Understanding the Impact of Reinforcement Learning Personalization on Subgroups of Students in Math Tutoring
Artificial Intelligence in Education. Posters and Late Breaking Results, Workshops and Tutorials, Industry and Innovation Tracks, Practitioners, Doctoral Consortium and Blue Sky(2023)
摘要
Reinforcement learning has the promise to help reduce the cost of creating effective educational software through automatically adapting the experience to each individual. Most reinforcement learning algorithms aim to learn an automated pedagogical strategy that optimizes performance on average across the population and outputs a decision policy that may rely on complex representations, like deep neural networks, that are largely opaque. Yet, in most educational contexts, we would like a deeper understanding of educational interventions, such as if the machine-learned pedagogical strategy differs in its benefits to different students, how it differentiates instruction across individuals or situations, and if the personalized strategy learned has benefits over alternative personalizations or automated strategies. Here we explore such analyses for a reinforcement learning decision policy for educational software teaching students about the concept of volume. While some related work covers part of these analyses, we suggest that conducting all three such analyses can help enhance our understanding of the impact of a reinforcement learning decision policy in education and help inform stakeholders’ decisions around the use of a particular learned decision policy.
更多查看译文
关键词
reinforcement learning personalization,students,reinforcement learning
AI 理解论文
溯源树
样例
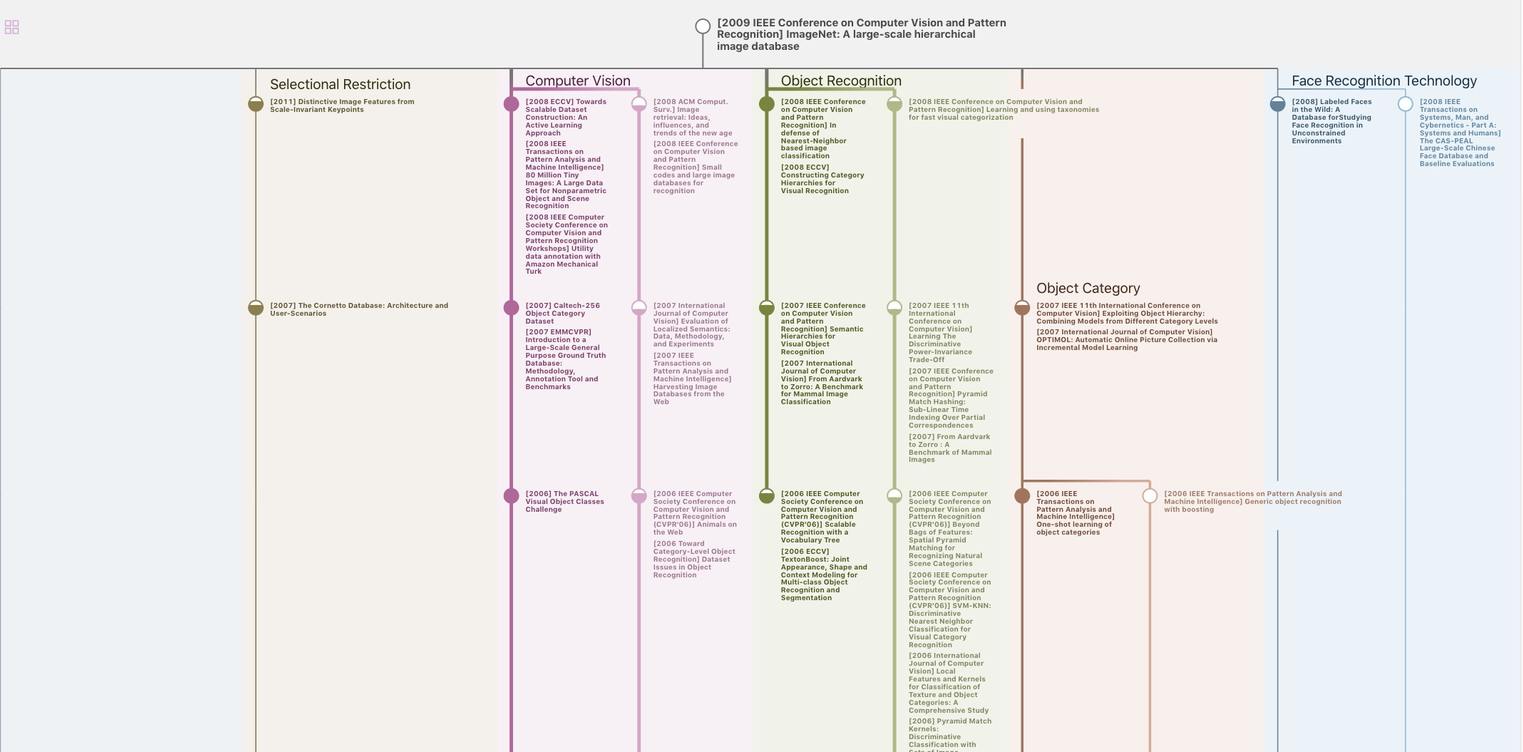
生成溯源树,研究论文发展脉络
Chat Paper
正在生成论文摘要