Invited Paper: Enhancing Privacy in Federated Learning via Early Exit.
ApPLIED@PODC(2023)
摘要
In this paper, we investigate the interplay between early exit mechanisms in deep neural networks and privacy preservation in the context of federated learning. Our primary objective is to assess how early exits impact privacy during the learning and inference phases. Through experiments, we demonstrate that models equipped with early exits perceivably boost privacy against membership inference attacks. Our findings suggest that the inclusion of early exits in neural models can serve as a valuable tool in mitigating privacy risks while, at the same time, retaining their original advantages of fast inference.
更多查看译文
AI 理解论文
溯源树
样例
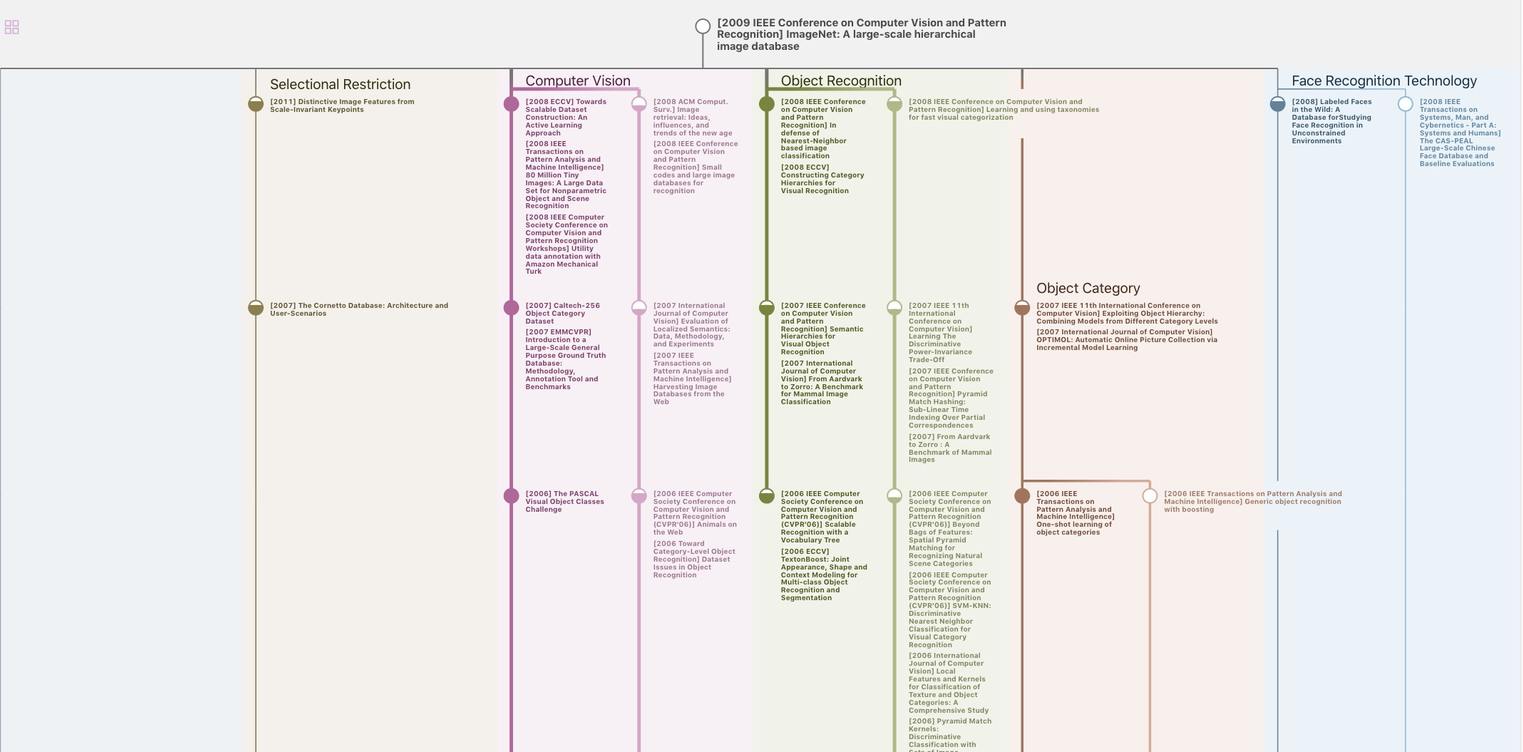
生成溯源树,研究论文发展脉络
Chat Paper
正在生成论文摘要