Multi-step reward ensemble methods for adaptive stock trading.
Expert Syst. Appl.(2023)
摘要
Stock trading can be considered a Markov decision process that comes naturally to applying reinforcement learning (RL) to this field. Numerous studies have proposed various methods to combine stock trading with RL, where only one single reward function is used to fit the market. However, the market in the real world shows distinct patterns in different periods, such as bullish or bearish. A reward function in bullish periods may perform poorly in bearish periods. In our work, we construct several kinds of multi-step future-price-based reward functions (profit-based reward and regularized-based reward), considering that the market changes consistently. Moreover, we propose two ensemble rewards based on the greedy method (MSR-GME, the abbreviation for Multi-Step Rewards Greedy Method Ensemble) and Thompson sampling (MSR-TSE, the abbreviation for Multi-Step Rewards Thompson Sampling Ensemble) to help agents to make adaptive trading decisions under distinct market patterns. We conduct extensive experiments to verify the mechanisms and the superiority of our constructed reward functions from multiple aspects. The results show the two constructed single-reward functions outperform both the buy-and-hold strategy (B&H) and the historical-price-based rewards consistently to a large extent (for example, the profit-based reward achieves at most 7.3 times the Sortino ratio and 78.6% lower maximum drawdown than B&H). Moreover, the ensemble rewards can substantially improve strategy performance in achieving higher profits and lower risks (for example, MSR-TSE achieves at most 49.7 times profits and 8.85 times Sortino ratio than B&H). We also find that MSR-TSE is risk-averse, but MSR-GME is risk-aggressive, indicating that Thompson sampling is an intensely competitive ensemble method, especially in bearish markets.
更多查看译文
关键词
Multi-step reward,Reward ensemble,Adaptive trading,Thompson sampling
AI 理解论文
溯源树
样例
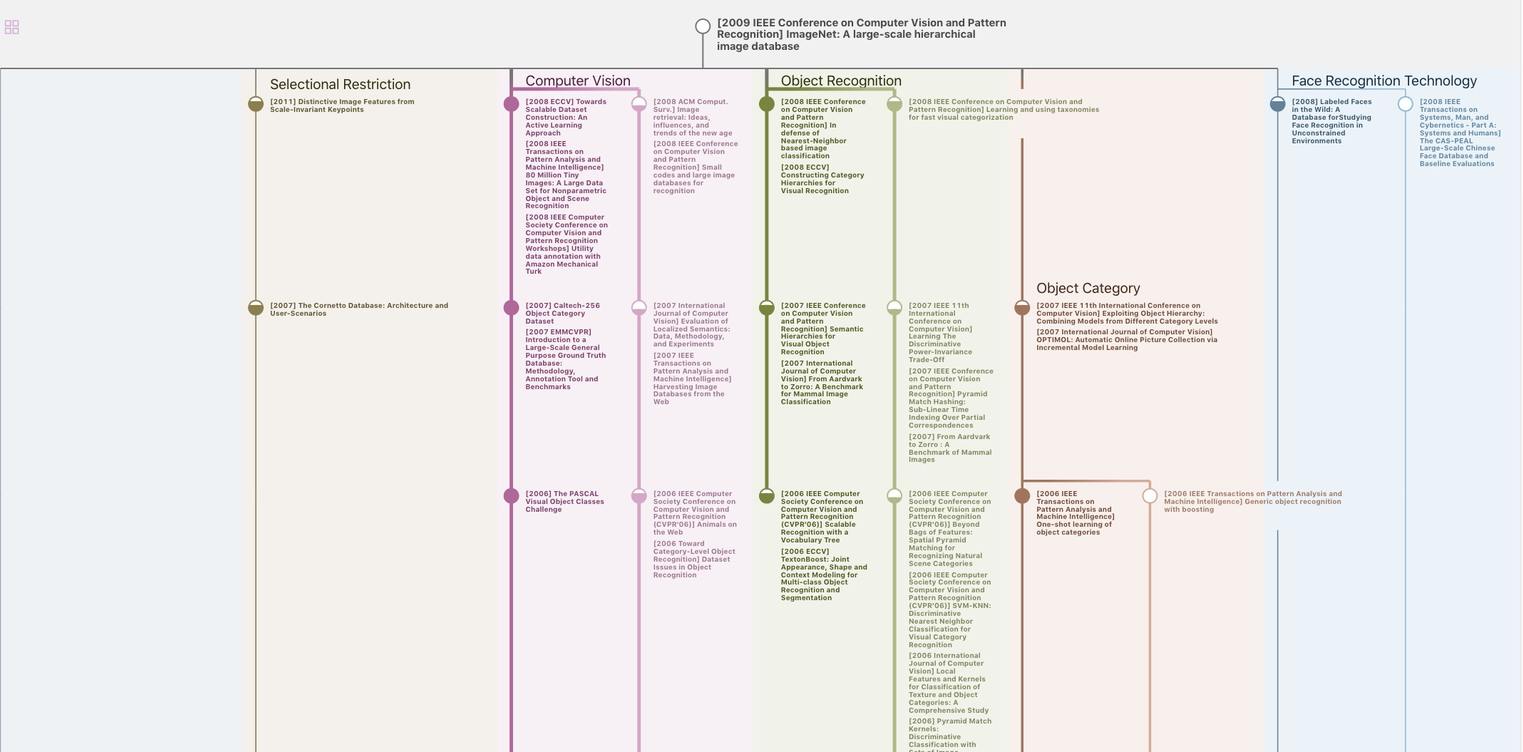
生成溯源树,研究论文发展脉络
Chat Paper
正在生成论文摘要