Generalizable Automatic Short Answer Scoring via Prototypical Neural Network.
AIED(2023)
摘要
We investigated the challenging task of generalizable automatic short answer scoring (ASAS), where a scoring model is tasked with generalizing to target domains (provided only with limited labeled data) that have no overlap with the auxiliary domains on which the model is trained. To address this, we introduced a framework based on Prototypical Neural Network (PNN). Specifically, for a target short answer instance whose score needs to be determined, the framework first calculates the distance between this target instance and each cluster of support instances (support instances are a set of labeled short answer instances that are grouped to different clusters according to their labels, i.e., the ground-truth scores). Then, it rates the target instance using the ground-truth score of the cluster that has the closest distance to the target instance. Through extensive empirical studies on an open-source ASAS dataset consisting of 10 different question prompts, we observed that the proposed approach consistently outperformed other baselines across settings concerning different numbers of support instances. We further observed that the proposed approach performed better when with wider training data sources than when with restricted data sources for training, showing that including more data sources for training may add to the generalizability of the proposed framework.
更多查看译文
关键词
prototypical neural network,short
AI 理解论文
溯源树
样例
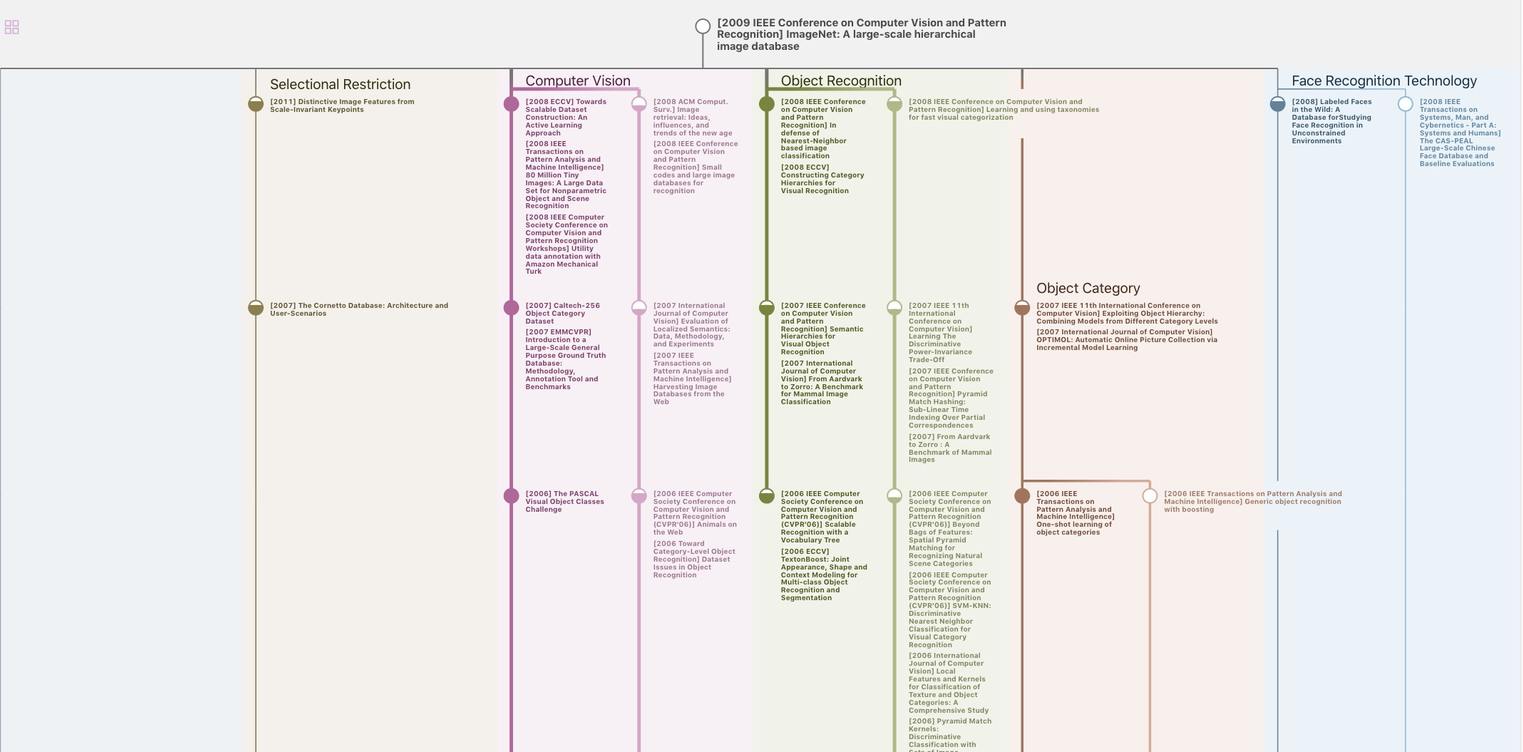
生成溯源树,研究论文发展脉络
Chat Paper
正在生成论文摘要