Optimizing Wireline Formation Testing in OilWells: A Data Science approach
SBSI(2023)
摘要
Context: Wireline Formation Testers are common operations in well testing and evaluation, as they provide key information for exploration and production activities, such as reservoir pressure and permeability. The operation is conducted by following a line graph, where the X and Y axes are time and pressure, respectively. Problem: The decision on whether to end or not the operation is based on this graph. Unfortunately, there is no consensus on the ideal method to conduct these operations, and that is precisely the objective of this work. Solution: Using mathematical modeling, optimization and data science techniques, this work aims to increase the efficiency of wireline formation testing, defining objective metrics for operations conductions, in order to reduce time and guarantee data quality.IS Theory: This work is associated with the Theory of Computational Learning, which aims to understand the fundamental principles of learning and design better-automated methods. Method: The research has a prescriptive character, following machine learning model building best-practices and using a quantitative approach in analyzing results. Summary of Results: The results obtained show that there is a clear potential for reducing operating time and, therefore, costs, if the proposed methodology is used in routine operations. Contributions and Impact in the IS area: This article shows that, through mathematical modeling and the application of data science techniques, it is possible to significantly reduce the time of Wireline Formation Test operations, without any relevant loss of information, which can be a significant gain for oil and gas companies.
更多查看译文
关键词
Formation test, wireline, well logging, formation pressure, optimization, machine learning
AI 理解论文
溯源树
样例
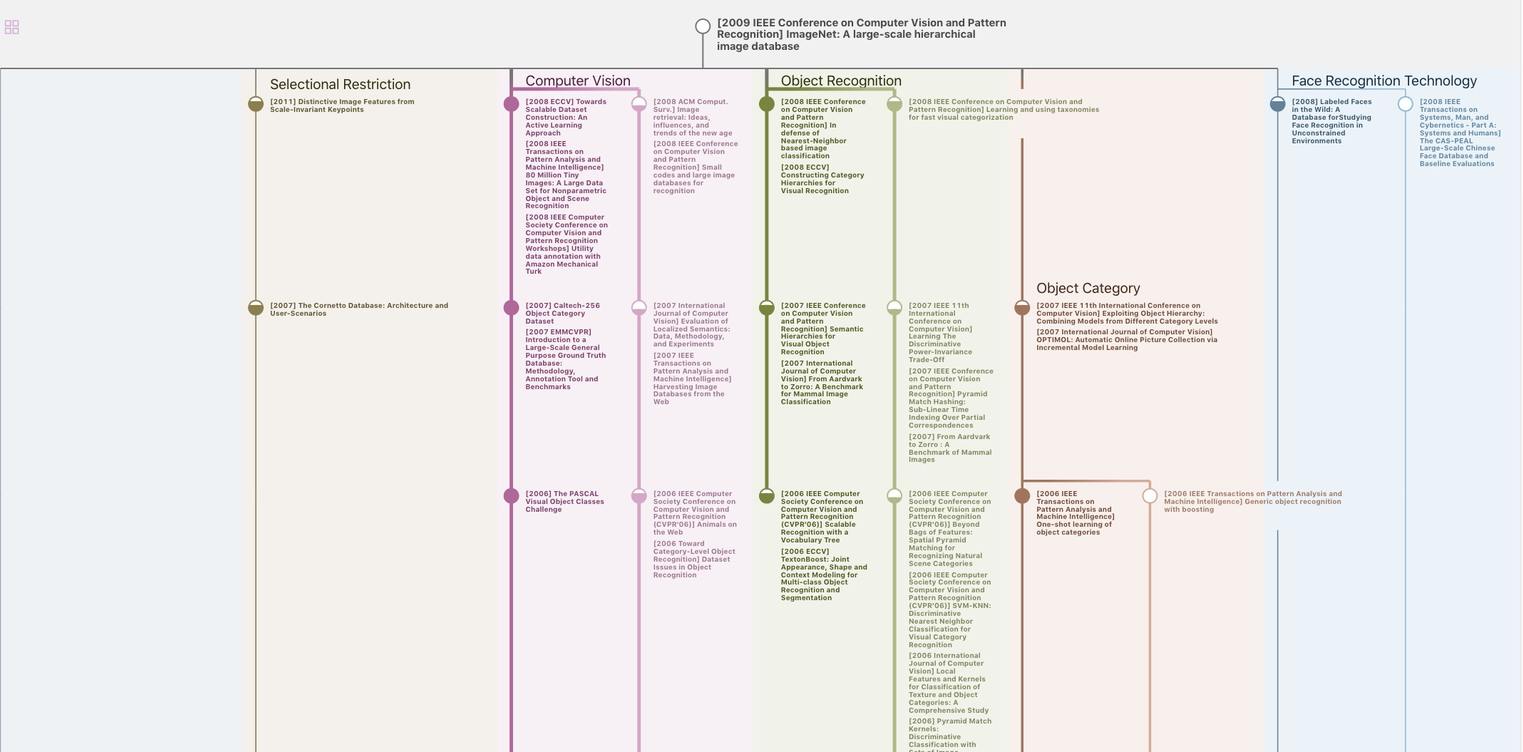
生成溯源树,研究论文发展脉络
Chat Paper
正在生成论文摘要