Addressing Popularity Bias in Recommender Systems: An Exploration of Self-Supervised Learning Models
2023 ADJUNCT PROCEEDINGS OF THE 31ST ACM CONFERENCE ON USER MODELING, ADAPTATION AND PERSONALIZATION, UMAP 2023(2023)
摘要
The rapid growth of the volume and variety of online media content has made it increasingly challenging for users to discover fresh content that meets their particular needs and tastes. Recommender Systems are digital tools that support users in navigating the plethora of available items. While these systems may offer several benefits, they may also create or reinforce certain undesired effects, including Popularity Bias, i.e., a short list of popular items becoming more popular while a long list of unpopular ones becoming even more unpopular. In this paper, we focus on this challenge and propose a novel recommendation approach that can generate accurate recommendations while effectively mitigating the popularity bias. Our proposed approach adopts models based on Self-Supervised Learning (SSL) that have recently drawn considerable attention in various application domains. Such models are known to enable recommender systems to exploit automatic mechanisms for data annotation hence providing self-supervisory signals for better training of the system from the available data. We considered various recommendation techniques based on the SSL model and compared their impact on popularity bias mitigation measured in terms of Average Recommendation Popularity (ARP), Gini-index, and Coverage. The results showed that SSL models could successfully mitigate the popularity bias while still maintaining the accuracy of the recommendation.
更多查看译文
关键词
Recommender systems,Self-supervised learning,Evaluation,Beyond accuracy
AI 理解论文
溯源树
样例
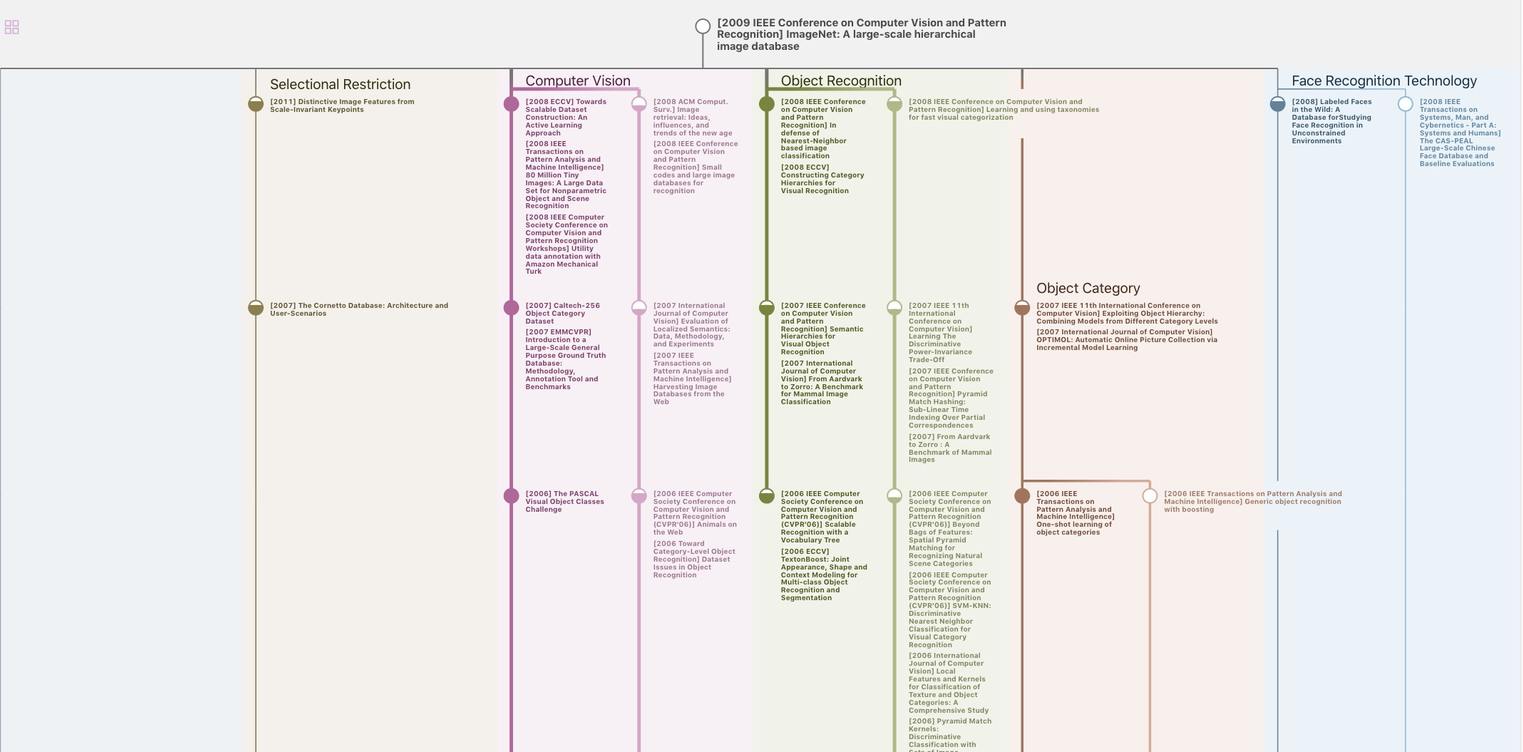
生成溯源树,研究论文发展脉络
Chat Paper
正在生成论文摘要