Componentized Task Scheduling in Cloud-Edge Cooperative Scenarios Based on GNN-enhanced DRL
NOMS(2023)
摘要
With the continuous functional enhancement of network services, a service usually presents a directed acyclic graphic (DAG) structure. This paper models the DAG task scheduling problem as a multi-objective optimization problem to balance the task execution efficiency, network traffic, and system load balance in componentized task deployment. To produce an instant decision, we propose the Cloud-edge Collaborative Task Scheduling (CCTS) Algorithm based on hybrid reward architecture deep reinforcement learning (DRL). Specifically, to reduce the redundancy of the state space of the Markov decision process, we use directed graph convolution networks and graph convolution networks (GCN) to embed the directed task graph and undirected network graph, respectively. Simulation results show that the proposed method outperforms the compared convolutional neural networks and GCN-based DRL schemes in reducing the system latency, energy cost, network traffic, and load balance.
更多查看译文
关键词
cloud-edge collaboration,DAG task,reinforcement learning,graph neural network
AI 理解论文
溯源树
样例
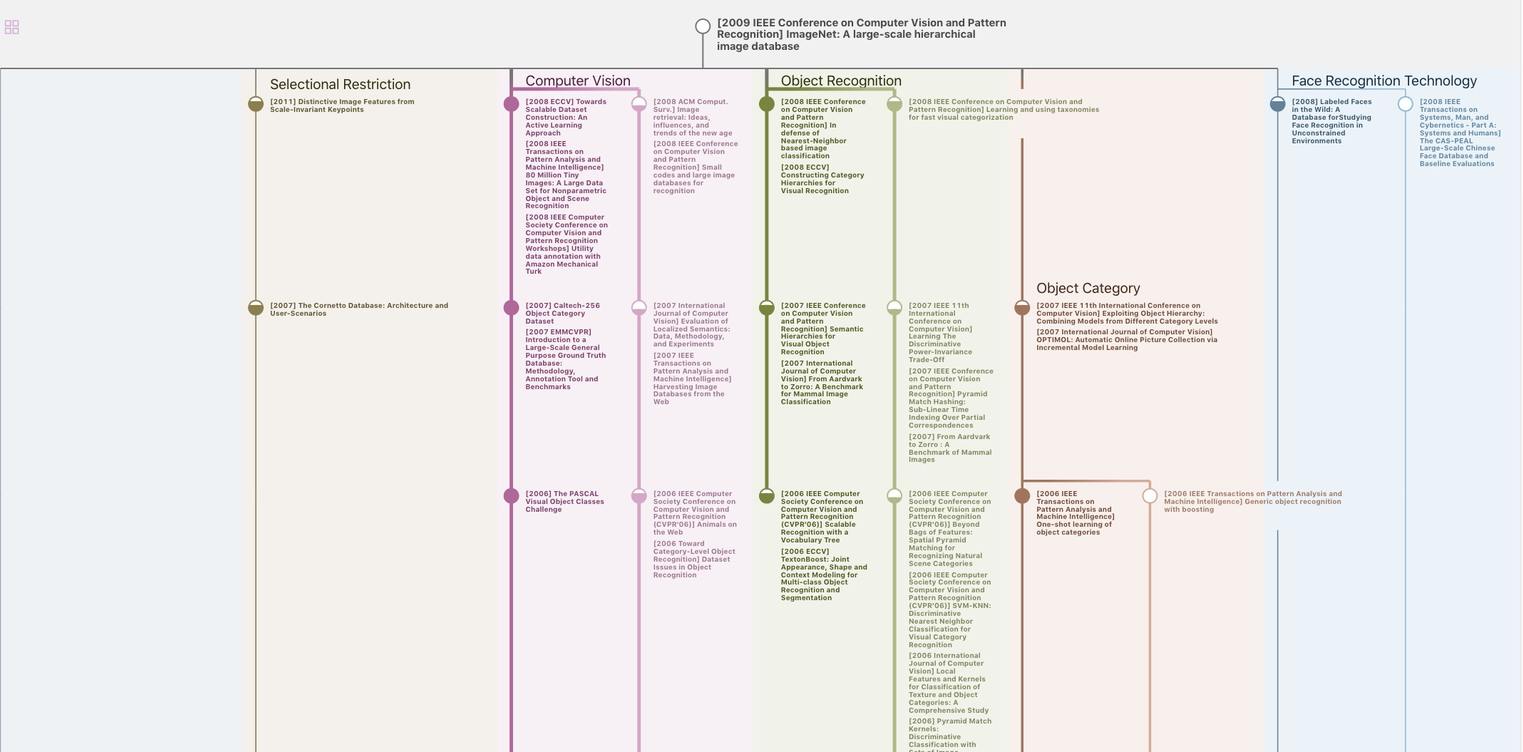
生成溯源树,研究论文发展脉络
Chat Paper
正在生成论文摘要