On the generation of global surrogate models through unconstrained multi-objective optimization
Research Square (Research Square)(2022)
摘要
Abstract Deep learning algorithms or ensemble machine learning models often increase accuracy in industry artificial intelligence applications. However, their complexity makes them difficult to understand and show their underlying logic and decisions. In safety-critical applications or for audit purposes, this opaqueness is undesirable. To this end, explainable artificial intelligence techniques attempt to show how these complex models work. One of these techniques is the global surrogate generation method, where simpler, intrinsically interpretable models are trained to provide predictions as close as possible to their complex counterparts. However, these methods often only consider a single error measure to generate these simpler models. In order to solve this problem, we propose a multi-objective optimization problem to generate surrogates optimizing multiple error metrics simultaneously. Results show that this proposal can generate surrogates for classification and regression problems, often outperforming the current state-of-the-art method.
更多查看译文
关键词
global surrogate models,optimization,multi-objective
AI 理解论文
溯源树
样例
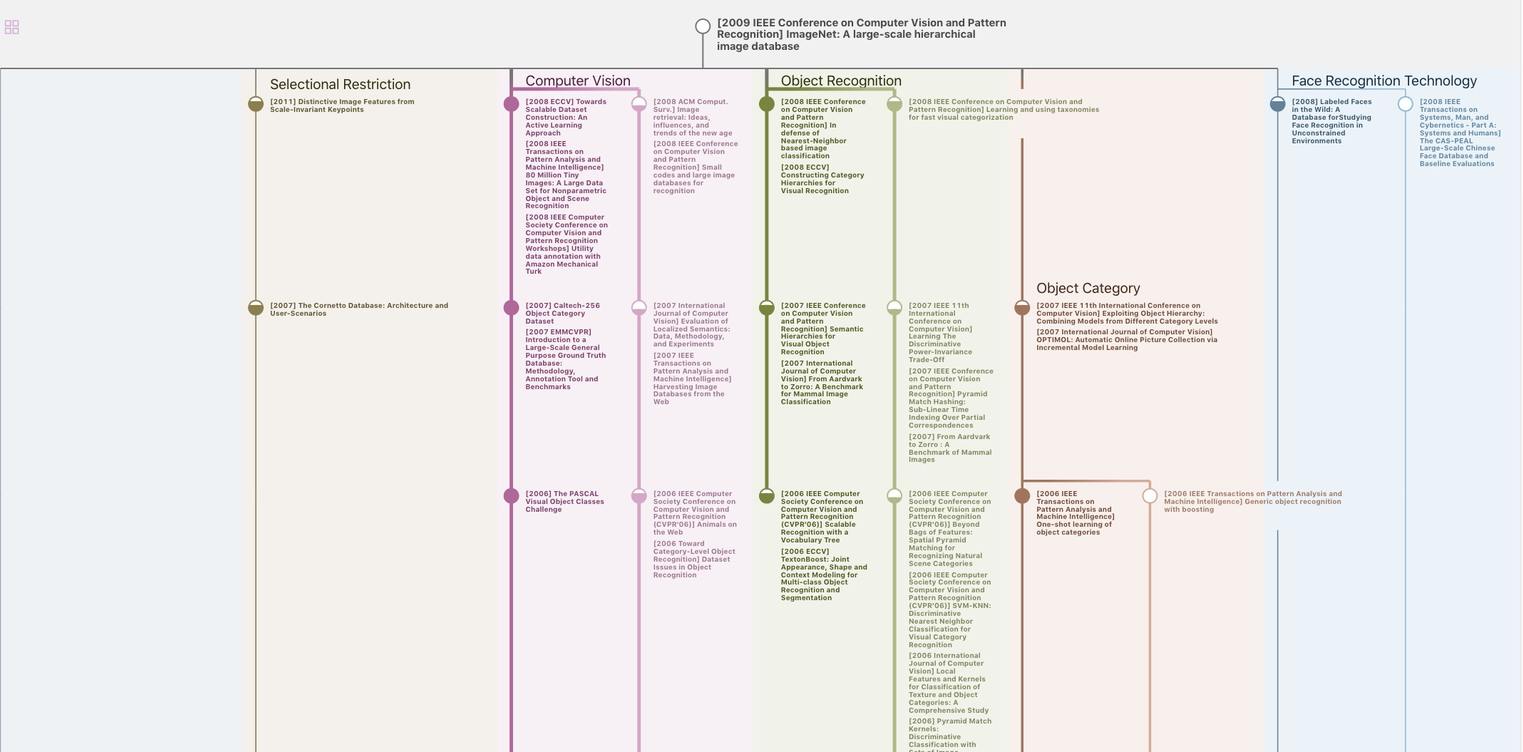
生成溯源树,研究论文发展脉络
Chat Paper
正在生成论文摘要