Practical and Asymptotically Exact Conditional Sampling in Diffusion Models
NeurIPS(2023)
摘要
Diffusion models have been successful on a range of conditional generation tasks including molecular design and text-to-image generation. However, these achievements have primarily depended on task-specific conditional training or error-prone heuristic approximations. Ideally, a conditional generation method should provide exact samples for a broad range of conditional distributions without requiring task-specific training. To this end, we introduce the Twisted Diffusion Sampler, or TDS. TDS is a sequential Monte Carlo (SMC) algorithm that targets the conditional distributions of diffusion models. The main idea is to use twisting, an SMC technique that enjoys good computational efficiency, to incorporate heuristic approximations without compromising asymptotic exactness. We first find in simulation and on MNIST image inpainting and class-conditional generation tasks that TDS provides a computational statistical trade-off, yielding more accurate approximations with many particles but with empirical improvements over heuristics with as few as two particles. We then turn to motif-scaffolding, a core task in protein design, using a TDS extension to Riemannian diffusion models. On benchmark test cases, TDS allows flexible conditioning criteria and often outperforms the state of the art.
更多查看译文
关键词
asymptotically exact conditional sampling,diffusion
AI 理解论文
溯源树
样例
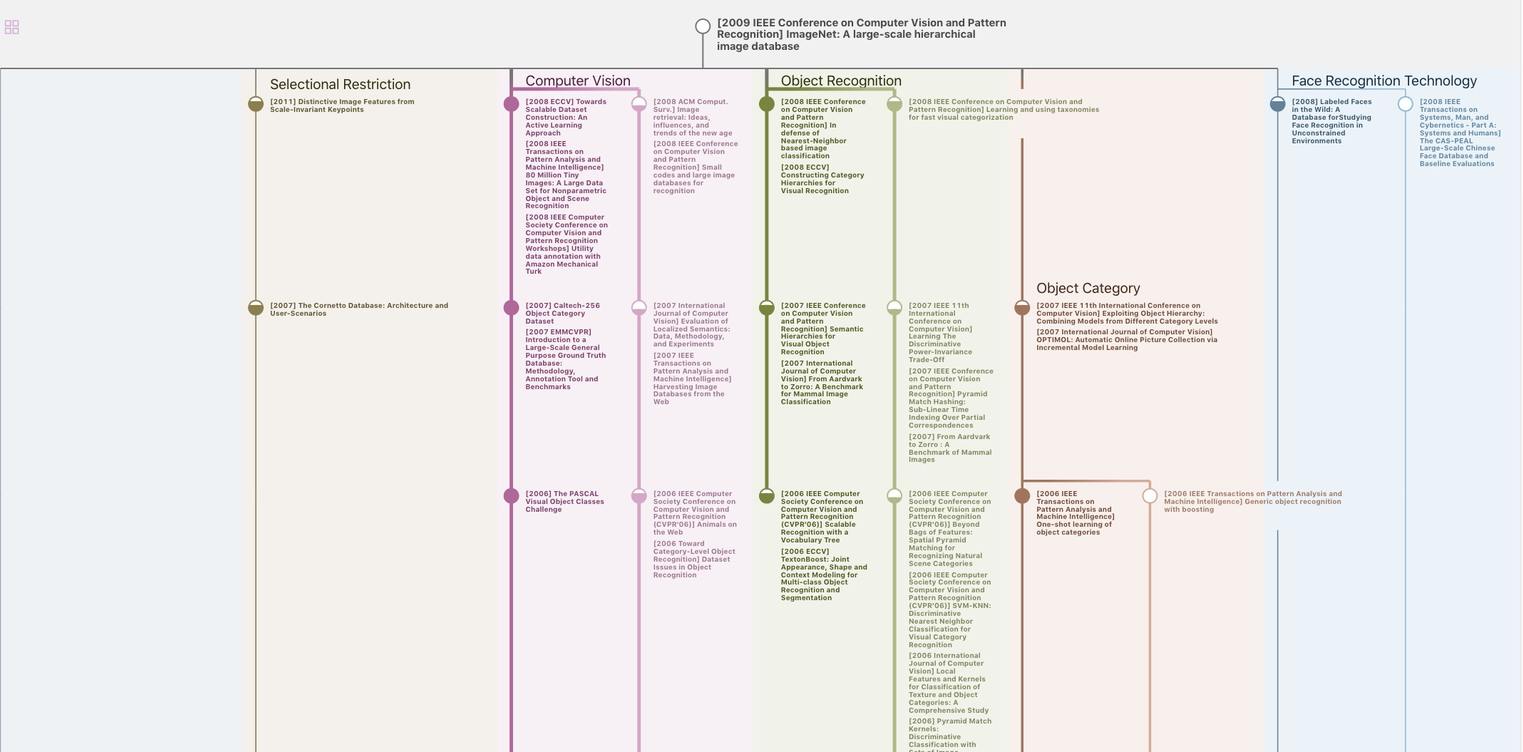
生成溯源树,研究论文发展脉络
Chat Paper
正在生成论文摘要