AIRCHITECT: Automating Hardware Architecture and Mapping Optimization
2023 DESIGN, AUTOMATION & TEST IN EUROPE CONFERENCE & EXHIBITION, DATE(2023)
摘要
Design space exploration and optimization is an essential but iterative step in custom accelerator design involving costly search based method to extract maximum performance and energy efficiency. State-of-the-art methods employ data centric approaches to reduce the cost of each iteration but still rely on search algorithms to obtain the optima. This work proposes a learned, constant time optimizer that uses a custom recommendation network called AIRCHITECT, which is capable of learning the architecture design and mapping space with a 94.3% test accuracy, and predicting optimal configurations, which achieve on an average (GeoMean) 99.9% of the best possible performance on a test dataset with 105 GEMM (GEneral Matrix-matrix Multiplication) workloads.
更多查看译文
关键词
machine learning,design space exploration,architecture design,mapping optimization
AI 理解论文
溯源树
样例
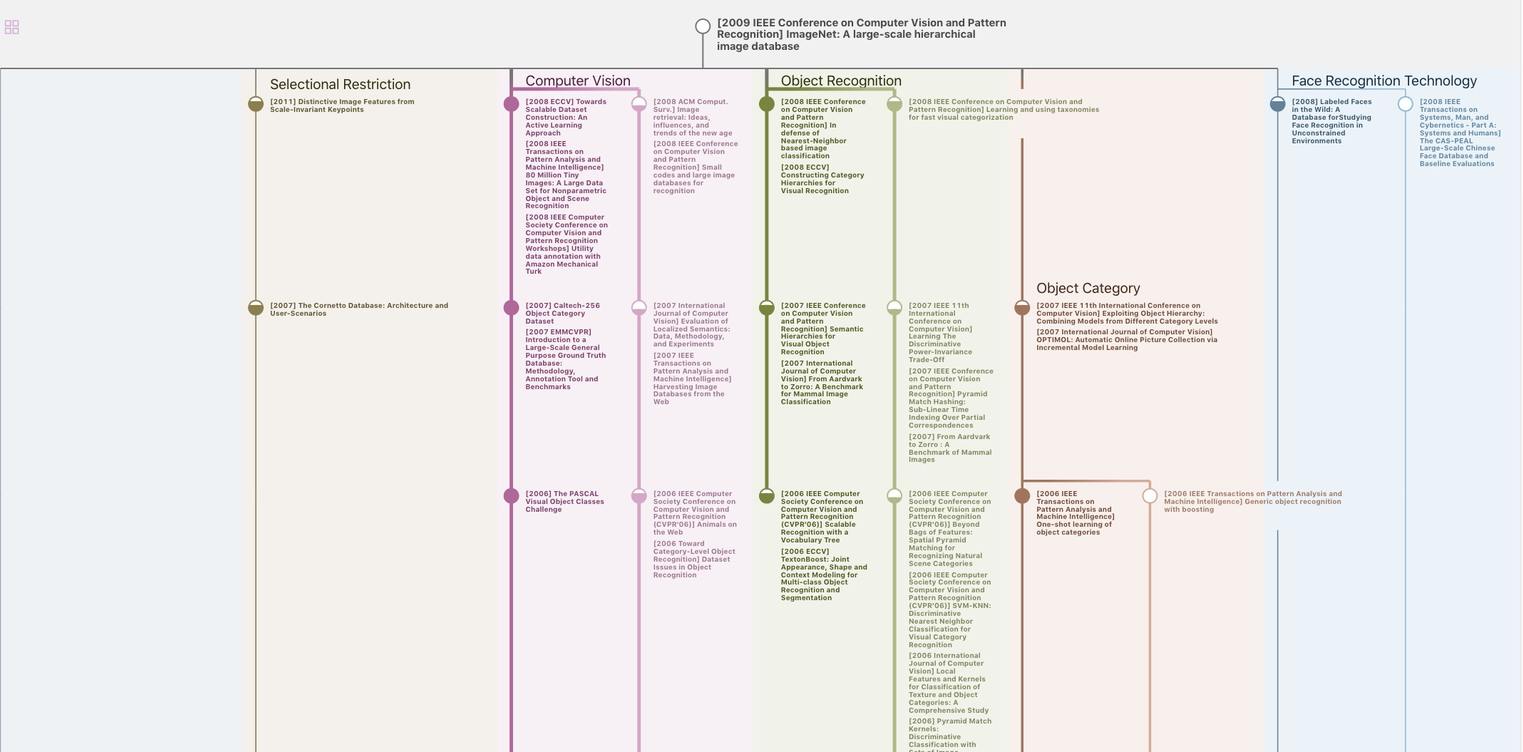
生成溯源树,研究论文发展脉络
Chat Paper
正在生成论文摘要