Evaluation of Calibration Paradigms to Improve Wearable EEG-Based Attention Recognition
IRC-SET 2022(2023)
摘要
Attention is a complex process that is important in achieving optimal task performance. EEG-based attention recognition involves calibration tasks that subjects need to perform, as well as training of the attention model for reliable detection of attention levels. However, these calibration paradigms might not be ideal for inducing desirable attention states, as they are simple and monotonous. There is also limited evaluation of the different calibration tasks to assess their attention detection performance. To fill up this gap, we designed different calibration tasks and compared their effectiveness in inducing attention. We collected EEG data from 29 subjects using a consumer-grade EEG headband according to our experimental protocol. We used six bandpower features per channel to classify binary attention states (attention VS inattention). Based on our evaluation results, we discovered that two modified calibration tasks (Modified Flanker and Colour Flanker) achieved higher classification accuracies compared to the baseline task (Baseline Flanker). The Modified Flanker task achieved the highest mean subject-independent accuracy of 66.45 ± 16.38% across subjects. Our findings showed that the different subjects need unique calibration paradigms to achieve a high attention classification performance. In comparison with qualitative survey data analysis, we also found that the subjects’ personality and learner types do not correlate highly with attention detection performance.
更多查看译文
关键词
EEG, Attention, Calibration tasks
AI 理解论文
溯源树
样例
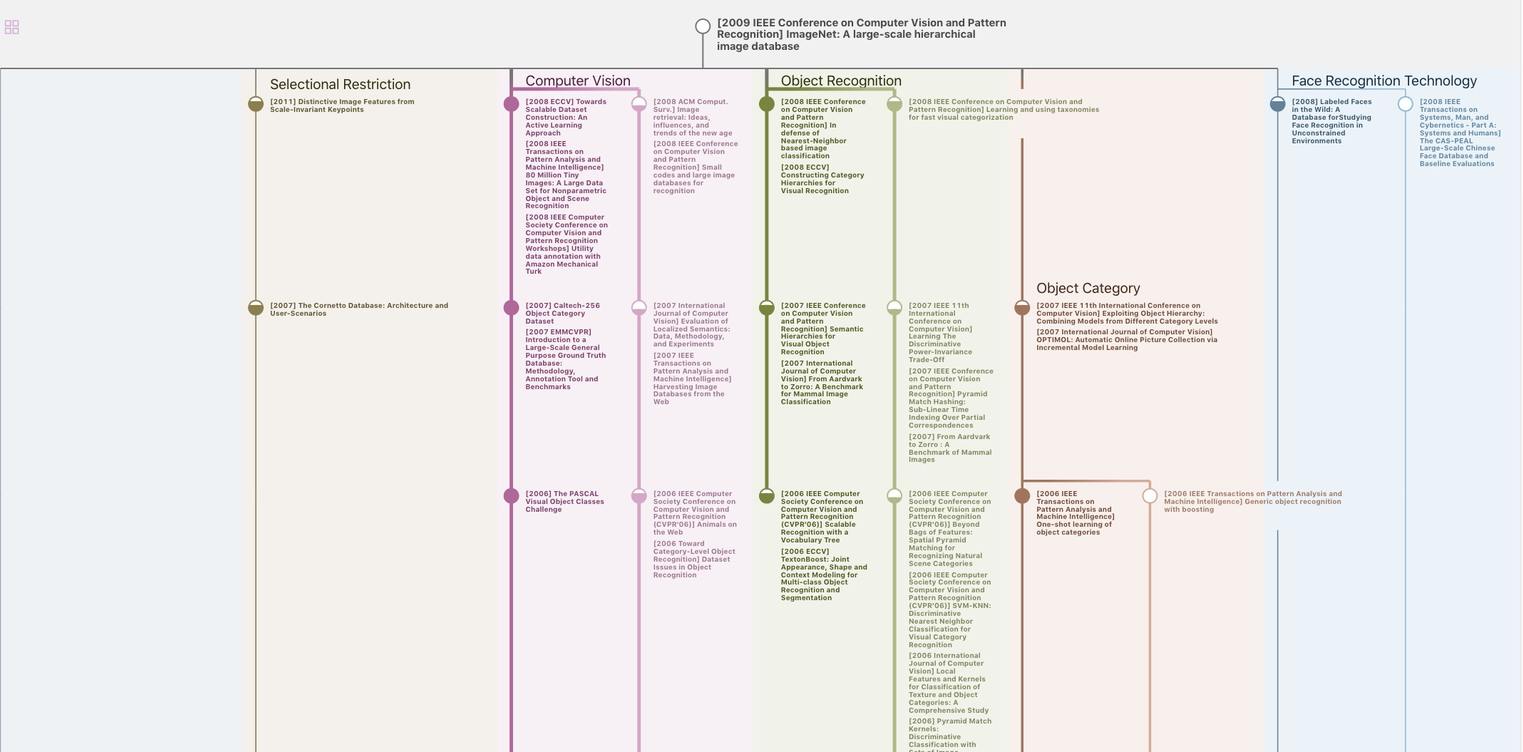
生成溯源树,研究论文发展脉络
Chat Paper
正在生成论文摘要