AI-enabled Automatic Multimodal Fusion of Cone-Beam CT and Intraoral Scans for Intelligent 3D Tooth-Bone Reconstruction and Clinical Applications
crossref(2022)
摘要
Abstract Cone-beam computed tomography (CBCT) is one of the most widely used digital models in dental practices. A critical step in virtual dental treatment planning is to accurately delineate all tooth-bone structures from CBCT with high fidelity. Previous studies have established several methods for CBCT segmentation using deep learning. However, the inherent resolution discrepancy and the loss of occlusal information in CBCT largely limited its clinical applicability. Here, we present a Deep Dental Multimodal Analysis (DDMA) framework consisting of a CBCT segmentation model, an intraoral scan (IOS) segmentation model (the most accurate digital dental model), and a fusion model to generate 3D fused tooth-bone structures with high fidelity and accurate occlusal information. Our model was trained with a large-scale dataset with 503 CBCT and 28,559 IOS meshes manually annotated by human experts. For CBCT segmentation, our framework achieves an average Dice coefficient of 93.99%, significantly outperforming the baselines. For IOS segmentation, our model achieves a mIoU of 93.07% and 95.70% on the maxillary and mandible on a test dataset of 200 IOS meshes, 1.77% and 3.52% higher than the state-of-art method. In addition, our DDMA framework takes 20 to 25 minutes to generate the fused model, compared to over 5 hours by human experts. Notably, real-world clinical cases demonstrate that our model can visualize tooth-bone structures and relationships during the entire orthodontic treatment and predict clinical risks like dehiscence and fenestration. These findings demonstrate the potential of deep multimodal learning to improve the quality of digital dental models and guide clinical treatment and diagnostic decisions.
更多查看译文
AI 理解论文
溯源树
样例
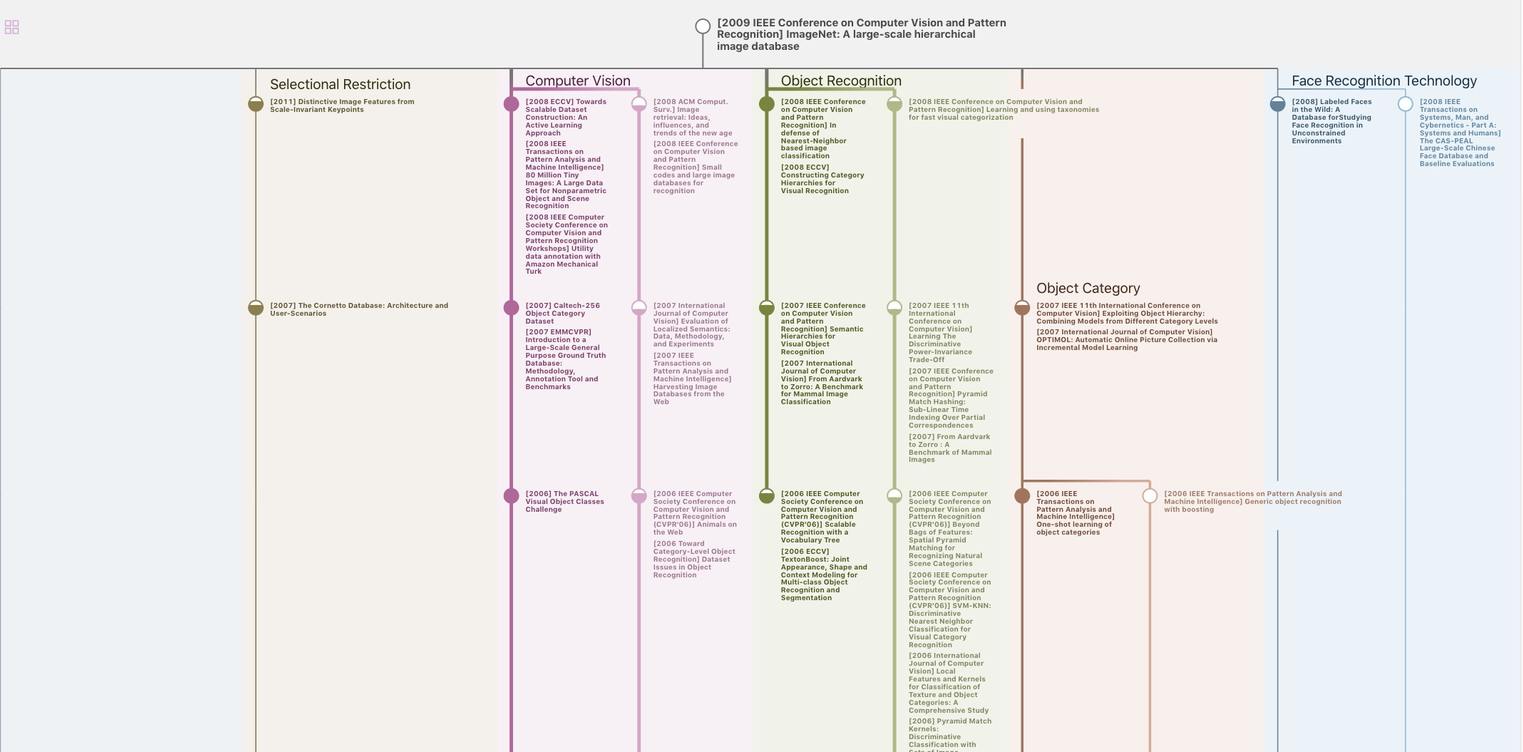
生成溯源树,研究论文发展脉络
Chat Paper
正在生成论文摘要