Deep Learning-Based Weight Initialization on Multi-layer Perceptron for Image Recognition.
DoSIER(2022)
摘要
The performance of the multi-layer perceptron (MLP) degrades while working with high-resolution images due to the issues of vanishing gradient and overfitting. However, the performance of an MLP can be improved with efficient weight initialization techniques. This paper puts forward a systematic deep neural network (DNN)-based weight initialization strategy for an MLP to enhance its classification accuracy. Moreover, the training of an MLP may not converge due to the presence of many local minima. It is feasible to avoid local minima by properly initializing weights as an alternative to random weight initialization. A restrictive Boltzmann machine (RBM) has been used in this paper to pre-train the MLP. An MLP is trained layer-by-layer, with weights between each neighboring layer up to the penultimate layer. The whole network is then fine-tuned after the pre-training, by the reduction of the mean square error (MSE). To compare the performance between the proposed initialization of weights and random weight initialization, two standard image classification data sets, (i) CIFER-10 and (ii) STL-10 are used. The outcomes of the simulation showcase the enhancement of the performance of MLP with the proposed weight initialization. Furthermore, the proposed method yields a better convergence speed than standard MLP.
更多查看译文
关键词
Deep neural network, Generalization, Multi-layer perceptron, Restricted Boltzmann machine
AI 理解论文
溯源树
样例
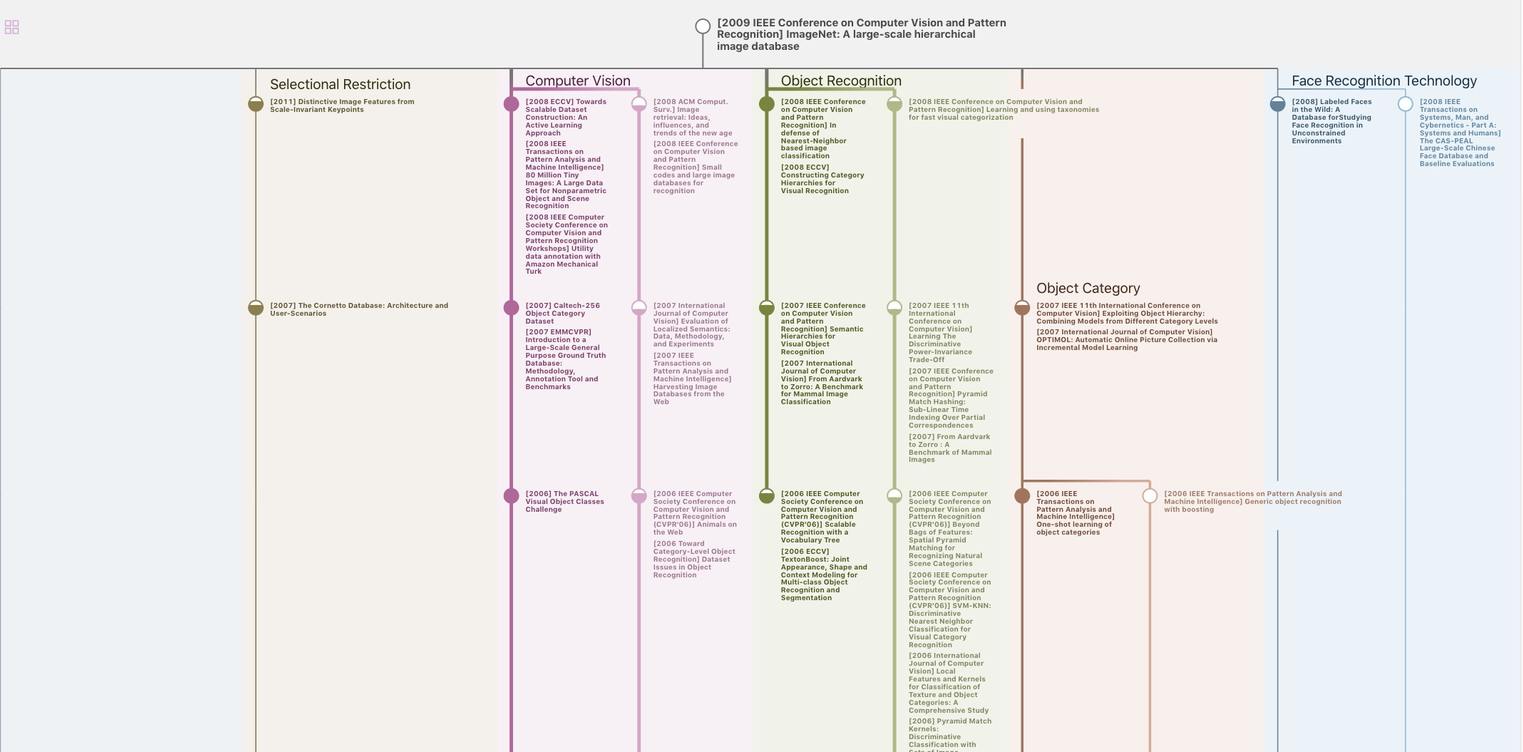
生成溯源树,研究论文发展脉络
Chat Paper
正在生成论文摘要