A Data-Centric Approach for Reducing Carbon Emissions in Deep Learning
Advanced Information Systems Engineering(2023)
摘要
The growing popularity of Deep Learning (DL) in recent years has had a large environmental impact. Training models require a lot of processing and computation and therefore require a lot of energy. The size of these models and the amount of data required for training them have grown exponentially, not comparable to the performance improvements. Recently, some model-centric approaches have been proposed to limit the environmental impact of AI. This paper complements them by proposing a data-centric “Green AI” approach, focusing on the data preparation phase of the DL pipeline. A general methodology, valid for any DL task, is proposed. This methodology is based on analyzing data characteristics, mainly the data quality and volume dimensions, and observing how these affect carbon emissions and performance on different models. With this information, a human-in-the-loop (HITL) approach is provided to support researchers in obtaining a modified and reduced version of a dataset that can decrease the environmental impact of training while achieving a specified performance goal. To demonstrate its validity, the proposed methodology is applied to the time series classification task and a prototype has been developed which demonstrates the possibility of reducing the carbon emissions of DL training by up to 50%.
更多查看译文
关键词
carbon emissions,deep learning,data-centric
AI 理解论文
溯源树
样例
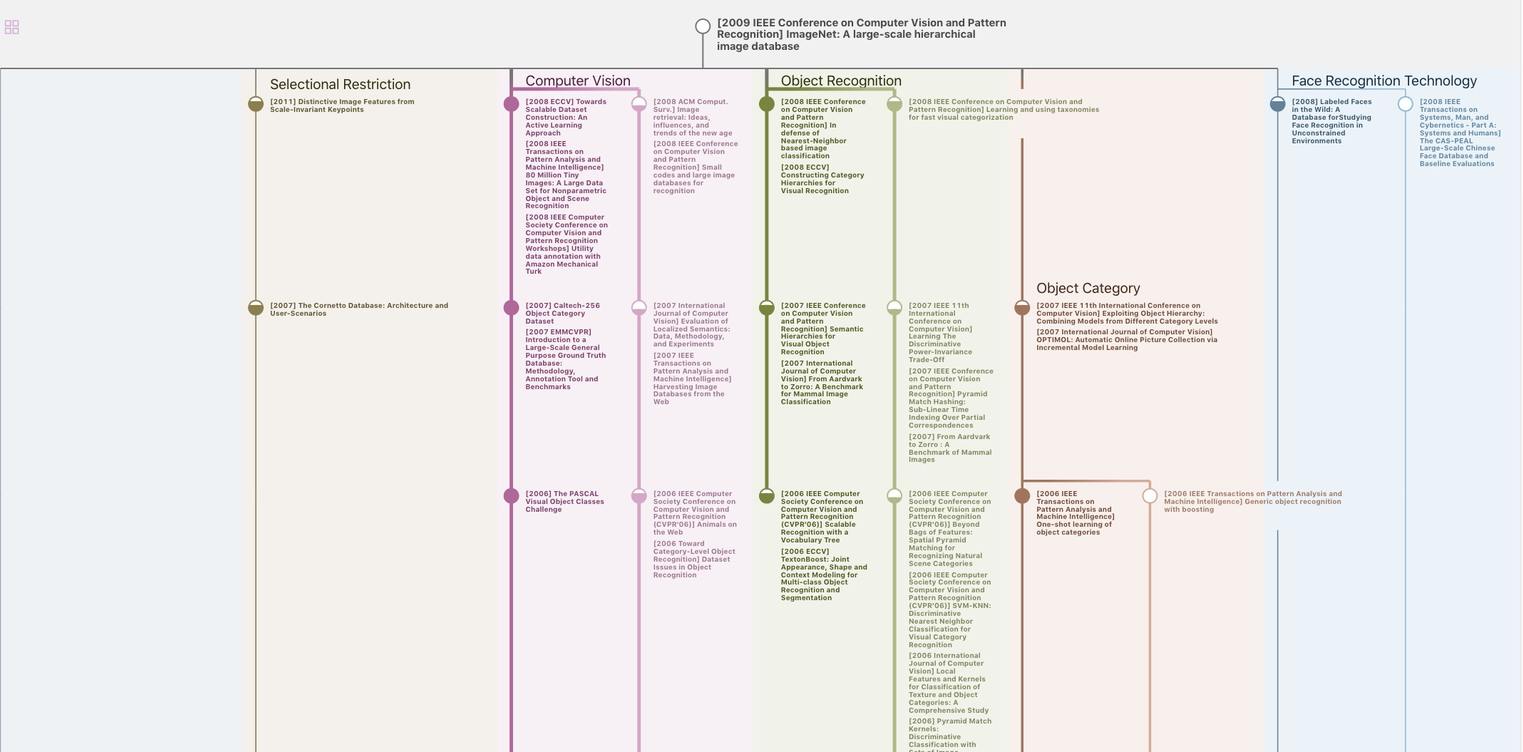
生成溯源树,研究论文发展脉络
Chat Paper
正在生成论文摘要