One-Class Models for Intrusion Detection at ISP Customer Networks.
AIAI (2)(2023)
摘要
Despite the explosion of IoT deployments at Internet Service Provider (ISP) customer networks, such devices remain vulnerable to cyber-attacks. We present a ML-based anomaly detection system, to be deployed at the Customer Premises Equipment (CPE), that leverages several One-Class Classification algorithms and majority voting to detect anomalous network traffic. We train these models using not only conventional per-flow features but also features extracted from sliding windows of flows. An extensive evaluation, using publicly available datasets shows that our algorithm has a higher detection rate than commonly supervised-learning algorithms, which require the use of labelled datasets. Our evaluation suggests that the detection capabilities of our algorithm are only marginally affected by Packet Acceleration, a technique used by CPEs to improve throughput but that reduces the number of packets (per flow) available to extract features from.
更多查看译文
关键词
IoT, Intrusion Detection System, One-Class Classification
AI 理解论文
溯源树
样例
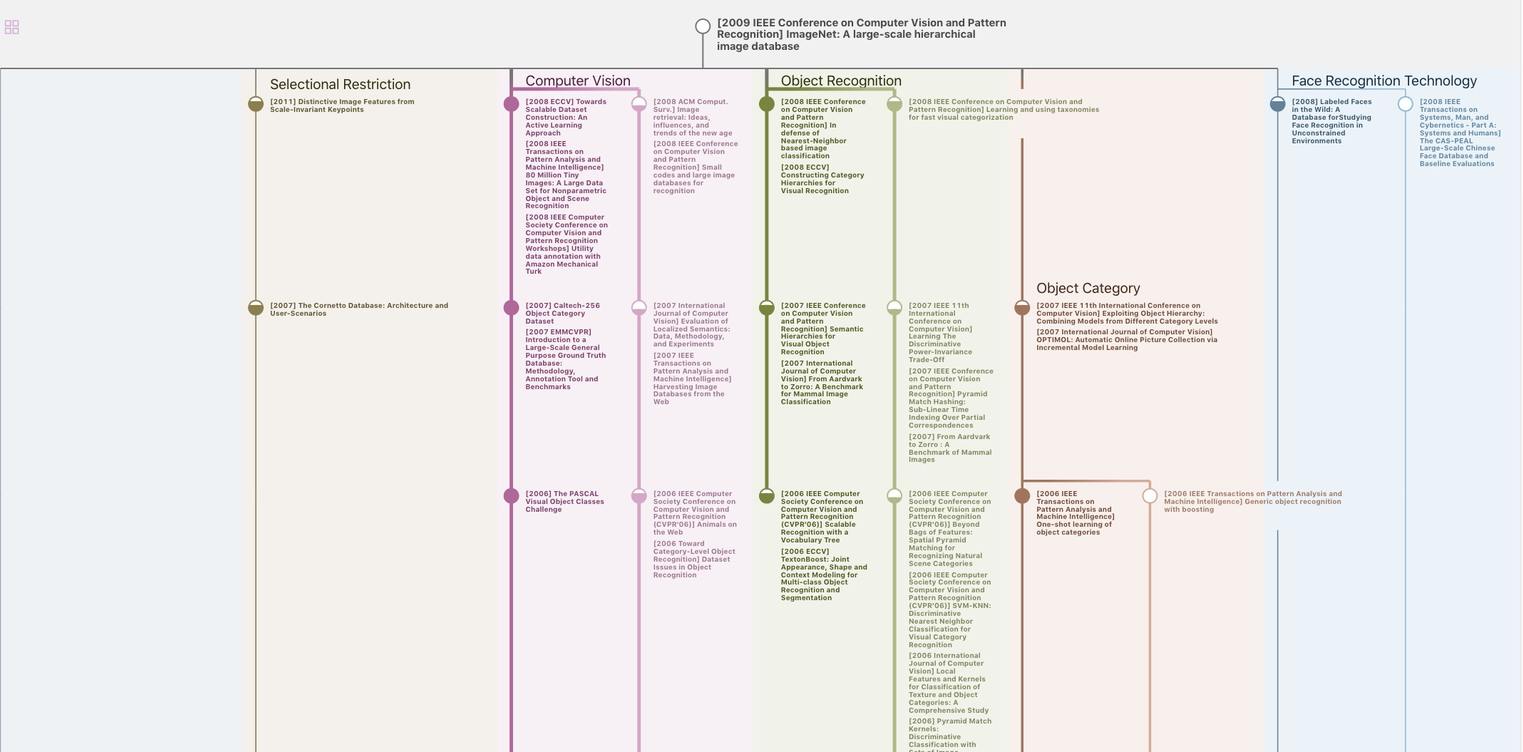
生成溯源树,研究论文发展脉络
Chat Paper
正在生成论文摘要