Can You Solve This on the First Try? – Understanding Exercise Field Performance in an Intelligent Tutoring System
Artificial Intelligence in Education(2023)
摘要
The analysis of fine-grained data on students’ learning behavior in intelligent tutoring systems using machine learning is a starting point for personalized learning. However, findings from such analyses are commonly viewed as uninterpretable and, hence, not helpful for understanding learning processes. The explainable AI method SHAP, which generates detailed explanations, is a promising approach here. It can help to better understand how different learning behaviors influence students’ learning outcomes and potentially produce new insights for adaptable intelligent tutoring systems in the future. Based on K-12 data (N = 472 students), we trained several ML models on student characteristics and behavioral trace data to predict whether a student will answer an exercise field correctly or not—a low-level approximation of academic performance. The optimized machine learning models (lasso regression, random forest, XGBoost) performed well (
$$AUC = 0.68$$
;
$$F_1 = [0.63; 0.66; 0.69]$$
), outperforming logistic regression (
$$AUC = 0.57$$
;
$$F_1 = 0.52$$
). SHAP importance values for the best model (XGBoost) indicated that, besides prior language achievement, several behavioral variables (e.g., performance on the previous attempts) were informative predictors. Thus, specific learning behaviors can help explain exercise field performance, independent of the student’s basic abilities and demographics—providing insights into areas of potential intervention. Moreover, the SHAP values highlight heterogeneity in the effects, supporting the notion of personalized learning.
更多查看译文
关键词
exercise field performance,understanding
AI 理解论文
溯源树
样例
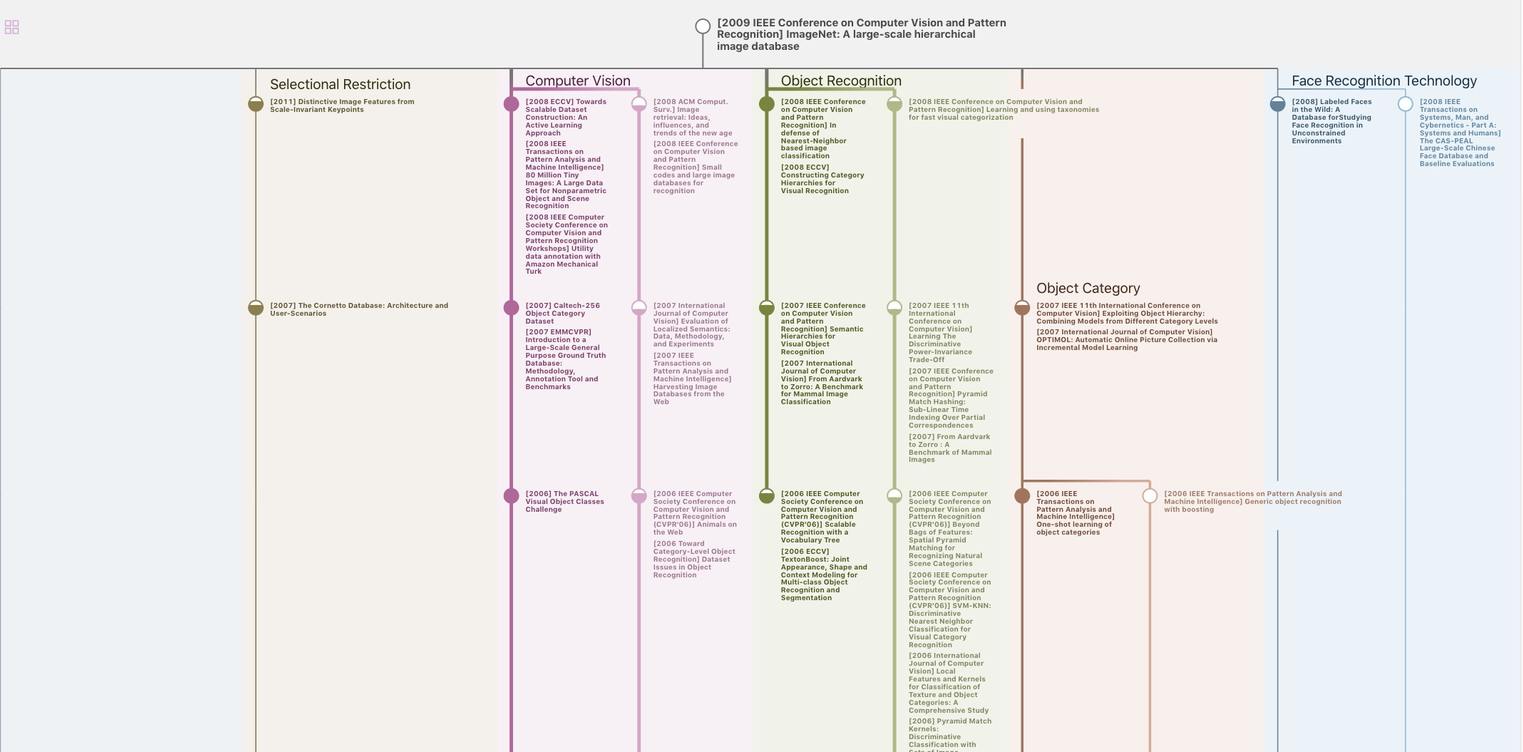
生成溯源树,研究论文发展脉络
Chat Paper
正在生成论文摘要