Enhanced prediction of short-term prognosis of community-acquired pneumonia using random survival forests by incorporating suppression of tumorigenicity 2 as a feature
Research Square (Research Square)(2022)
摘要
Abstract Purpose: The development of biomarkers and models can screen inpatients with a low probability of cure in the early stages of admission to help doctors adjust the management of community-acquired pneumonia (CAP) patients.Methods: We conducted a 30-day cohort study of newly admitted adult CAP patients over 20 years of age. Prognosis models to predict the short-term prognosis were developed using random survival forest (RSF) method.Results: A total of 247 adult CAP patients were studied and 208 (84.21%) of them reached clinical stability within 30 days. The soluble form of suppression of tumorigenicity-2 (sST2) was an independent predictor of clinical stability and the addition of sST2 to the prognosis model could improve the performance of the prognosis model. The C-index of the RSF model to predict clinical stability was 0.8342 (95%CI, 0.8086-0.8598), which is higher than 0.7181 (95%CI, 0.6933-0.7429) of CURB 65 score, 0.8025 (95%CI, 0.7776-8274) of PSI score and 0.8214(95% CI, 0.8080-0.8348) of cox regression. In addition, the RSF model was associated with adverse clinical events during hospitalization, ICU admissions and short-term mortality.Conclusion: The RSF model by incorporating sST2 was more accurate than traditional methods in assessing the short-term prognosis of CAP patients.
更多查看译文
关键词
pneumonia,random survival forests,prognosis,prediction,short-term,community-acquired
AI 理解论文
溯源树
样例
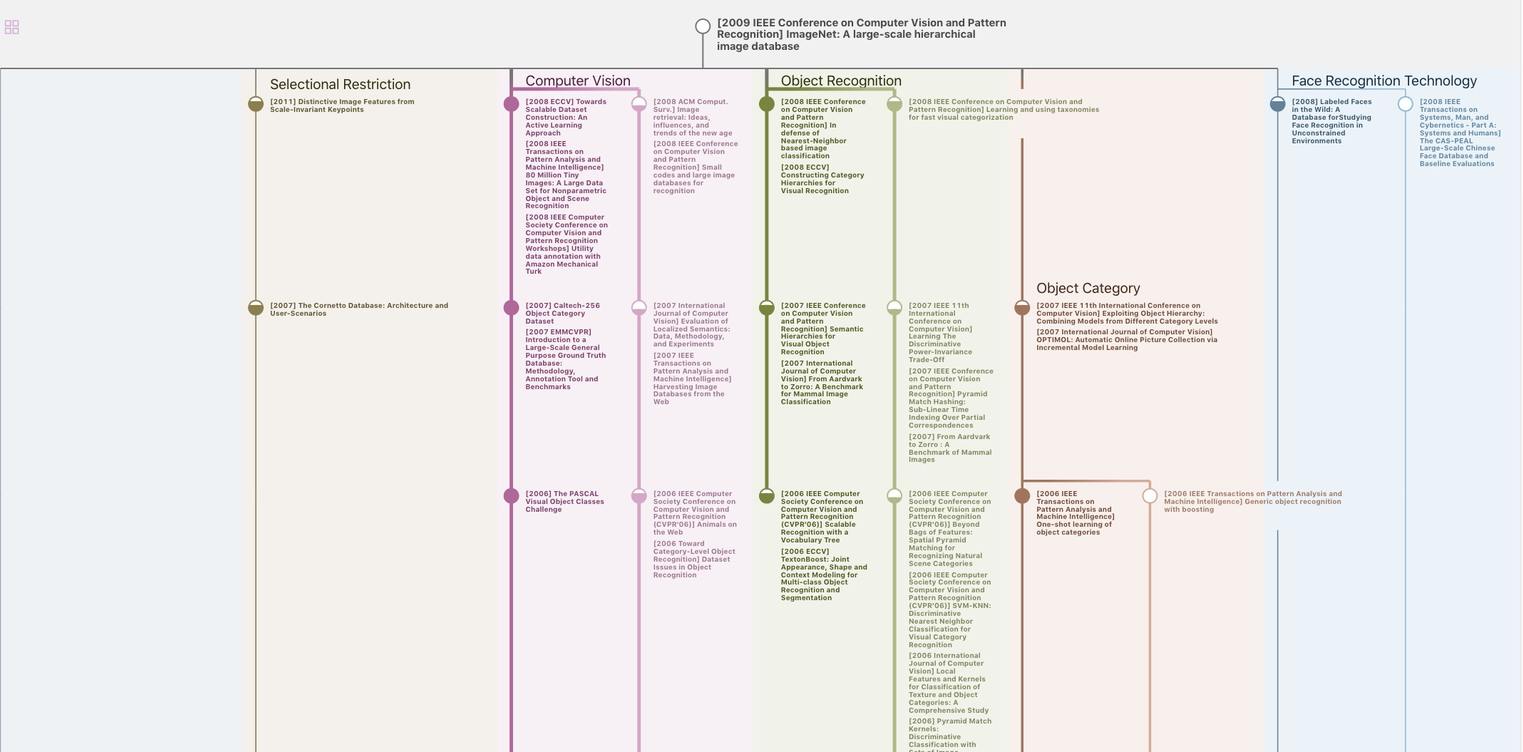
生成溯源树,研究论文发展脉络
Chat Paper
正在生成论文摘要