Spatial parameter optimization of a terrestrial biosphere model for improving estimation of carbon fluxes for deciduous forests in the eastern United States: an efficient model-data fusion method
crossref(2022)
摘要
Abstract. Inaccurate parameter estimation is a significant source of uncertainty in complex terrestrial biosphere models. Model parameters may have large spatial variability, even within a vegetation type. Model uncertainty from parameter uncertainty can be significantly reduced by model-data fusion (MDF), which, however, is difficult to implement over a large region with traditional methods due to the high computational cost. This study proposed a hybrid modeling approach that couples a terrestrial biosphere model with a data-driven machine learning method, which uses satellite information and considers the physical mechanisms. We developed a two-step framework to estimate the essential parameters of the revised Integrated Biosphere Simulator (IBIS) pixel by pixel using the satellite-derived leaf area index (LAI) and gross primary productivity (GPP) products as “true values.” The first step was to estimate the optimal parameters for each sample using a modified adaptive surrogate modeling algorithm (MASM). We applied the Gaussian Process Regression algorithm (GPR) as a surrogate model to learn the relationship between model parameters and errors. In our second step, we built an eXtreme Gradient Boosting (XGBoost) model between the optimized parameters and local environmental variables. The trained XGBoost model was then used to predict optimal parameters spatially across the deciduous forests in the eastern United States. The results showed that the parameters were highly variable spatially and quite different from the default values over forests, and the simulation errors of the GPP and LAI could be markedly reduced with the optimized parameters. The effectiveness of the optimized model in estimating GPP, ecosystem respiration (ER) and net ecosystem exchange (NEE) were also tested through site validation. The optimized model reduced the root-mean-squared-error (RMSE) from 7.03 to 6.22 gC m-2 8 d-1 for GPP, 2.65 to 2.11 gC m-2 8 d-1 for ER and 4.45 to 4.38 gC m-2 8 d-1 for NEE. The mean annual GPP, ER and NEE of the region from 2000 to 2019 were 5.79, 4.60 and -1.19 Pg year-1, respectively. The strategy used in this study requires only a few hundred model runs to calibrate regional parameters and is readily applicable to other complex terrestrial biosphere models with different spatial resolutions. Our study also emphasizes the necessity of pixel-level parameter calibration and the value of remote sensing products for per-pixel parameter optimization.
更多查看译文
AI 理解论文
溯源树
样例
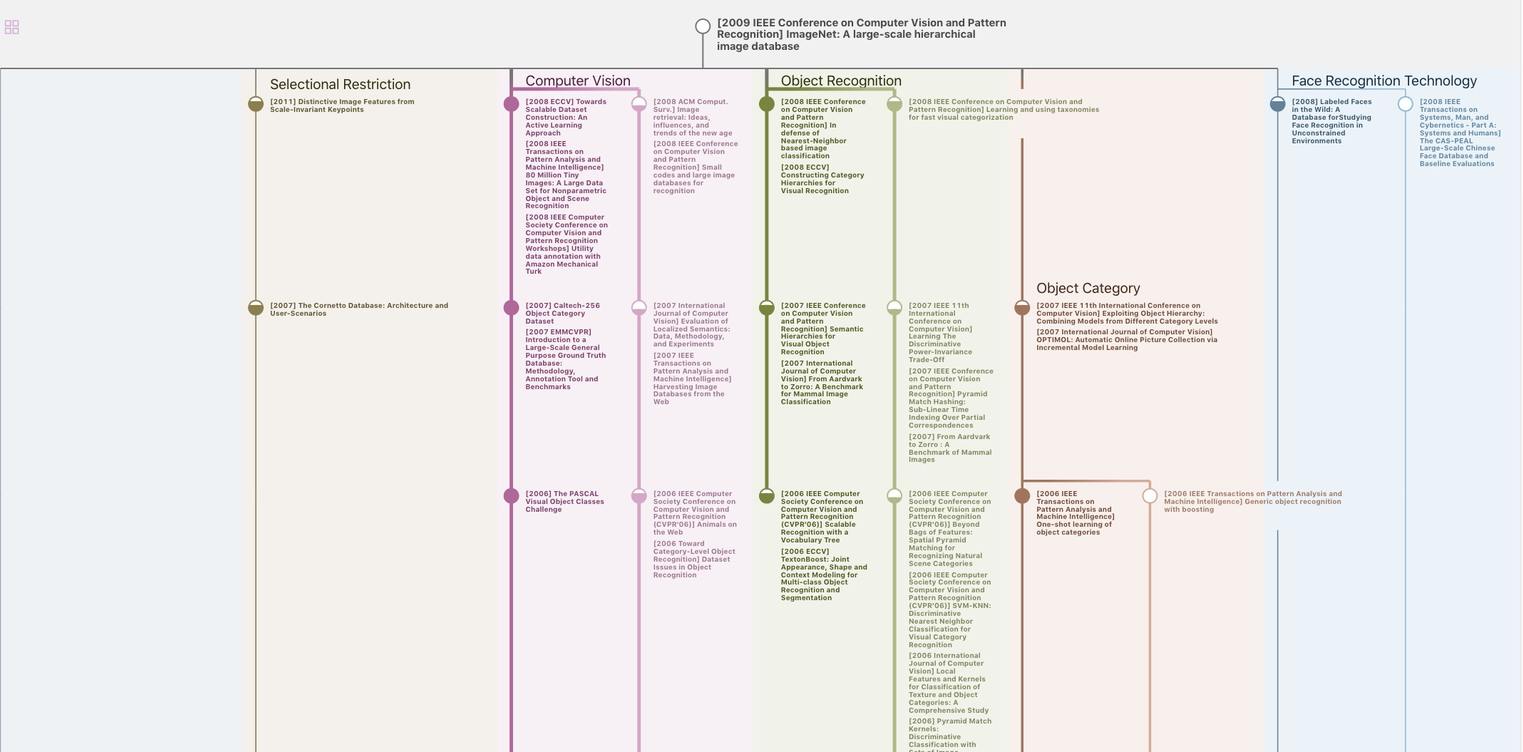
生成溯源树,研究论文发展脉络
Chat Paper
正在生成论文摘要