Multi-domain Feature Fusion Neural Network for Electrocardiogram Classification
crossref(2022)
摘要
Abstract Electrocardiogram (ECG) is of great significant in detecting cardiovascular disease. With the continuous development of computerized ECG interpretation technology, artificial intelligence method shows potential in realizing high accuracy of arrhythmia auto classification. Recently, many research studies information mining of ECG signals from time domain to time-frequency domain. However, current models of automatic arrhythmia detection extract the features in time-domain and time-frequency domain separately, and merge the features of multi-domain in the last layer. The information of middle layers of multi-domain is not used fully. In this study, we develop an ECG classifier based on Multiple Domain Features Fusion Network with Lead Attention (MDFF-LA), which can realize feature fusion in multi-domain. MDFF-LA can extract features in time domain and frequency domain features respectively. In the process of feature extraction in multi-domain, the feature weight of one domain is optimized and adjusted through the attention module generated by the other domain. In the proposed model, feature fusion is operated at multi stages to fully integrate information of multi domain. Finally, we conduct comprehensive experiments on three multi lead ECG databases to test the performance. The results demonstrate that the fusion features in multi domain extracted by MDFF-LA can obtain more valuable information, which can provide supported diagnosis for clinicians in practical.
更多查看译文
AI 理解论文
溯源树
样例
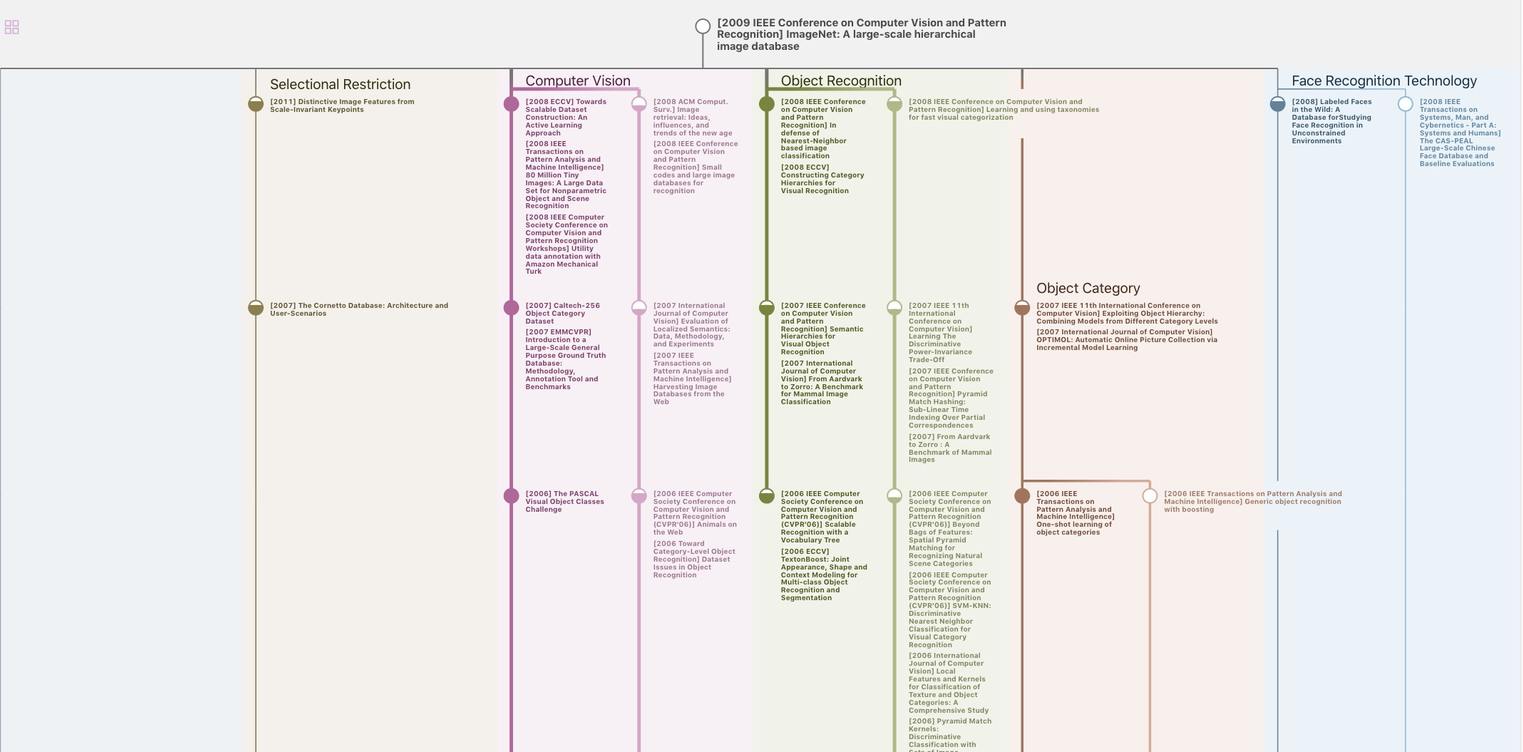
生成溯源树,研究论文发展脉络
Chat Paper
正在生成论文摘要