F3Net: Fast Fourier Filter Network for Hyperspectral Image Classification
IEEE Trans. Instrum. Meas.(2023)
摘要
In the hyperspectral image (HSI) classification, there are numerous deep learning-based research routes that have emerged recently. Among them, two methodologies attract our attention. One is convolutional neural network (CNN)-based classification, and the other is transformer-based classification. The essence of these two methodologies is to interchange information locally or at a long distance for HSI pixels in the spatial or spectral-spatial domain. There are two principles underlying this essence-the information mixing mechanism and the information mixing domain. Although both CNN-based and transformer-based have made efforts in these two principles and obtained favorable classification results, there is still room for improvement in terms of accuracy and efficiency. To further enhance the accuracy and efficiency under the two principles, fast Fourier transform (FFT) is introduced to HSI classification and a fast Fourier filter is designed to mix information efficiently in the frequency domain by means of FFT. The parametric-free characteristic and fast computation of FFT can assist us in efficiently learning interactions among features in the frequency domain. Furthermore, a fast Fourier filter block ({F3 Block) is built upon the fast Fourier filter for repeatedly using as a basic block. In addition, we propose a spectral-spatial convolution tokenizer (SSCT) to extract shallow features and prepare spectral-spatial tokens for F3 Blocks. Finally, by employing SSCT and F3 Blocks, a novel deep neural network architecture-fast Fourier filter network (F3 Net), is proposed for HSI classification.F3 Net-P as a pyramidal variant of F3 Net is also investigated. The experimental results on four datasets comprehensively evaluate our models and indicate that they are competitive with several current state-of-the-art methods, especially when the training samples are extremely limited. Specifically, F3Net-P achieves the highest accuracy of 97.25%, 98.08%, 97.49%, and 97.95% on the four datasets, respectively, outperforming second best compared model by 1.49%, 2.03%, 2.14%, and 1.94%.
更多查看译文
关键词
Convolutional neural network (CNN),factor analysis (FA),Fourier transform,hyperspectral image (HSI) classification,transformer
AI 理解论文
溯源树
样例
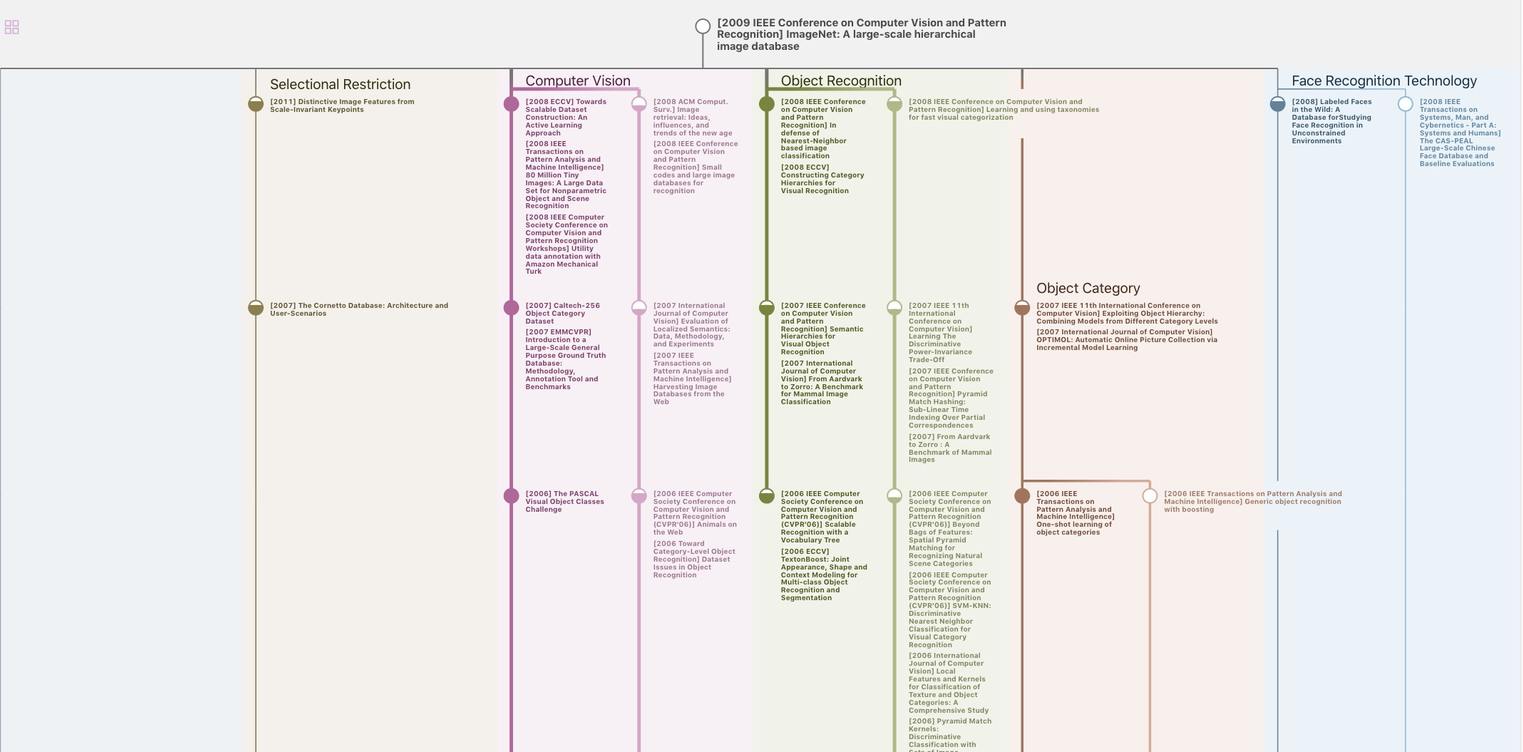
生成溯源树,研究论文发展脉络
Chat Paper
正在生成论文摘要