A Demonstration of Interpretability Methods for Graph Neural Networks
PROCEEDINGS OF THE 6TH ACM SIGMOD JOINT INTERNATIONAL WORKSHOP ON GRAPH DATA MANAGEMENT EXPERIENCES & SYSTEMS AND NETWORK DATA ANALYTICS, GRADES-NDA 2023(2023)
摘要
Graph neural networks (GNNs) are widely used in many downstream applications, such as graphs and nodes classification, entity resolution, link prediction, and question answering. Several interpretability methods for GNNs have been proposed recently. However, since they have not been thoroughly compared with each other, their trade-offs and efficiency in the context of underlying GNNs and downstream applications are unclear. To support more research in this domain, we develop an end-to-end interactive tool, named gInterpreter, by re-implementing 15 recent GNN interpretability methods in a common environment on top of a number of state-of-the-art GNNs employed for different downstream tasks. This paper demonstrates gInterpreter with an interactive performance profiling of 15 recent GNN interpretability methods, aiming to explain the complex deep learning pipelines over graph-structured data.
更多查看译文
关键词
Graph neural network,interpretability,explainable AI
AI 理解论文
溯源树
样例
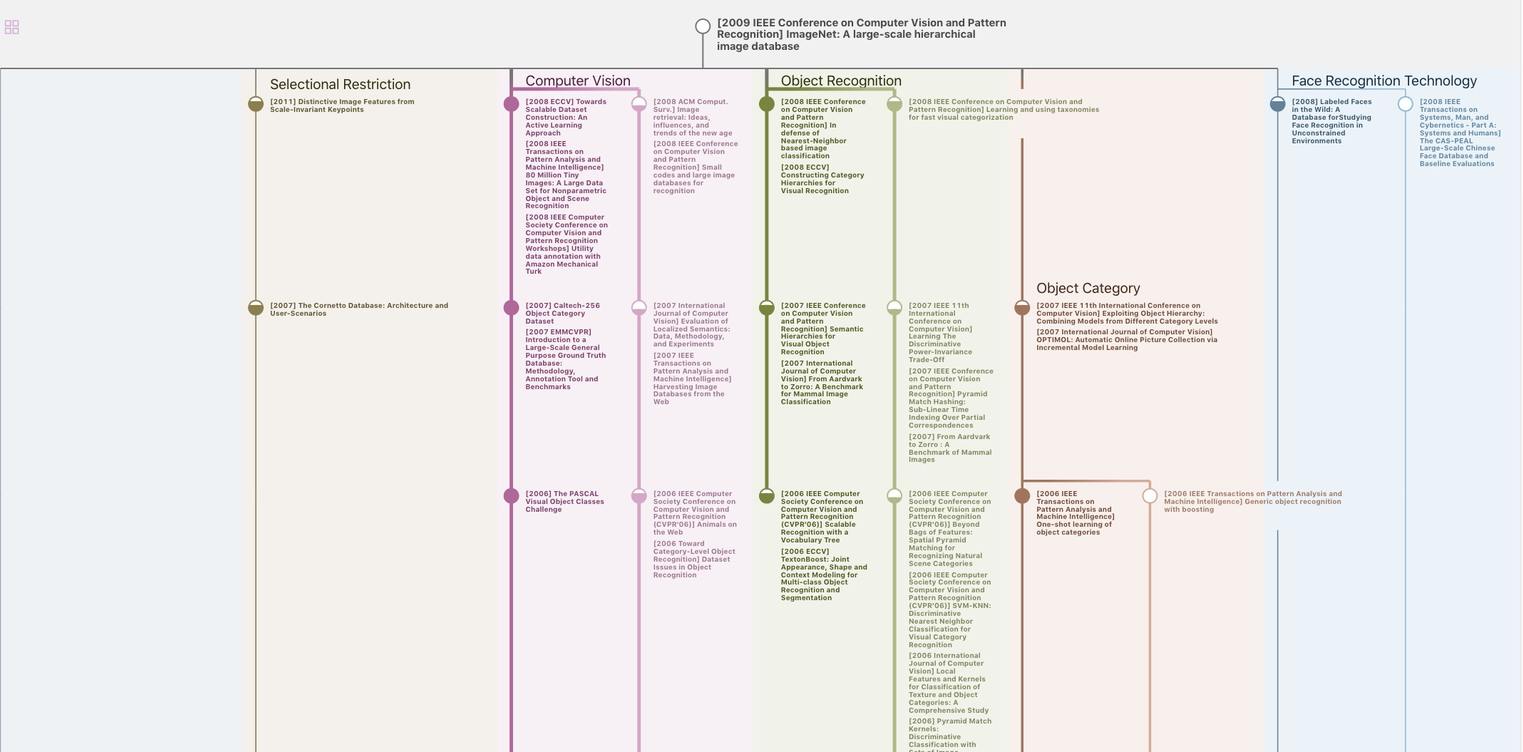
生成溯源树,研究论文发展脉络
Chat Paper
正在生成论文摘要