Predicting Papillary Renal Cell Carcinoma Prognosis Using Integrative Analysis of Histopathological Images and Genomic Data
Bioinformatics and Biomedical Engineering(2023)
摘要
Renal cell carcinoma (RCC) is a common malignant tumor of the adult kidney, with the papillary subtype (pRCC) as the second most frequent. There is a need to improve evaluative criteria for pRCC due to overlapping diagnostic characteristics in RCC subtypes. To create a better prognostic model for pRCC, we proposed an integration of morphologic and genomic features. Matched images and genomic data from The Cancer Genome Atlas were used. Image features were extracted using CellProfiler, and prognostic image features were selected using least absolute shrinkage and selection operator and support vector machine algorithms. Eigengene modules were identified using weighted gene co-expression network analysis. Risk groups based on prognostic features were significantly distinct (p < 0.05) according to Kaplan-Meier analysis and log-rank test results. We used two image features and nine eigengene modules to construct a model with the Random Survival Forest method, measuring 11-, 16-, and 20-month areas under the curve (AUC) of a time-dependent receiver operating curve. The integrative model (AUCs: 0.877, 0.769, and 0.811) outperformed models trained with eigengenes alone (AUCs: 0.75, 0.733, and 0.785) and morphological features alone (AUCs: 0.593, 0.523, 0.603). This suggests that an integrative prognostic model based on histopathological images and genomic features could significantly improve survival prediction for pRCC patients and assist in clinical decision-making.
更多查看译文
关键词
renal cell carcinoma,histopathological images
AI 理解论文
溯源树
样例
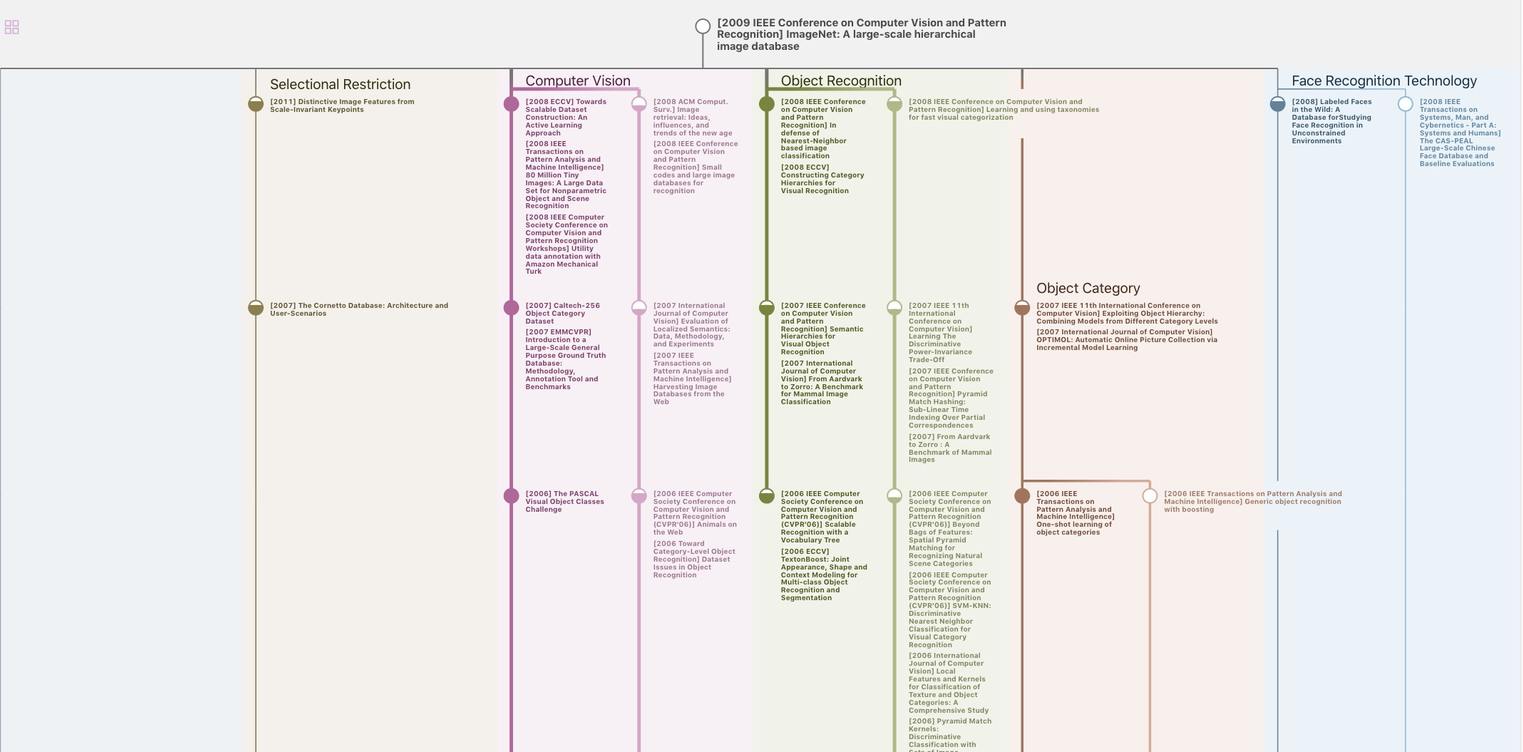
生成溯源树,研究论文发展脉络
Chat Paper
正在生成论文摘要