Act3D: Infinite Resolution Action Detection Transformer for Robotic Manipulation
CoRR(2023)
摘要
3D perceptual representations are well suited for robot manipulation as they easily encode occlusions and simplify spatial reasoning. Many manipulation tasks require high spatial precision in end-effector pose prediction, typically demanding high-resolution 3D perceptual grids that are computationally expensive to process. As a result, most manipulation policies operate directly in 2D, foregoing 3D inductive biases. In this paper, we propose Act3D, a manipulation policy Transformer that casts 6-DoF keypose prediction as 3D detection with adaptive spatial computation. It takes as input 3D feature clouds unprojected from one or more camera views, iteratively samples 3D point grids in free space in a coarse-to-fine manner, featurizes them using relative spatial attention to the physical feature cloud, and selects the best feature point for end-effector pose prediction. Act3D sets a new state-of-the-art in RLbench, an established manipulation benchmark. Our model achieves 10% absolute improvement over the previous SOTA 2D multi-view policy on 74 RLbench tasks and 22% absolute improvement with 3x less compute over the previous SOTA 3D policy. In thorough ablations, we show the importance of relative spatial attention, large-scale vision-language pre-trained 2D backbones, and weight tying across coarse-to-fine attentions. Code and videos are available at our project site: https://act3d.github.io/.
更多查看译文
AI 理解论文
溯源树
样例
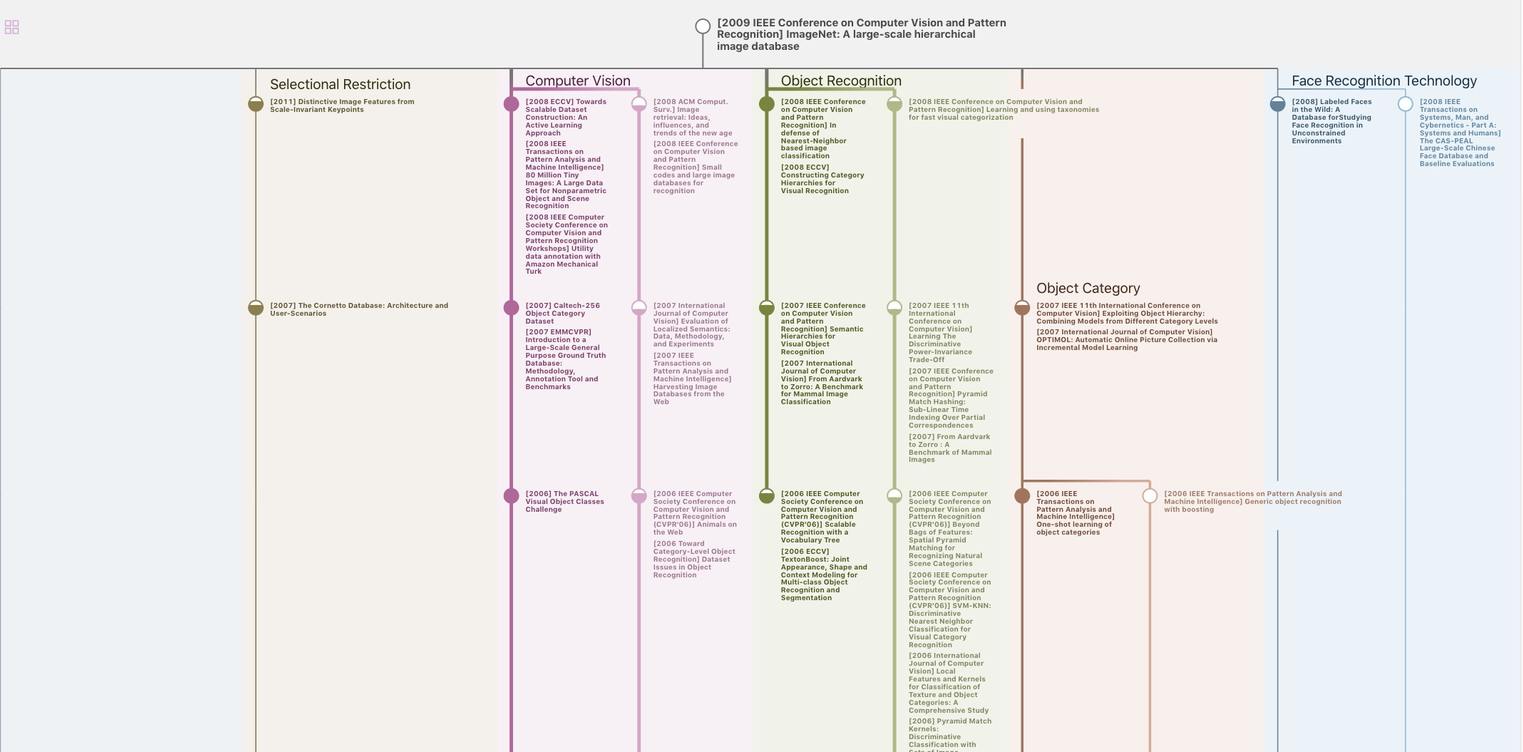
生成溯源树,研究论文发展脉络
Chat Paper
正在生成论文摘要