Designing stable neural networks using convex analysis and ODEs
Physica D: Nonlinear Phenomena(2024)
摘要
Motivated by classical work on the numerical integration of ordinary differential equations we present a ResNet-styled neural network architecture that encodes non-expansive (1-Lipschitz) operators, as long as the spectral norms of the weights are appropriately constrained. This is to be contrasted with the ordinary ResNet architecture which, even if the spectral norms of the weights are constrained, has a Lipschitz constant that, in the worst case, grows exponentially with the depth of the network. Further analysis of the proposed architecture shows that the spectral norms of the weights can be further constrained to ensure that the network is an averaged operator, making it a natural candidate for a learned denoiser in Plug-and-Play algorithms. Using a novel adaptive way of enforcing the spectral norm constraints, we show that, even with these constraints, it is possible to train performant networks. The proposed architecture is applied to the problem of adversarially robust image classification, to image denoising, and finally to the inverse problem of deblurring.
更多查看译文
关键词
Deep learning,Numerical integration of ODEs,Convex analysis,Monotone operator theory,Inverse problems
AI 理解论文
溯源树
样例
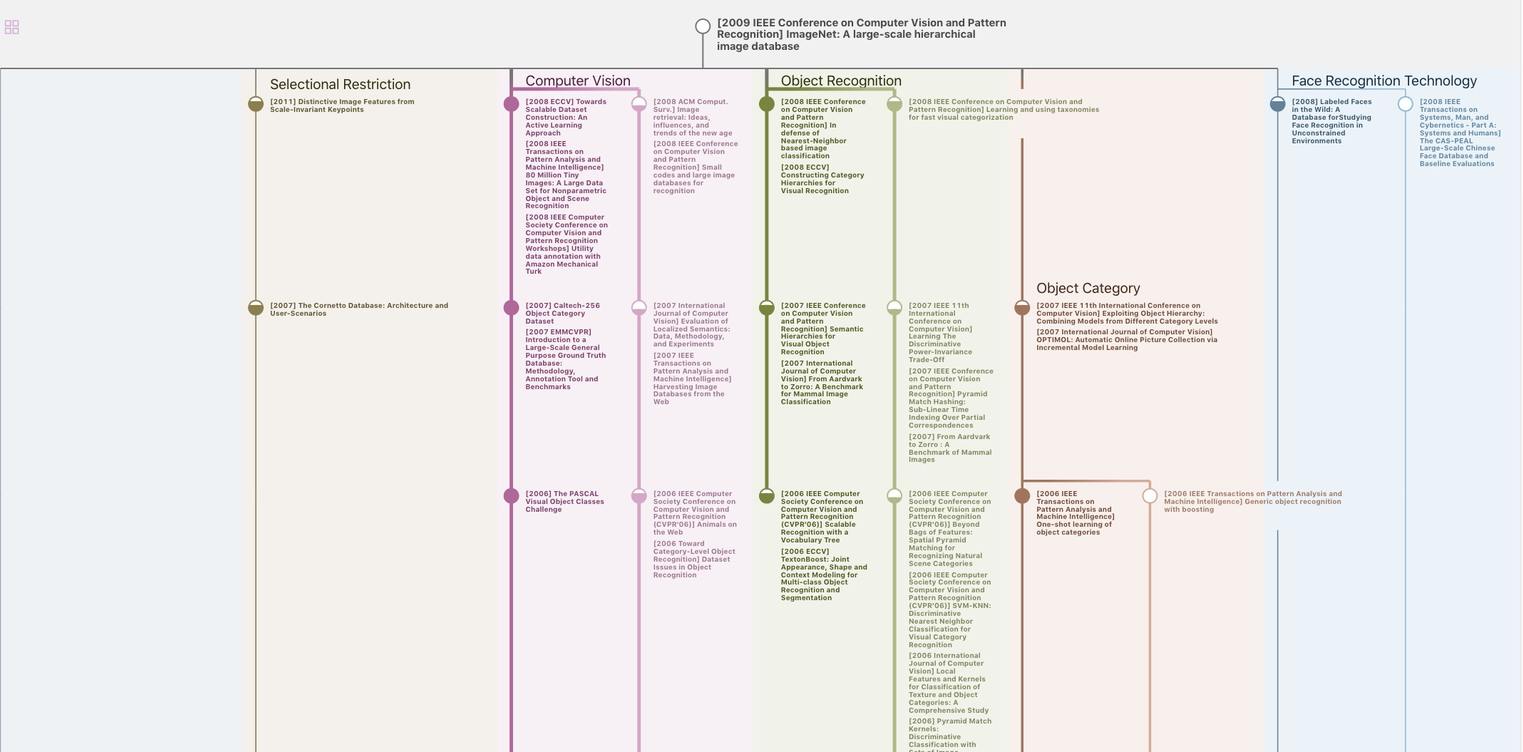
生成溯源树,研究论文发展脉络
Chat Paper
正在生成论文摘要