Efficient Hyperdimensional Learning with Trainable, Quantizable, and Holistic Data Representation
2023 DESIGN, AUTOMATION & TEST IN EUROPE CONFERENCE & EXHIBITION, DATE(2023)
摘要
Hyperdimensional computing (HDC) is a computing paradigm that draws inspiration from human memory models. It represents data in the form of high-dimensional vectors. Recently, many works in literature have tried to use HDC as a learning model due to its simple arithmetic and high efficiency. However, learning frameworks in HDC use encoders that are randomly generated and static, resulting in many parameters and low accuracy. In this paper, we propose TrainableHD, a framework for HDC that utilizes a dynamic encoder with effective quantization for higher efficiency. Our model considers errors gained from the HD model and dynamically updates the encoder during training. Our evaluations show that TrainableHD improves the accuracy of the HDC by up to 22.26% (on average 3.62%) without any extra computation costs, achieving a comparable level to state-of-the-art deep learning. Also, the proposed solution is 56.4x faster and 73x more energy efficient as compared to the deep learning on NVIDIA Jetson Xavier, a low-power GPU platform.
更多查看译文
关键词
Hyperdimensional Computing,Quantization,Alternative Computing,Data Representation
AI 理解论文
溯源树
样例
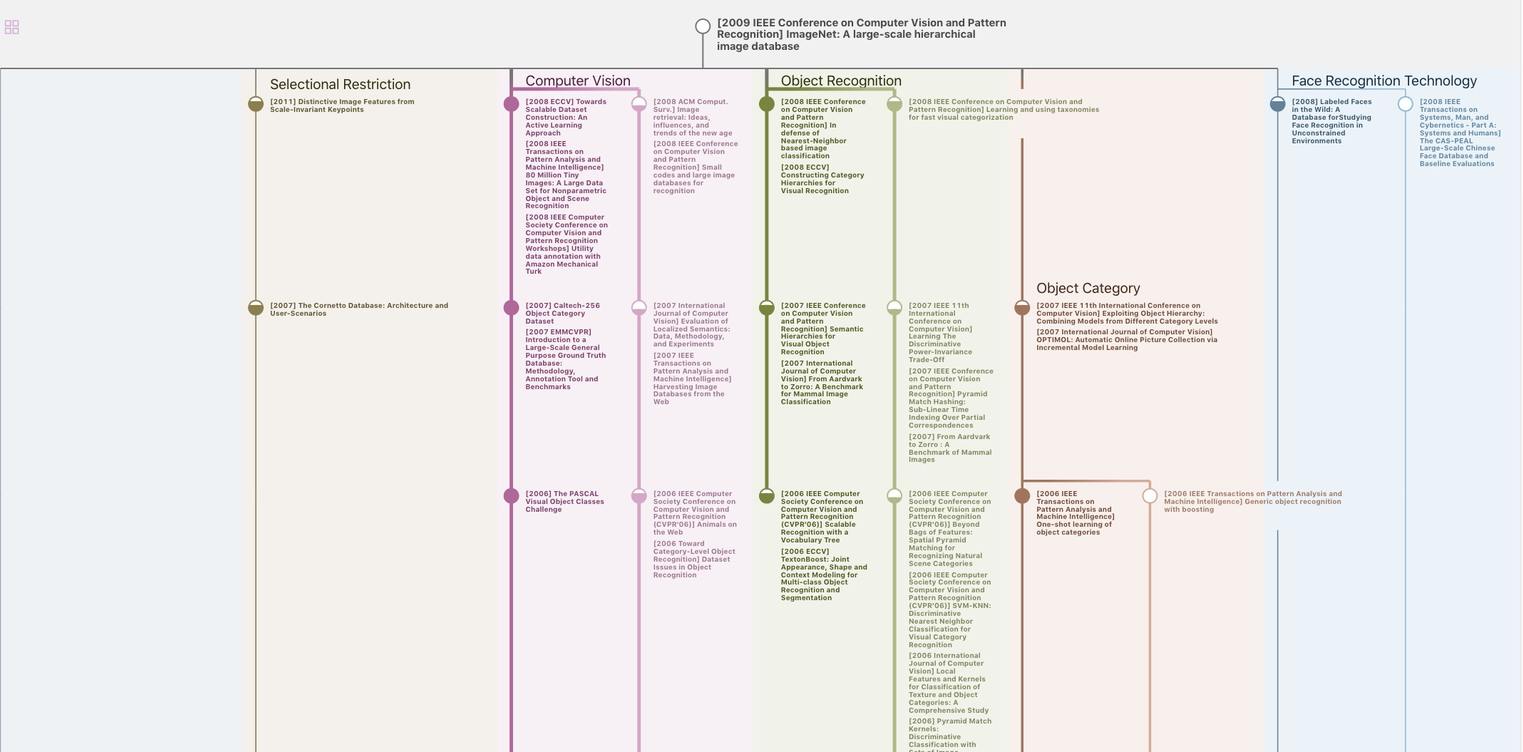
生成溯源树,研究论文发展脉络
Chat Paper
正在生成论文摘要