Understanding Semantics in Feature Selection for Fault Diagnosis in Network Telemetry Data.
NOMS(2023)
摘要
Expert systems for fault diagnosis are computationally expensive to build and maintain, and lack scalability and inherent adaptability to unknown events or modifications in the topology of the monitored system. While data-driven feature selection mechanisms can facilitate diagnosis without the hardship of developing and maintaining expert systems, purely data-driven mechanisms lack understanding of semantic importance within a feature set, and would benefit from additional domain knowledge. Part of this additional knowledge can be extracted from metadata. The proposed approach combines data-driven metrics and semantic information contained in the feature names to produce selections of features which best represent an underlying event. This study extends a cross entropy based optimization method to join semantic importance with data behavior. A benchmarking architecture is introduced to evaluate the benefits of semantic analysis, and demonstrate the performance and robustness of semantic feature selection on different types of faults in network telemetry datasets, modeled with the YANG data modeling language. The results illustrate the interest of such a complementary meta-data analysis for data-driven fault diagnosis, and highlight the robustness of the studied approach against variations in the input feature set.
更多查看译文
关键词
feature selection,fault diagnosis
AI 理解论文
溯源树
样例
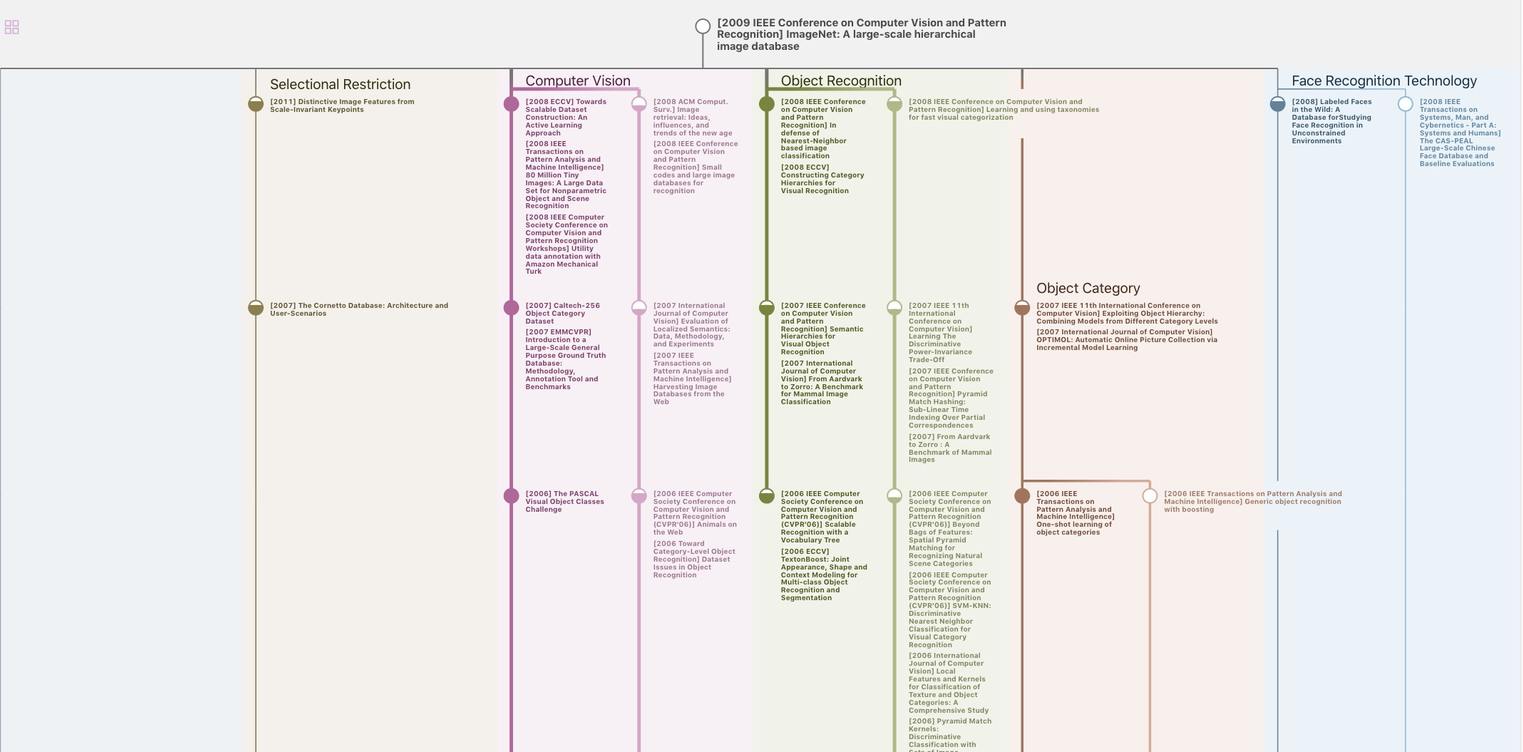
生成溯源树,研究论文发展脉络
Chat Paper
正在生成论文摘要