RBSR: Efficient and Flexible Recurrent Network for Burst Super-Resolution
PATTERN RECOGNITION AND COMPUTER VISION, PRCV 2023, PT VI(2024)
摘要
Burst super-resolution (BurstSR) aims at reconstructing a high-resolution (HR) image from a sequence of low-resolution (LR) and noisy images, which is conducive to enhancing the imaging effects of smartphones with limited sensors. The main challenge of BurstSR is to effectively combine the complementary information from input frames, while existing methods still struggle with it. In this paper, we suggest fusing cues frame-by-frame with an efficient and flexible recurrent network. In particular, we emphasize the role of the base-frame and utilize it as a key prompt to guide the knowledge acquisition from other frames in every recurrence. Moreover, we introduce an implicit weighting loss to improve the model's flexibility in facing input frames with variable numbers. Extensive experiments on both synthetic and real-world datasets demonstrate that our method achieves better results than state-of-the-art ones. Codes and pre-trained models are available at https://github. com/ZcsrenlongZ/RBSR.
更多查看译文
关键词
Burst Super-Resolution,Recurrent Network,Super-Resolution
AI 理解论文
溯源树
样例
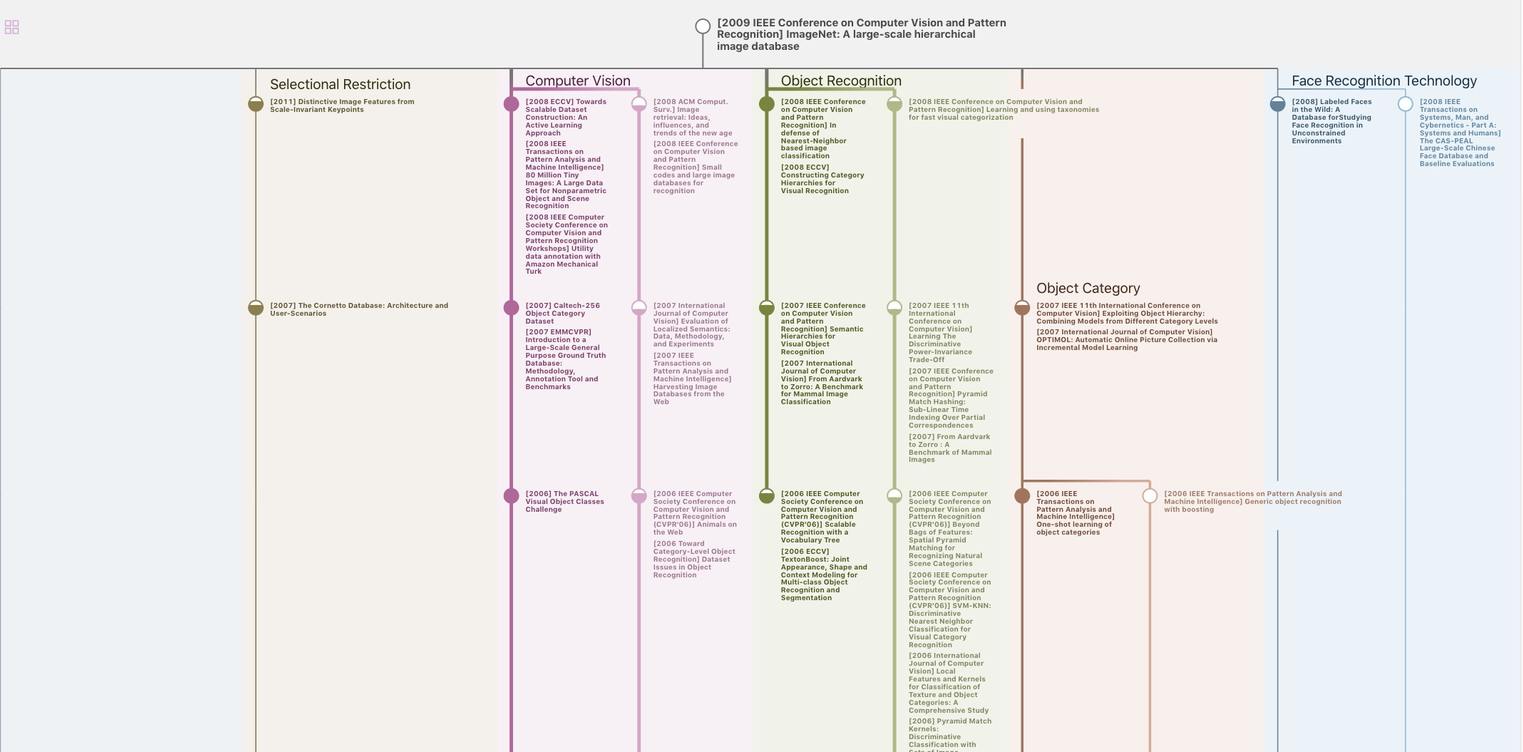
生成溯源树,研究论文发展脉络
Chat Paper
正在生成论文摘要