Intelligent fault diagnosis for triboelectric nanogenerators via a novel deep learning framework.
Expert Syst. Appl.(2023)
摘要
Triboelectric nanogenerators (TENGs) provide a new approach to converting mechanical energy into electric power. Although many researchers have made progress in the improvement of the electric output of TENG, the service life of the electronic components in TENG is generally shorter than that of the stator or rotor windings in conventional generators. Considering that the fault of electronic components in TENG results in a decline in the electric output, which may subsequently increase the usage cost and even endanger the users of the TENG-based self-power devices. Thus, it is necessary to provide parametric schemes for the high-reliable iterative optimization design of TENG by identifying the fault-susceptible components in TENG. This study proposes a novel deep learning framework based on an attention neural network (AttCNN) for the fault diagnosis of TENG. The proposed method integrates the attention mechanism and convolutional network. Therein, the global correlation features in voltage signals extracted by the attention mechanism can effectively reflect the status variation of TENG among different moments, and the feature information extracted by the convolutional network guarantees the fault diagnosis efficiency of TENG. Thereby the proposed method fills in the gaps in the field of fault diagnosis for TENG. In the case study, the fault diagnosis performance of the proposed method for an experimental TENG is analyzed. The results indicate that the predicted and real status of TENG are in good agreement, validating the effectiveness of the proposed method for the fault diagnosis of TENG. Moreover, the results reveal that the proposed method possesses a promising generalization performance, demonstrating the feasibility of the application of the proposed method for the real-time fault diagnosis of TENG. A comparative analysis illustrates that the performance of the proposed method is better than that of recently published data-driven methods in terms of fault diagnosis accuracy and efficiency. Hence, the results of the case study indicate that the proposed method has the characteristic of high efficiency, high accuracy, and well-generalization, thereby the application of the proposed method is conducive to the safe operation of TENG.
更多查看译文
关键词
Triboelectric nanogenerator,Intelligent fault diagnosis,Deep learning,Real-time
AI 理解论文
溯源树
样例
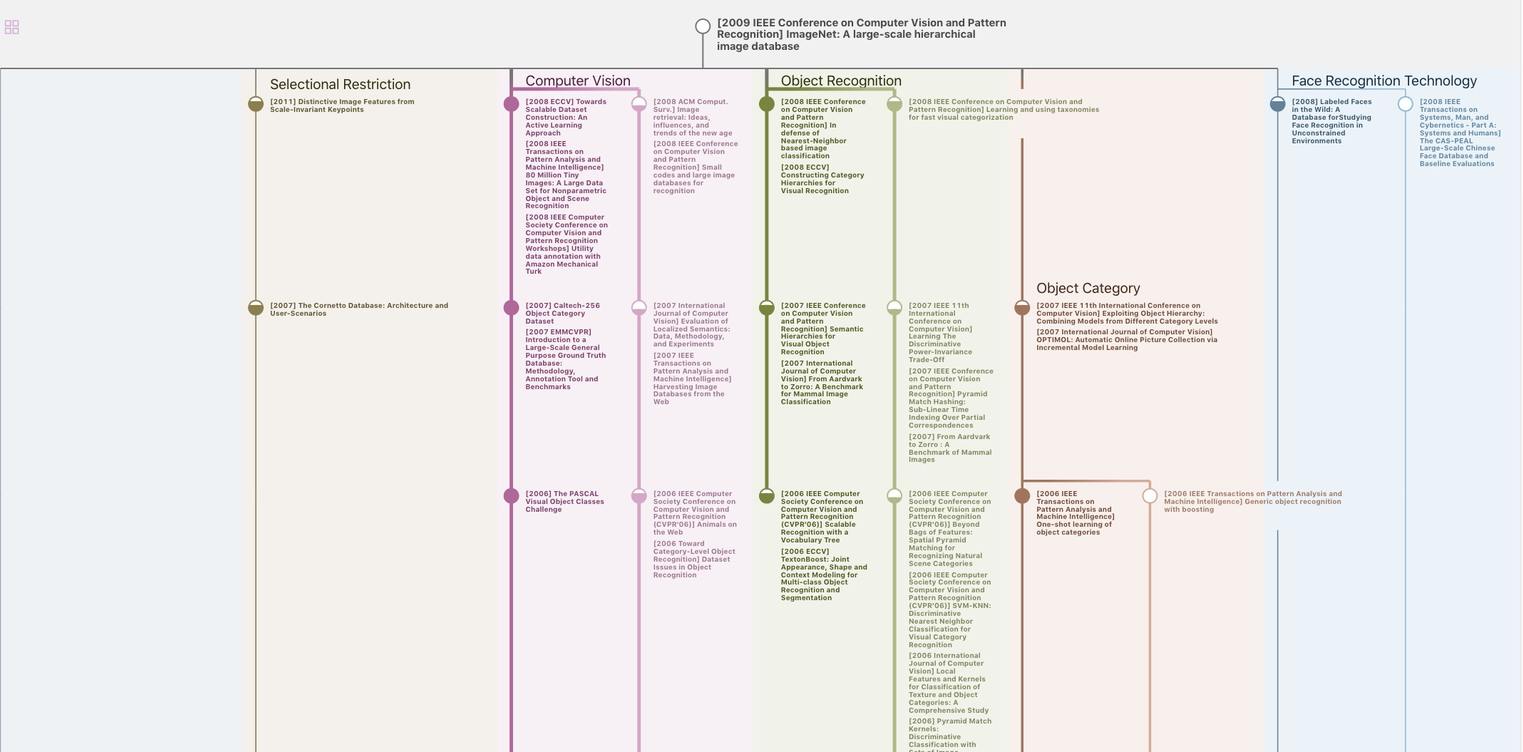
生成溯源树,研究论文发展脉络
Chat Paper
正在生成论文摘要