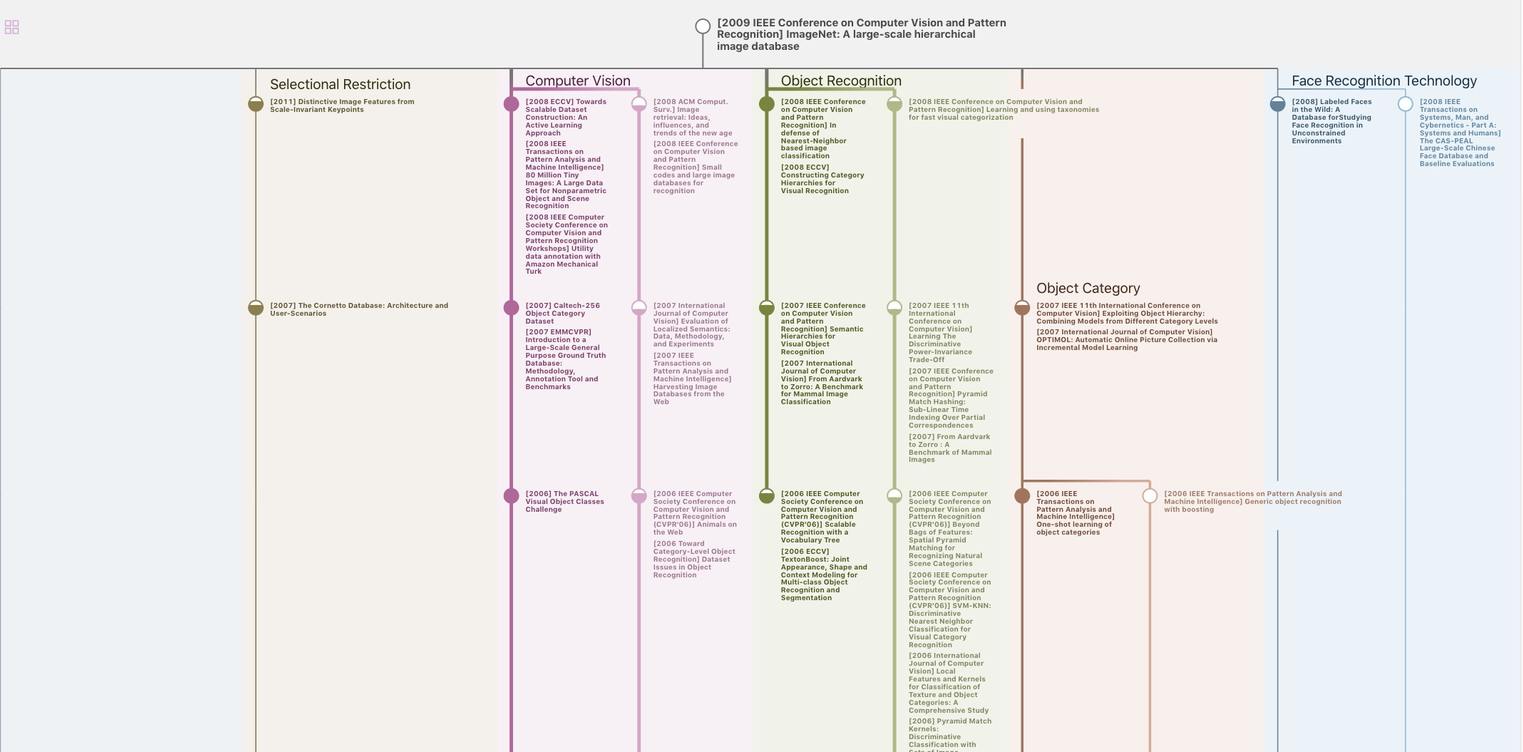
生成溯源树,研究论文发展脉络
Chat Paper
正在生成论文摘要