RankSearch: An Automatic Rank Search towards Optimal Tensor Compression for Video LSTM Networks on Edge
2023 DESIGN, AUTOMATION & TEST IN EUROPE CONFERENCE & EXHIBITION, DATE(2023)
摘要
Various industrial and domestic applications call for optimized lightweight video LSTM network models on edge. The recent tensor-train method can transform space-time features into tensors, which can be further decomposed into low-rank network models for lightweight video analysis on edge. The rank selection of tensor is however manually performed with no optimization. This paper formulates a rank search algorithm to automatically decide tensor ranks with consideration of the trade-off between network accuracy and complexity. A fast rank search method, called RankSearch, is developed to find optimized low-rank video LSTM network models on edge. Results from experiments show that RankSearch achieves a 4.84x reduction in model complexity, and 1.96x speed-up in run time while delivering a 3.86% accuracy improvement compared with the manual-ranked models.
更多查看译文
关键词
Tensor Rank Search,Complexity Aware Optimization,Tensorized Video LSTM,Edge Computing
AI 理解论文
溯源树
样例
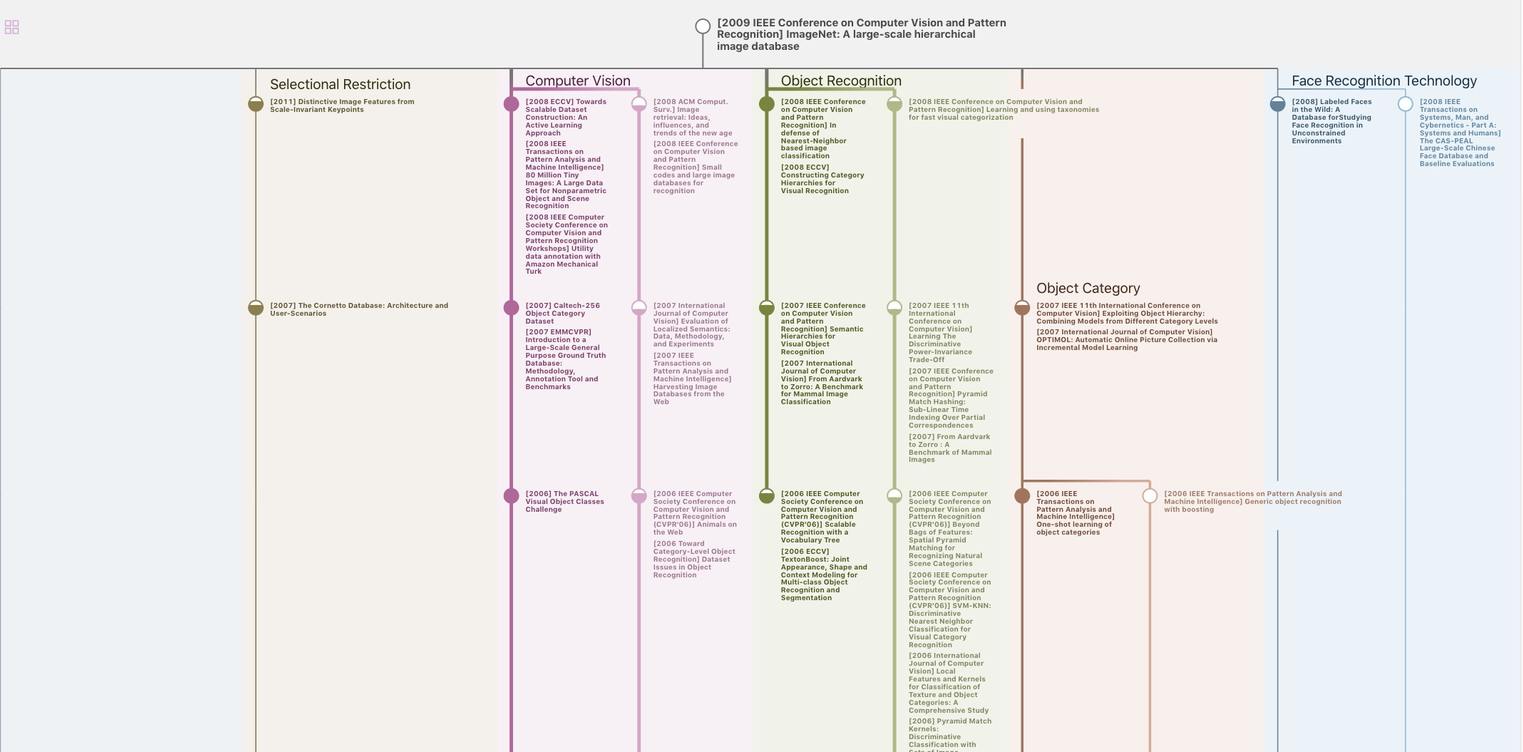
生成溯源树,研究论文发展脉络
Chat Paper
正在生成论文摘要