Self-adaptive and Efficient Training Node Selection for Federated Learning in B5G/6G Edge Network.
NOMS(2023)
摘要
In the upcoming B5G/6G era, devices will generate a amount of heterogeneous data at the network edge. As a paradigm for implementing distributed and privacy-preserving machine learning (ML), Federated Learning (FL) has drawn great attention to secure data sharing in edge networks. However, FL takes too much time and communication resources to train and transmit model parameters, which is unaffordable for edge devices with limited capabilities. To achieve a trade-off between resource and efficiency, it is crucial to select appropriate training nodes. While existing works about node selection focus on the resources allocation and pay less attention to the node mobility and seamless service. In this paper, we considering mobility, computation capability, and transmission power of training nodes to minimize the FL system cost. We propose an algorithm and mechanism respectively for different scenarios of node speed. An algorithm based on Deep Reinforcement Learning (DRL) matches with stationary and low-speed training nodes. A heuristic mechanism is used for nodes with high mobility. Simulation results show that the proposed schemes select appropriate training nodes effectively, and reduce the system cost by up to 20%.
更多查看译文
关键词
6G,Edge Network,Federated Learning,node selection,Deep Reinforcement Learning
AI 理解论文
溯源树
样例
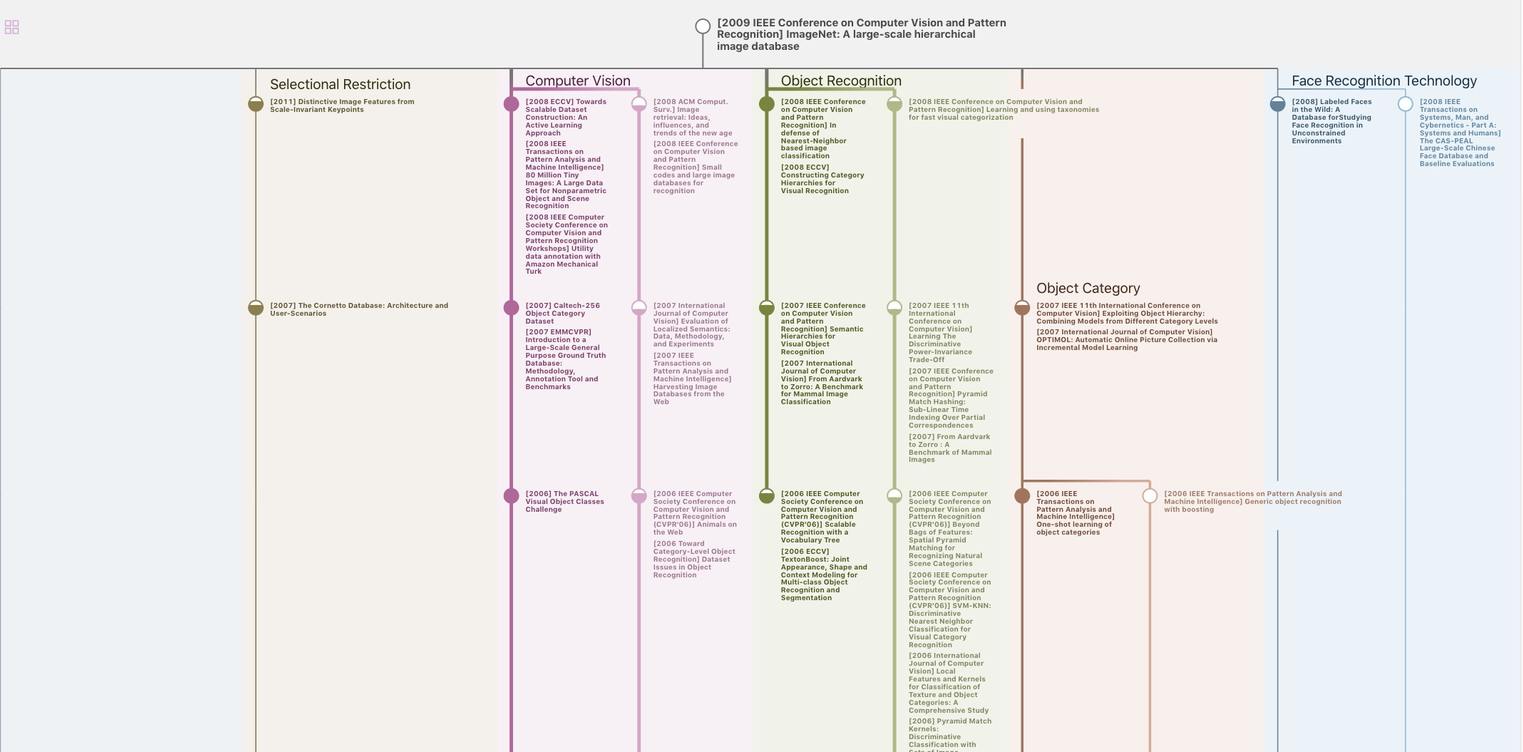
生成溯源树,研究论文发展脉络
Chat Paper
正在生成论文摘要