Virtual Arm with Multimodal Biased Feedback for Improving EEG Motor Imagery Calibration Training
IRC-SET 2022(2023)
摘要
The brain-computer interface (BCI) records electrical signals in the brain. BCI-generated data could be used to develop devices that help people with motor disabilities to move. For this to be done, we must build machine learning (ML) models that are capable of translating brain signals into motor activity. However, it is challenging to build such ML models with current-day BCI data, which are based on motor imagery designs. Herein, we assess the possibility of training ML models based on an alternative form of BCI data that is generated when subjects observe motor movements, as opposed to mere imagery. We demonstrated that this method of data collection improves training accuracy and the ML model’s performance. We further improved its performance by providing multimodal biased feedback. Our work provides the foundation for further development of BCI-based ML models.
更多查看译文
关键词
BCI, ML, EEG
AI 理解论文
溯源树
样例
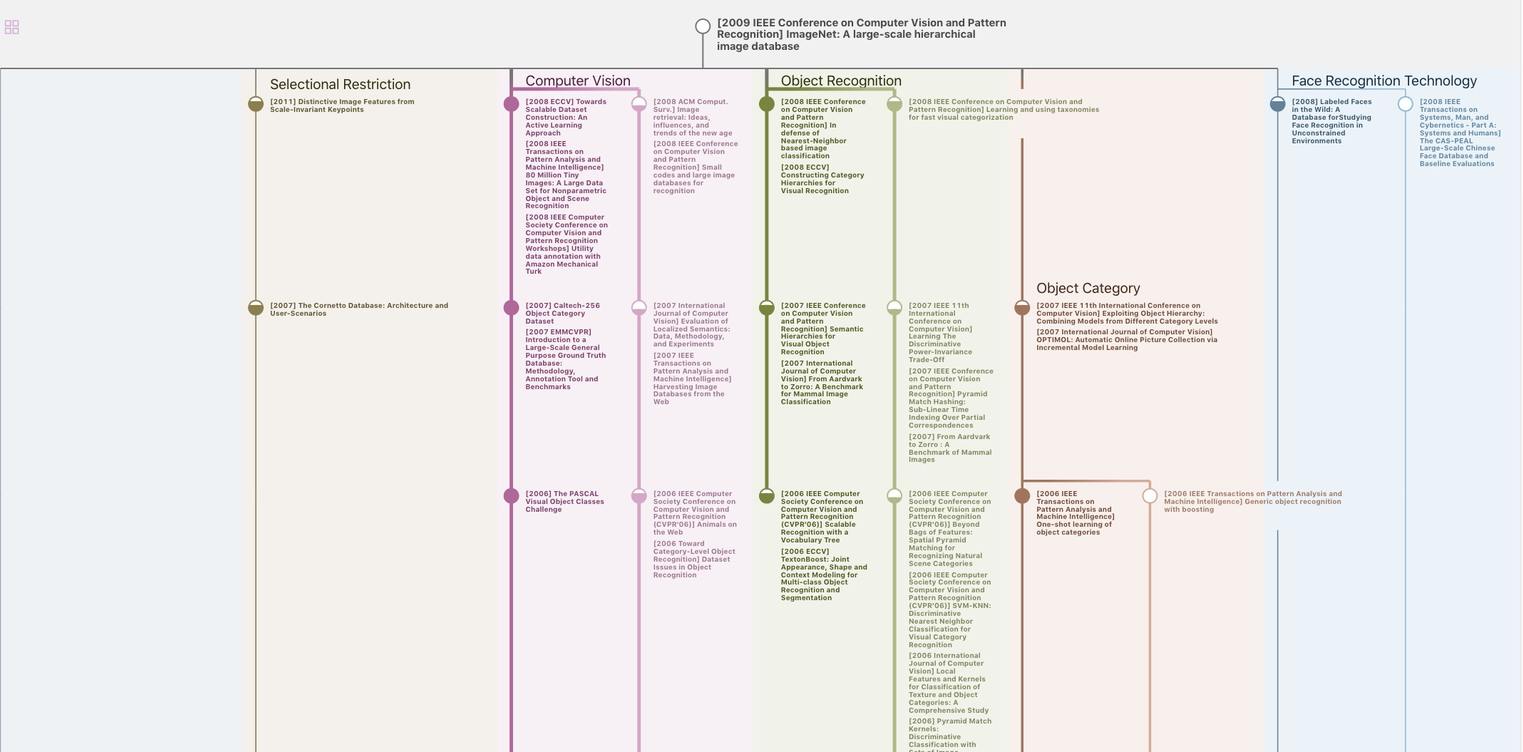
生成溯源树,研究论文发展脉络
Chat Paper
正在生成论文摘要