FRC-Net: A Simple Yet Effective Architecture for Low-Light Image Enhancement
IEEE Transactions on Consumer Electronics(2023)
摘要
Low-light image enhancement (LLIE) aims at refining illumination and restoring the details of low-light images. However, current deep LLIE models still face two crucial issues causing blurred textures and inaccurate illumination: 1) low-quality detail-recovery results due to information loss; 2) complex and even redundant model structure. In this paper, we therefore propose a simple yet effective deep LLIE architecture, termed Full-Resolution Context Network (FRC-Net). To avoid the visual information loss caused by feature scaling, we present a novel full-resolution representation strategy to replace all feature scaling operations, which can prevent the information degradation by making the intermediary features keep the original resolution. The structure of FRC-Net is very simple, which only contains 12 cascaded layers: 7 convolutional layers and 5 newly-designed context attention (CA) modules. The plug-and-play CA module is designed to overcome the limited receptive field caused by shallow structures by learning global context as well as retaining local details. Extensive experiments show that our model obtains better detail-recovery quality over current SOTA methods, with relatively fewer parameters and faster inference speed.
更多查看译文
关键词
Low-light image enhancement,simple yet effective,full resolution,context attention
AI 理解论文
溯源树
样例
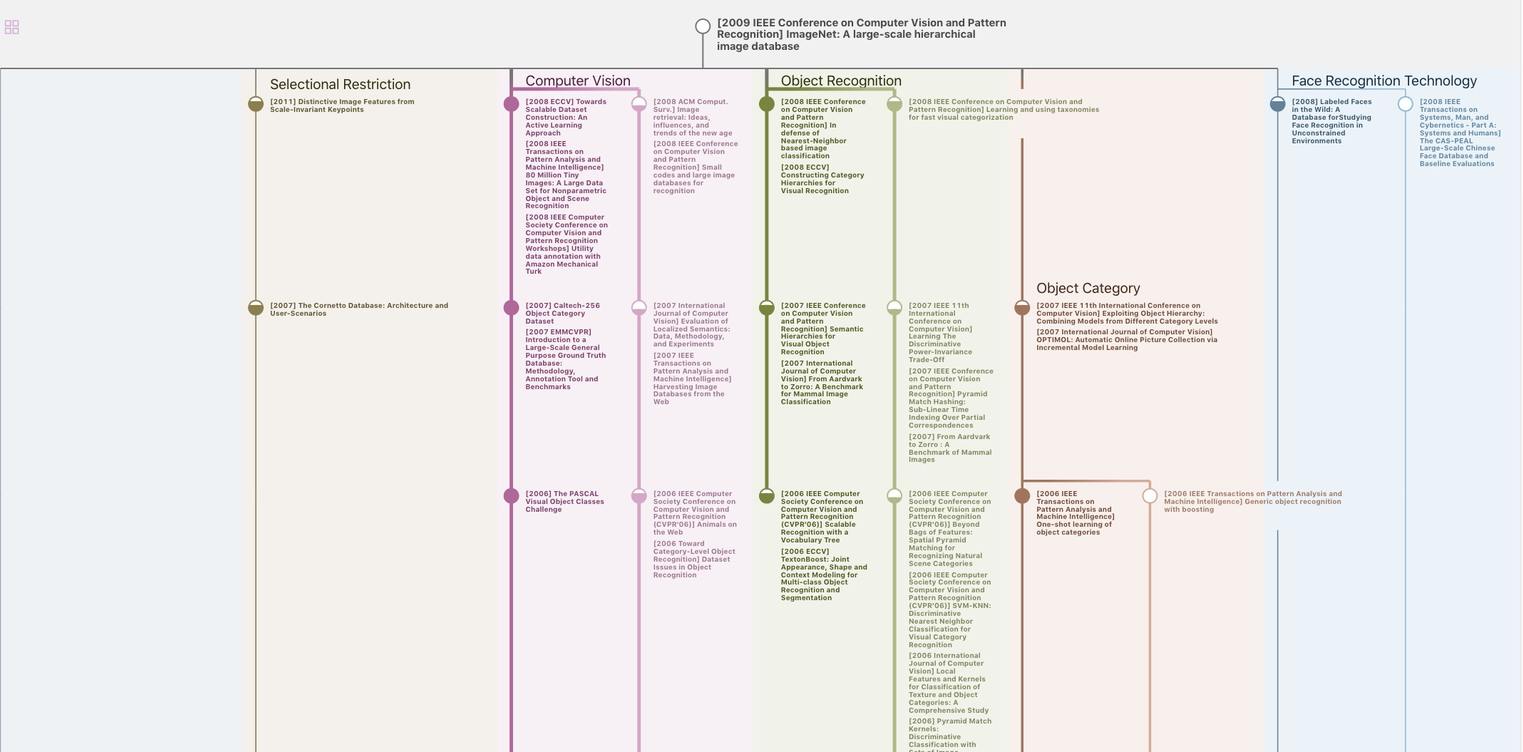
生成溯源树,研究论文发展脉络
Chat Paper
正在生成论文摘要